Learning Efficient Coding of Natural Images with Maximum Manifold Capacity Representations
NeurIPS(2023)
摘要
Self-supervised Learning (SSL) provides a strategy for constructing useful representations of images without relying on hand-assigned labels. Many such methods aim to map distinct views of the same scene or object to nearby points in the representation space, while employing some constraint to prevent representational collapse. Here we recast the problem in terms of efficient coding by adopting manifold capacity, a measure that quantifies the quality of a representation based on the number of linearly separable object manifolds it can support, as the efficiency metric to optimize. Specifically, we adapt the manifold capacity for use as an objective function in a contrastive learning framework, yielding a Maximum Manifold Capacity Representation (MMCR). We apply this method to unlabeled images, each augmented by a set of basic transformations, and find that it learns meaningful features using the standard linear evaluation protocol. Specifically, we find that MMCRs support performance on object recognition comparable to or surpassing that of recently developed SSL frameworks, while providing more robustness to adversarial attacks. Empirical analyses reveal differences between MMCRs and representations learned by other SSL frameworks, and suggest a mechanism by which manifold compression gives rise to class separability.
更多查看译文
关键词
natural images,efficient coding,capacity representations
AI 理解论文
溯源树
样例
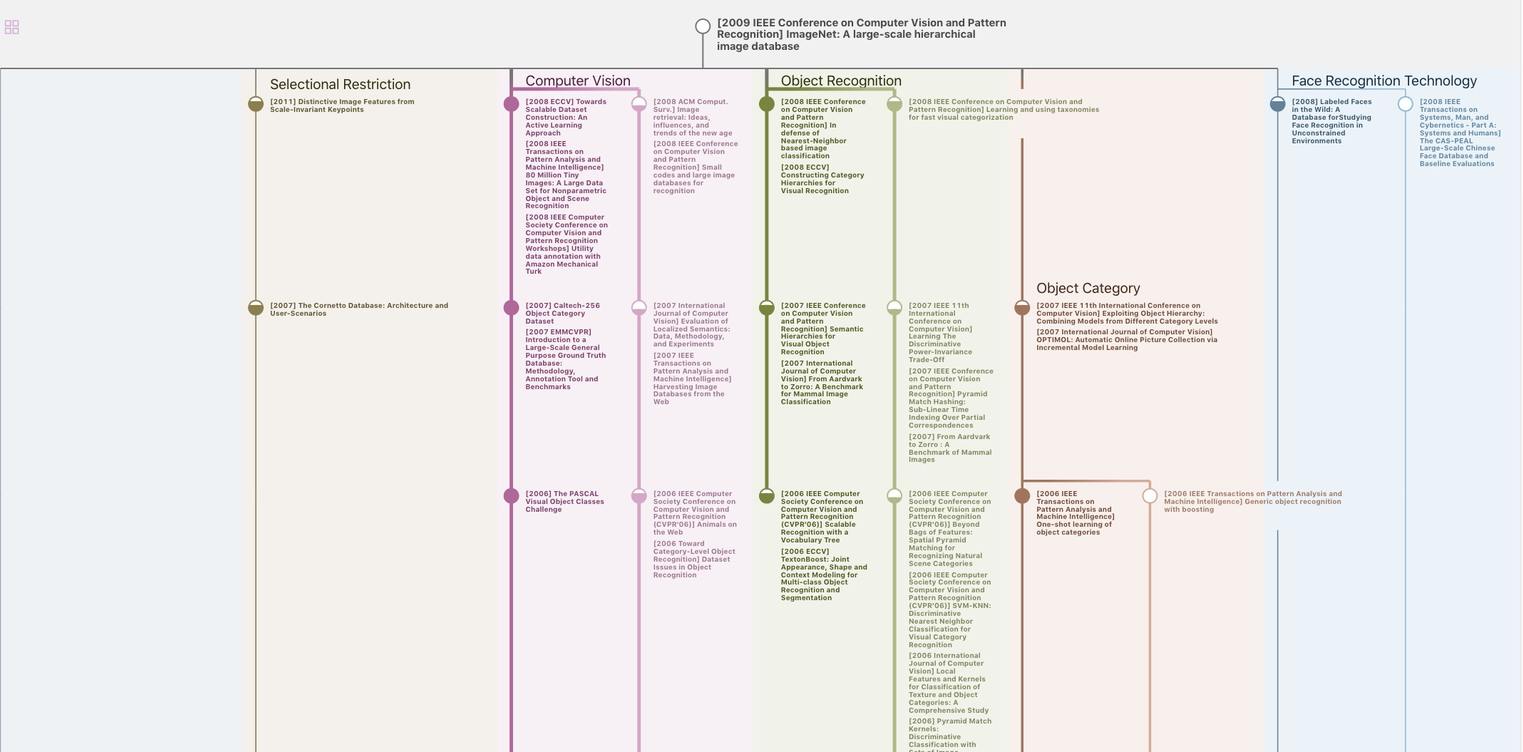
生成溯源树,研究论文发展脉络
Chat Paper
正在生成论文摘要