Deep Learning-Based Channel Extrapolation for Pattern Reconfigurable Massive MIMO
IEEE TRANSACTIONS ON VEHICULAR TECHNOLOGY(2024)
摘要
Reconfigurable antennas that can dynamically change their operation state exhibit excellent adaptivity and flexibility over traditional antennas, and MIMO arrays that consist of multifunctional and reconfigurable antennas (MRAs) are foreseen as one promising solution towards future Holographic MIMO. Specifically, in pattern reconfigurable MIMO (PR-MIMO) communication systems, accurate acquisition of channel state information (CSI) of all the radiation modes is a challenging task, because using conventional pilot-based channel estimation techniques in PR-MIMO systems incurs overwhelming pilot overheads. In this letter, we leverage deep learning methods to design a PR neural network, which can use the estimated CSI for one radiation mode to infer CSIs for the other radiation modes. In order to reduce the pilot overheads, we propose a new channel estimation method specially for PR-MIMO systems, which divides the transmit antennas of PR-MIMO into groups and antennas in different groups employ different radiation modes. Compared with conventional full-connected real-valued deep neural networks (DNN), the PR neural network which uses complex-valued coefficients can work directly in the complex domain. Experiment results show that the proposed channel extrapolation method offers significant performance gains in terms of extrapolation accuracy over benchmark schemes.
更多查看译文
关键词
Deep learning,Massive MIMO,pattern reconfigurable antenna,channel extrapolation,neural networks
AI 理解论文
溯源树
样例
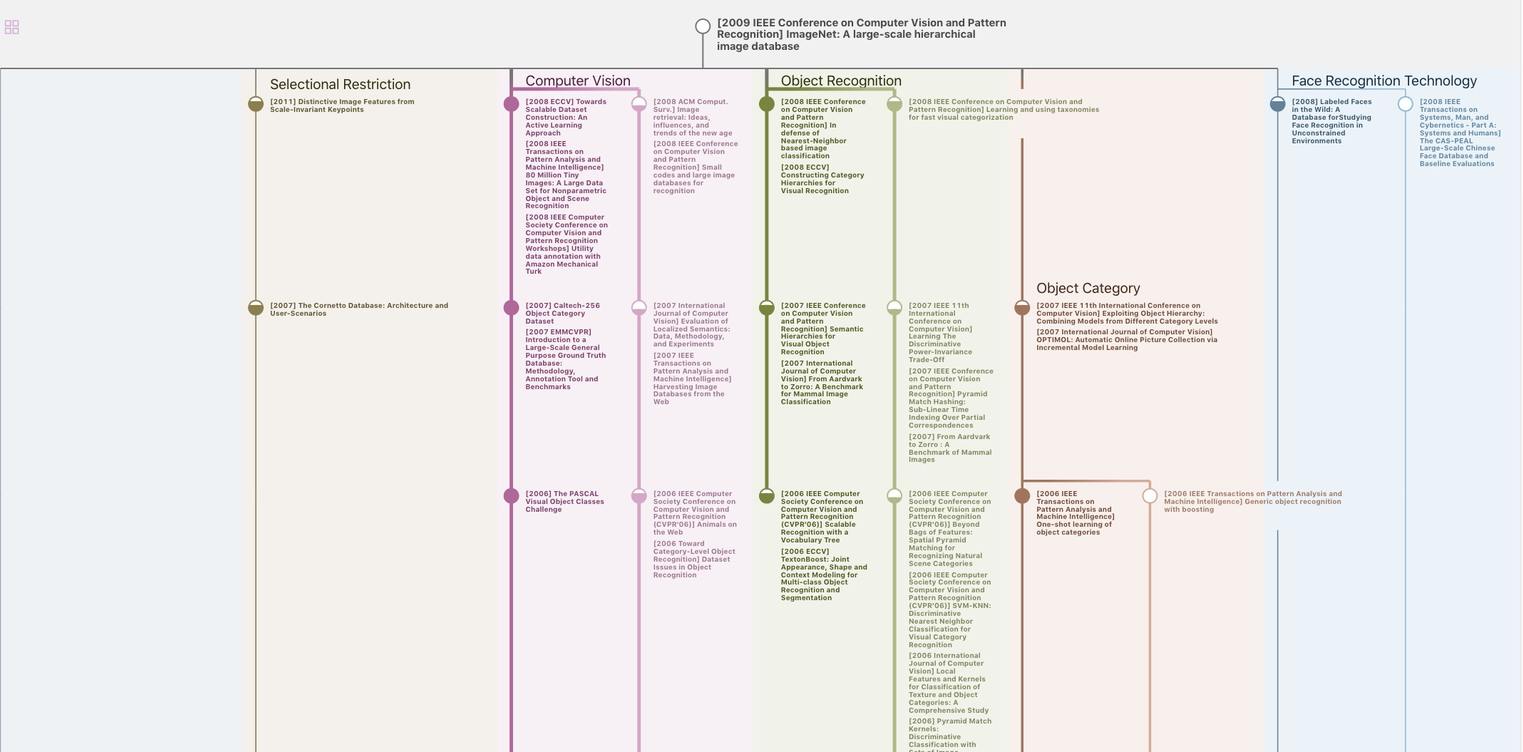
生成溯源树,研究论文发展脉络
Chat Paper
正在生成论文摘要