A POV-based Highway Vehicle Trajectory Dataset and Prediction Architecture
IEEE TRANSACTIONS ON INTELLIGENT TRANSPORTATION SYSTEMS(2024)
摘要
Vehicle Trajectory datasets that provide multiple point-of-views (POVs) can be valuable for various traffic safety and management applications. Despite the abundance of trajectory datasets, few offer a comprehensive and diverse range of driving scenes, capturing multiple viewpoints of various highway layouts, merging lanes, and configurations. This limits their ability to capture the nuanced interactions between drivers, vehicles, and the roadway infrastructure. We introduce the Carolinas Highway Dataset (CHD) (CHD available at: https://github.com/TeCSAR-UNCC/Carolinas_Dataset), a vehicle trajectory, detection, and tracking dataset. CHD is a collection of 1.6 million frames captured in highway-based videos from eye-level and high-angle POVs at eight locations across Carolinas with 338,000 vehicle trajectories. The locations, timing of recordings, and camera angles were carefully selected to capture various road geometries, traffic patterns, lighting conditions, and driving behaviors. We also present PishguVe (PishguVe code available at: https://github.com/TeCSAR-UNCC/PishguVe), a novel vehicle trajectory prediction architecture that uses attention-based graph isomorphism and convolutional neural networks. The results demonstrate that PishguVe outperforms existing algorithms with better ADE and FDE in eye-level, and high-angle POV trajectory datasets. Compared to best-performing models on CHD, PishguVe achieves lower ADE and FDE on eye-level data by 14.58% and 27.38%, respectively, and improves ADE and FDE on high-angle data by 8.3% and 6.9%, respectively.
更多查看译文
关键词
Trajectory,Road transportation,Annotations,Videos,Safety,Cameras,Predictive models,Dataset,vehicle trajectory prediction,real-time,surveillance,monitoring,highway-safety,graph isomorphism network,naturalistic driving behavior
AI 理解论文
溯源树
样例
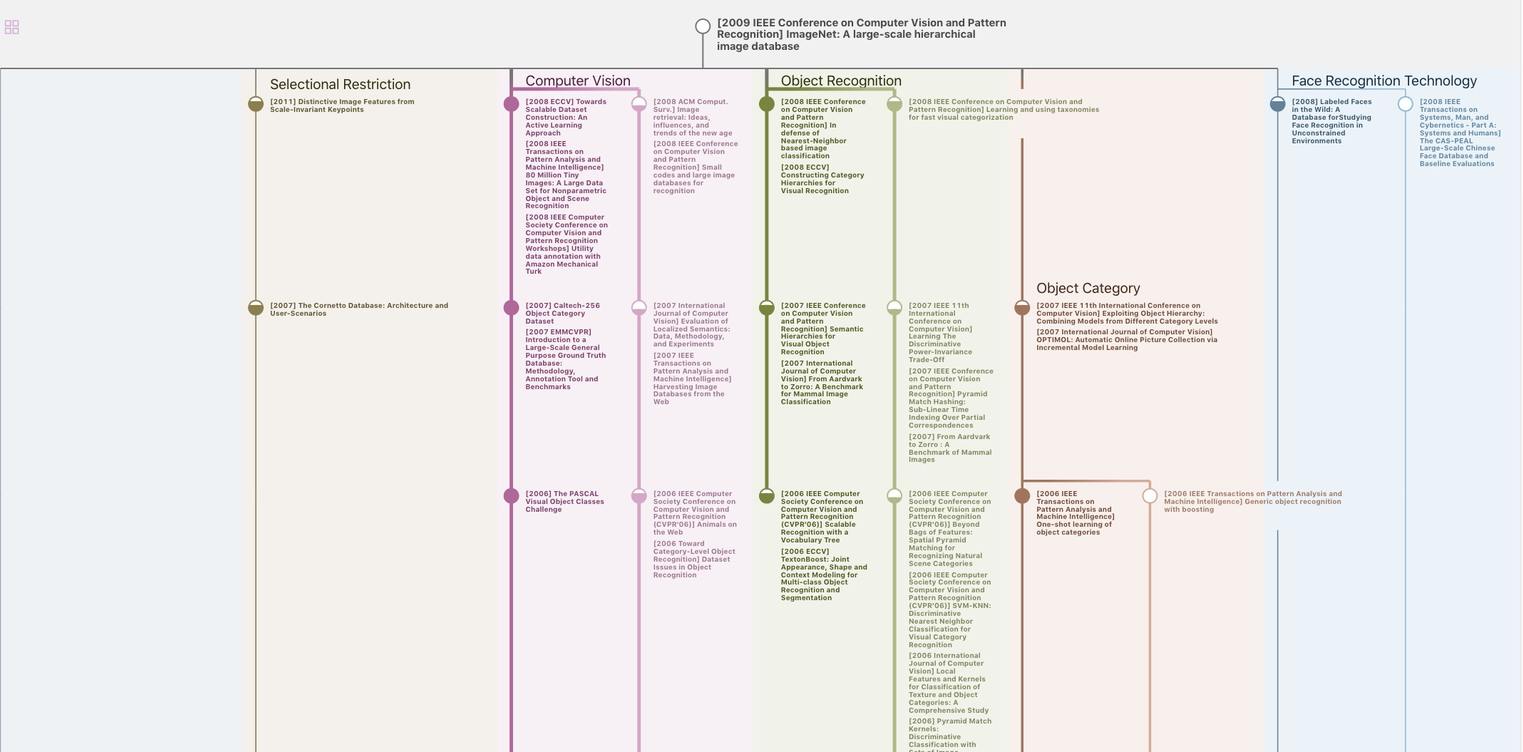
生成溯源树,研究论文发展脉络
Chat Paper
正在生成论文摘要