T-Cell Receptor Optimization with Reinforcement Learning and Mutation Polices for Precision Immunotherapy
arxiv(2023)
摘要
T cells monitor the health status of cells by identifying foreign peptides displayed on their surface. T-cell receptors (TCRs), which are protein complexes found on the surface of T cells, are able to bind to these peptides. This process is known as TCR recognition and constitutes a key step for immune response. Optimizing TCR sequences for TCR recognition represents a fundamental step towards the development of personalized treatments to trigger immune responses killing cancerous or virus-infected cells. In this paper, we formulated the search for these optimized TCRs as a reinforcement learning (
$$\mathop {\texttt{RL}}\limits $$
) problem, and presented a framework
$$\mathop {\texttt{TCRPPO}}\limits $$
with a mutation policy using proximal policy optimization.
$$\mathop {\texttt{TCRPPO}}\limits $$
mutates TCRs into effective ones that can recognize given peptides.
$$\mathop {\texttt{TCRPPO}}\limits $$
leverages a reward function that combines the likelihoods of mutated sequences being valid TCRs measured by a new scoring function based on deep autoencoders, with the probabilities of mutated sequences recognizing peptides from a peptide-TCR interaction predictor. We compared
$$\mathop {\texttt{TCRPPO}}\limits $$
with multiple baseline methods and demonstrated that
$$\mathop {\texttt{TCRPPO}}\limits $$
significantly outperforms all the baseline methods to generate positive binding and valid TCRs. These results demonstrate the potential of
$$\mathop {\texttt{TCRPPO}}\limits $$
for both precision immunotherapy and peptide-recognizing TCR motif discovery.
更多查看译文
关键词
reinforcement learning,mutation polices,receptor,t-cell
AI 理解论文
溯源树
样例
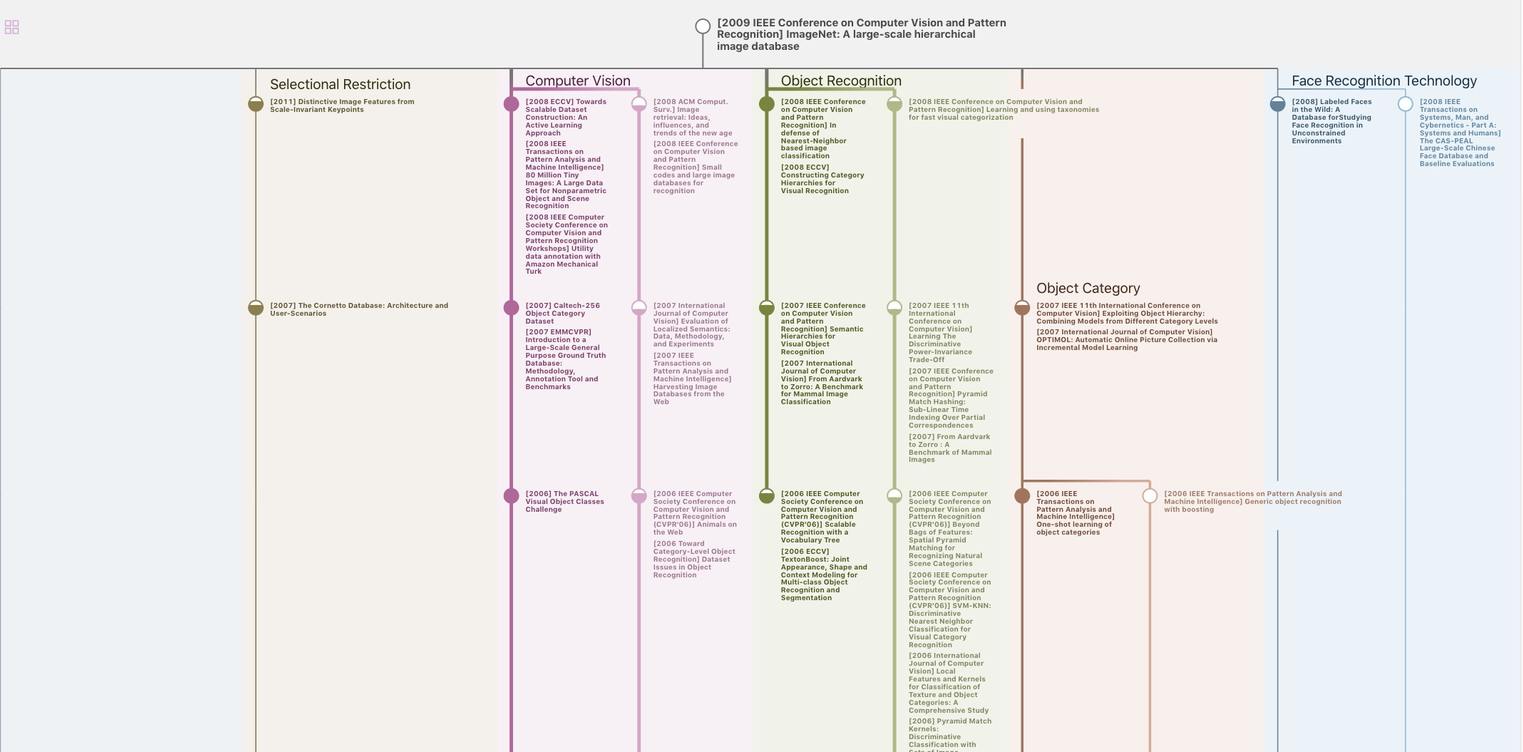
生成溯源树,研究论文发展脉络
Chat Paper
正在生成论文摘要