Mitigating Modality Discrepancies for RGB-T Semantic Segmentation.
IEEE TRANSACTIONS ON NEURAL NETWORKS AND LEARNING SYSTEMS(2024)
摘要
Semantic segmentation models gain robustness against adverse illumination conditions by taking advantage of complementary information from visible and thermal infrared (RGB-T) images. Despite its importance, most existing RGB-T semantic segmentation models directly adopt primitive fusion strategies, such as elementwise summation, to integrate multimodal features. Such strategies, unfortunately, overlook the modality discrepancies caused by inconsistent unimodal features obtained by two independent feature extractors, thus hindering the exploitation of cross-modal complementary information within the multimodal data. For that, we propose a novel network for RGB-T semantic segmentation, i.e. MDRNet+, which is an improved version of our previous work ABMDRNet. The core of MDRNet+ is a brand new idea, termed the strategy of bridging-then-fusing, which mitigates modality discrepancies before cross-modal feature fusion. Concretely, an improved Modality Discrepancy Reduction (MDR+) subnetwork is designed, which first extracts unimodal features and reduces their modality discrepancies. Afterward, discriminative multimodal features for RGB-T semantic segmentation are adaptively selected and integrated via several channel-weighted fusion (CWF) modules. Furthermore, a multiscale spatial context (MSC) module and a multiscale channel context (MCC) module are presented to effectively capture the contextual information. Finally, we elaborately assemble a challenging RGB-T semantic segmentation dataset, i.e., RTSS, for urban scene understanding to mitigate the lack of well-annotated training data. Comprehensive experiments demonstrate that our proposed model surpasses other state-of-the-art models on the MFNet, PST900, and RTSS datasets remarkably.
更多查看译文
关键词
Bridging-then-fusing,contextual information,dataset,modality discrepancy reduction,RGB-T semantic segmentation
AI 理解论文
溯源树
样例
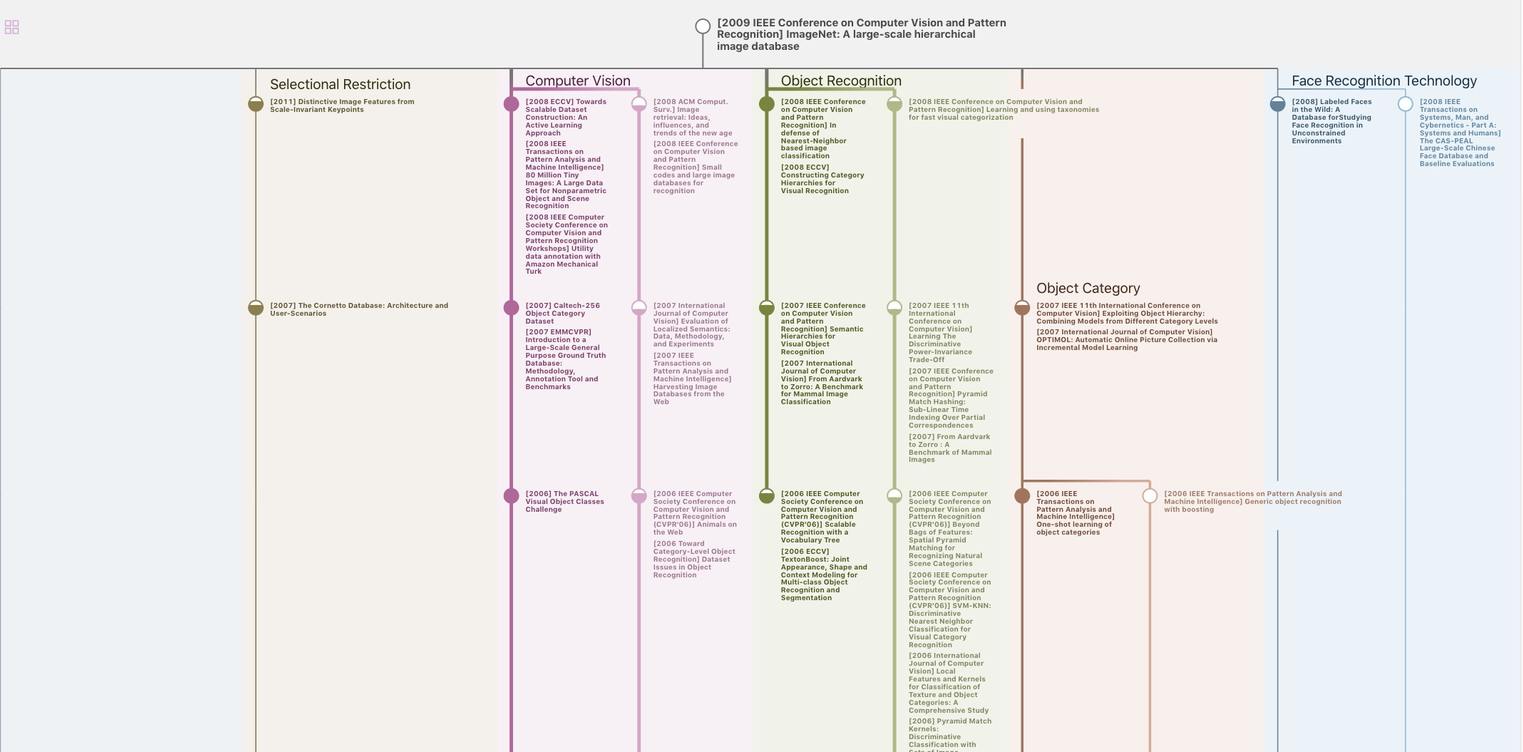
生成溯源树,研究论文发展脉络
Chat Paper
正在生成论文摘要