YOLO POD: a Fast and Accurate Multi-Task Model for Dense Soybean Pod Counting
Plant methods(2023)
摘要
Background The number of soybean pods is one of the most important indicators of soybean yield, pod counting is crucial for yield estimation, cultivation management, and variety breeding. Counting pods manually is slow and laborious. For crop counting, using object detection network is a common practice, but the scattered and overlapped pods make the detection and counting of the pods difficult. Results We propose an approach that we named YOLO POD, based on the YOLO X framework. On top of YOLO X, we added a block for predicting the number of pods, modified the loss function, thus constructing a multi-task model, and introduced the Convolutional Block Attention Module (CBAM). We achieve accurate identification and counting of pods without reducing the speed of inference. The results showed that the R 2 between the number predicted by YOLO POD and the ground truth reached 0.967, which is improved by 0.049 compared to YOLO X, while the inference time only increased by 0.08 s. Moreover, MAE, MAPE, RMSE are only 4.18, 10.0%, 6.48 respectively, the deviation is very small. Conclusions We have achieved the first accurate counting of soybean pods and proposed a new solution for the detection and counting of dense objects.
更多查看译文
关键词
Soybean,Deep learning,Objection detection,Multi-Task learning,Yield estimation
AI 理解论文
溯源树
样例
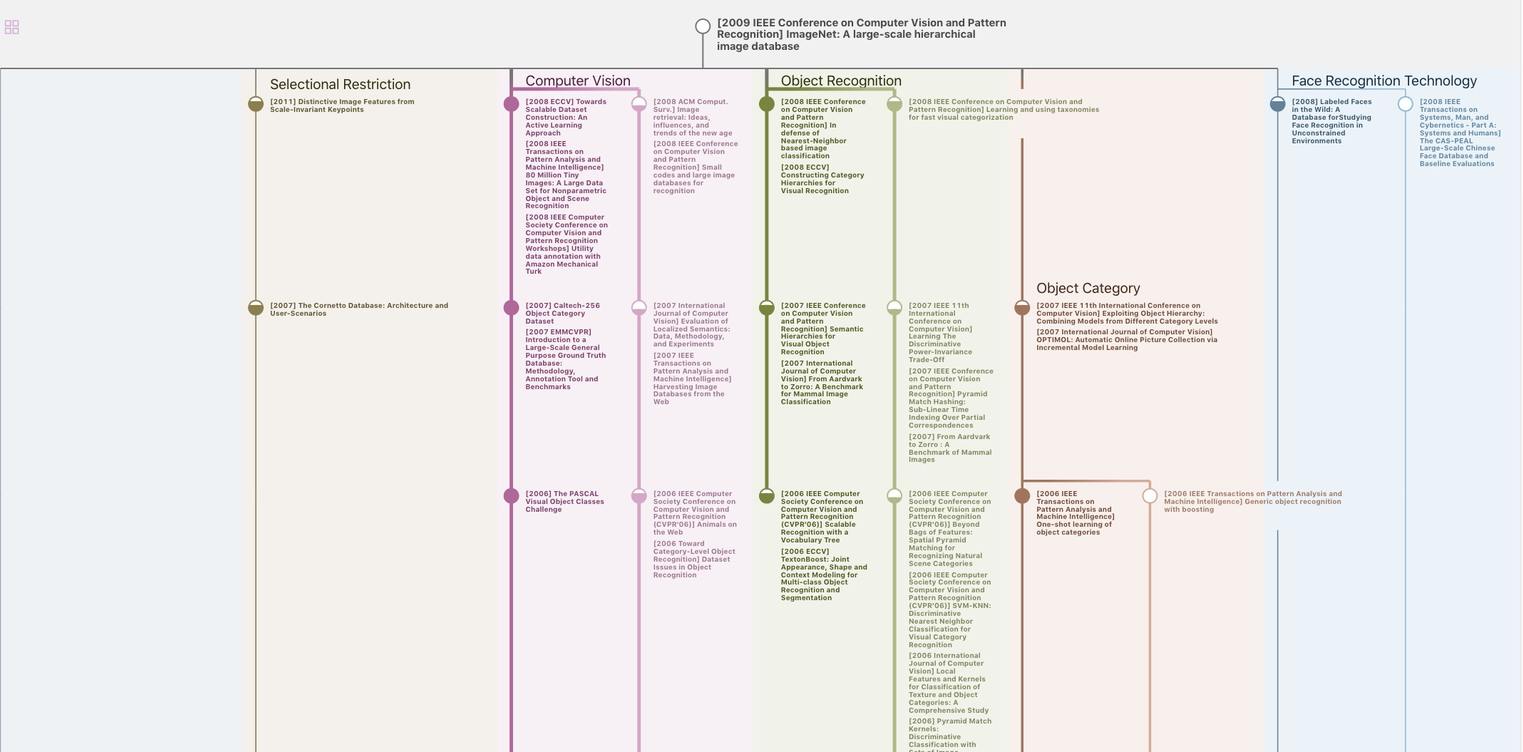
生成溯源树,研究论文发展脉络
Chat Paper
正在生成论文摘要