Deep Hypothesis Tests Detect Clinically Relevant Subgroup Shifts in Medical Images
arXiv (Cornell University)(2023)
Key words
clinically relevant subgroup shifts,deep hypothesis tests,medical
AI Read Science
Must-Reading Tree
Example
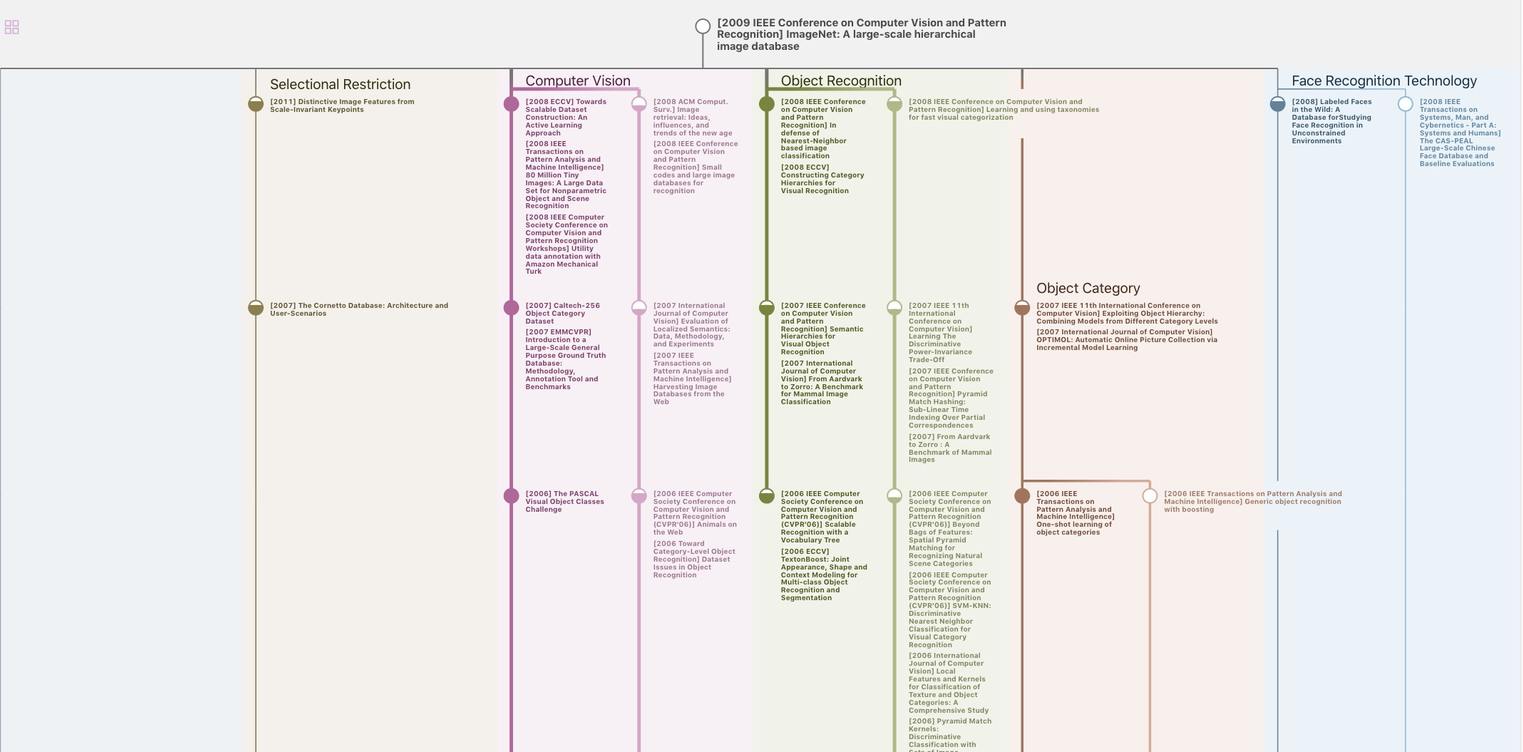
Generate MRT to find the research sequence of this paper
Chat Paper
Summary is being generated by the instructions you defined