The Explainable Potential of Coupling Metaheuristics-Optimized-XGBoost and SHAP in Revealing VOCs’ Environmental Fate
Atmosphere(2023)
摘要
In this paper, we explore the computational capabilities of advanced modeling tools to reveal the factors that shape the observed benzene levels and behavior under different environmental conditions. The research was based on two-year hourly data concentrations of inorganic gaseous pollutants, particulate matter, benzene, toluene, m, p-xylenes, total nonmethane hydrocarbons, and meteorological parameters obtained from the Global Data Assimilation System. In order to determine the model that will be capable of achieving a superior level of performance, eight metaheuristics algorithms were tested for eXtreme Gradient Boosting optimization, while the relative SHapley Additive exPlanations values were used to estimate the relative importance of each pollutant level and meteorological parameter for the prediction of benzene concentrations. According to the results, benzene levels are mostly shaped by toluene and the finest aerosol fraction concentrations, in the environment governed by temperature, volumetric soil moisture content, and momentum flux direction, as well as by levels of total nonmethane hydrocarbons and total nitrogen oxide. The types of conditions which provided the environment for the impact of toluene, the finest aerosol, and temperature on benzene dynamics are distinguished and described.
更多查看译文
关键词
machine learning,extreme gradient boosting,metaheuristics,swarm intelligence,explainable artificial intelligence,volatile organic compounds,BTEX,benzene
AI 理解论文
溯源树
样例
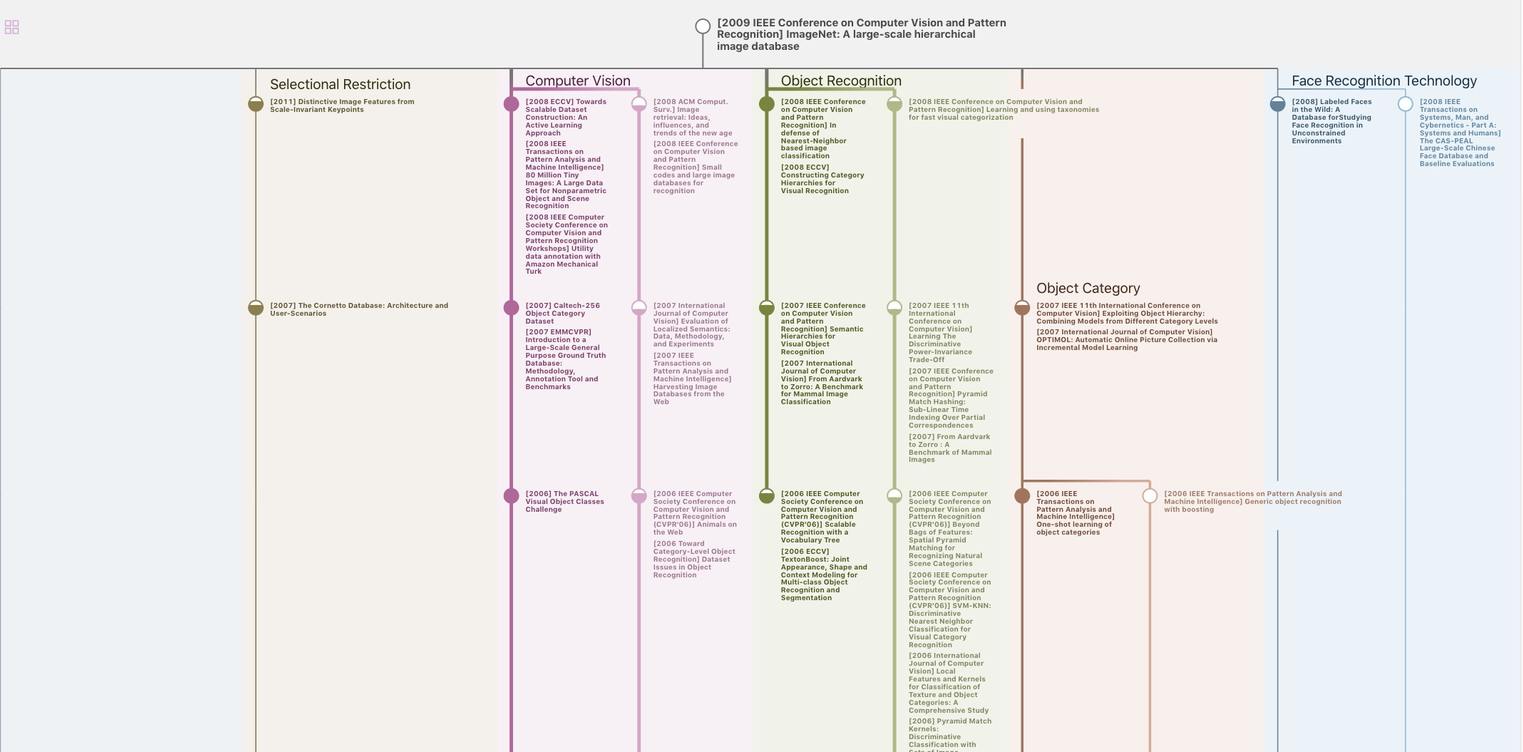
生成溯源树,研究论文发展脉络
Chat Paper
正在生成论文摘要