Dimensionality Reduction Method Based on Multiple Feature-Space Collaborative Discriminative Projection for Rotor Fault Diagnosis
Measurement science & technology(2023)
摘要
At present, the trend for complex and intelligent rotating machinery and equipment, which generate a large amount of high-dimensional and nonlinear fault monitoring data that is difficult to handle, is becoming more and more apparent. This makes the traditional dimensionality reduction algorithms based on point-to-point metrics or a small number of graph-embedding structures lose their utility. To solve this problem, a multiple feature-space collaborative discriminative projection (MFSCDP) algorithm for rotor fault data set dimensionality reduction is proposed. The algorithm first improves the projection metric from sample point to feature space into the median metric in order to achieve the effect of weakening the extrapolation error of the algorithm, and based on this, we propose a sample point-to-point guided nearest-neighbor feature space selection method to improve the construction efficiency of the feature space embedding graph. Then, we use Relief F to indirectly construct the reduced dimensional projection matrix with multiple feature space of collaboration. Finally, the proposed MFSCDP algorithm is used for the dimensionality reduction process of a rotor fault data set. The algorithm's performance was verified using experimental information from rotor failure simulations of two different structural types. The results show that the algorithm can reduce the difficulty of fault classification and improve the accuracy of identification.
更多查看译文
关键词
fault diagnosis,dimension reduction,feature space,multi-graph collaboration
AI 理解论文
溯源树
样例
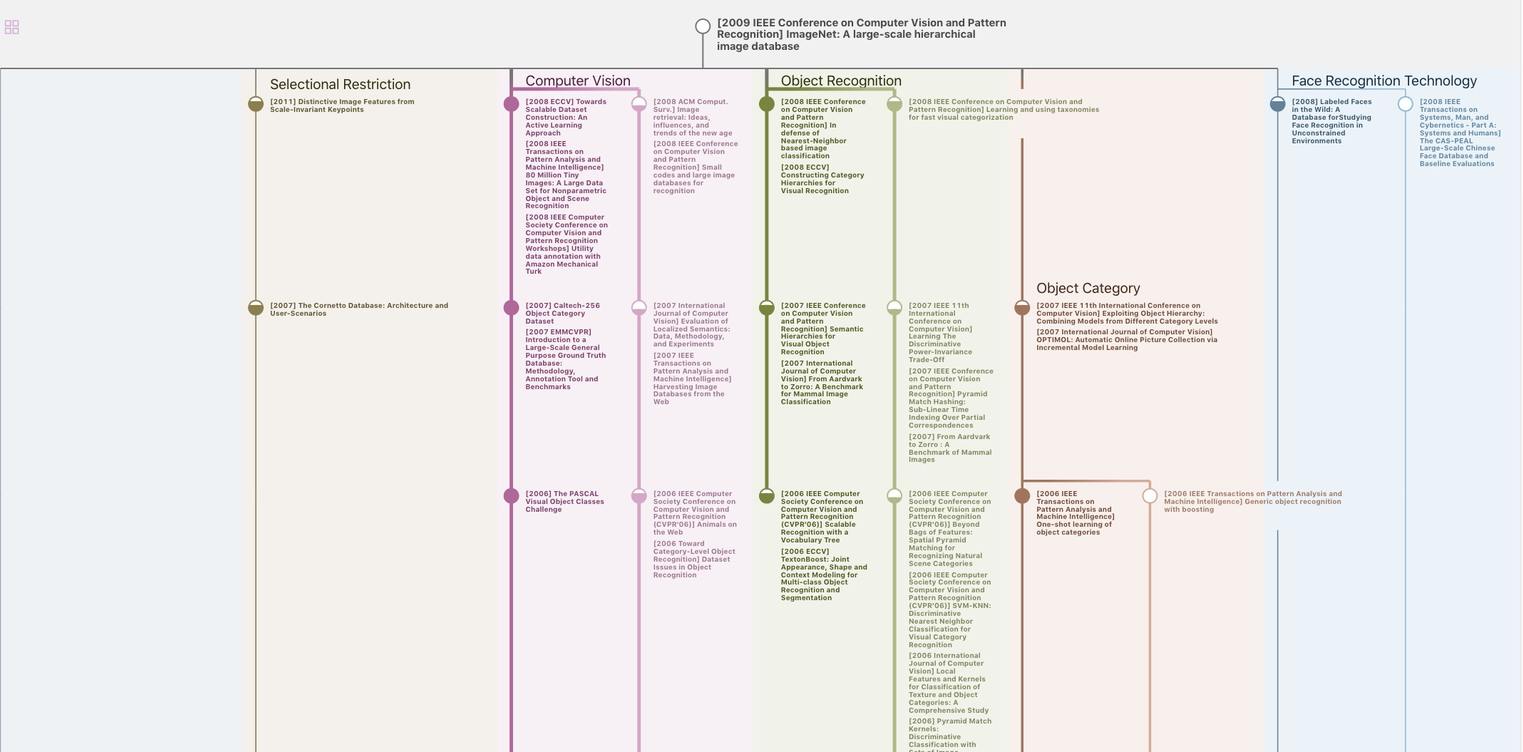
生成溯源树,研究论文发展脉络
Chat Paper
正在生成论文摘要