HyperDNE: Enhanced Hypergraph Neural Network for Dynamic Network Embedding
Neurocomputing(2023)
摘要
Representation learning provides an attractive opportunity to model the evolution of dynamic networks. However, the existing methods have two limitations: (1) most graph neural network-based methods fail to utilize the high-order proximity of nodes that captures the important properties of a network topology; (2) evolutionary dynamics-based methods are much fine-grained in modeling time information but neglect the coherence of dynamic networks, which leads to the model being susceptible to subtle noise. In this paper, we propose an enhanced hypergraph neural network framework for dynamic network embedding (HyperDNE) to tackle these issues. Specifically, we innovatively design a sequential hypergraph with dual-stream output to explore the group properties of nodes and edges, and a line graph neural network is added as an auxiliary enhancement scheme to further aggregate social influence from the degree of social convergence. Then, we compute the final embedding through attentions along the node and hyperedge levels to fuse multi-level variations in the network structure. The experimental results on six real networks demonstrate significant gains for HyperDNE over several state-of-the-art network embedding baselines. The dataset and source code of HyperDNE are publicly available at https://github.com/qhgz2013/HyperDNE.
更多查看译文
关键词
Dynamic network,Graph neural network,Attention,Representation learning
AI 理解论文
溯源树
样例
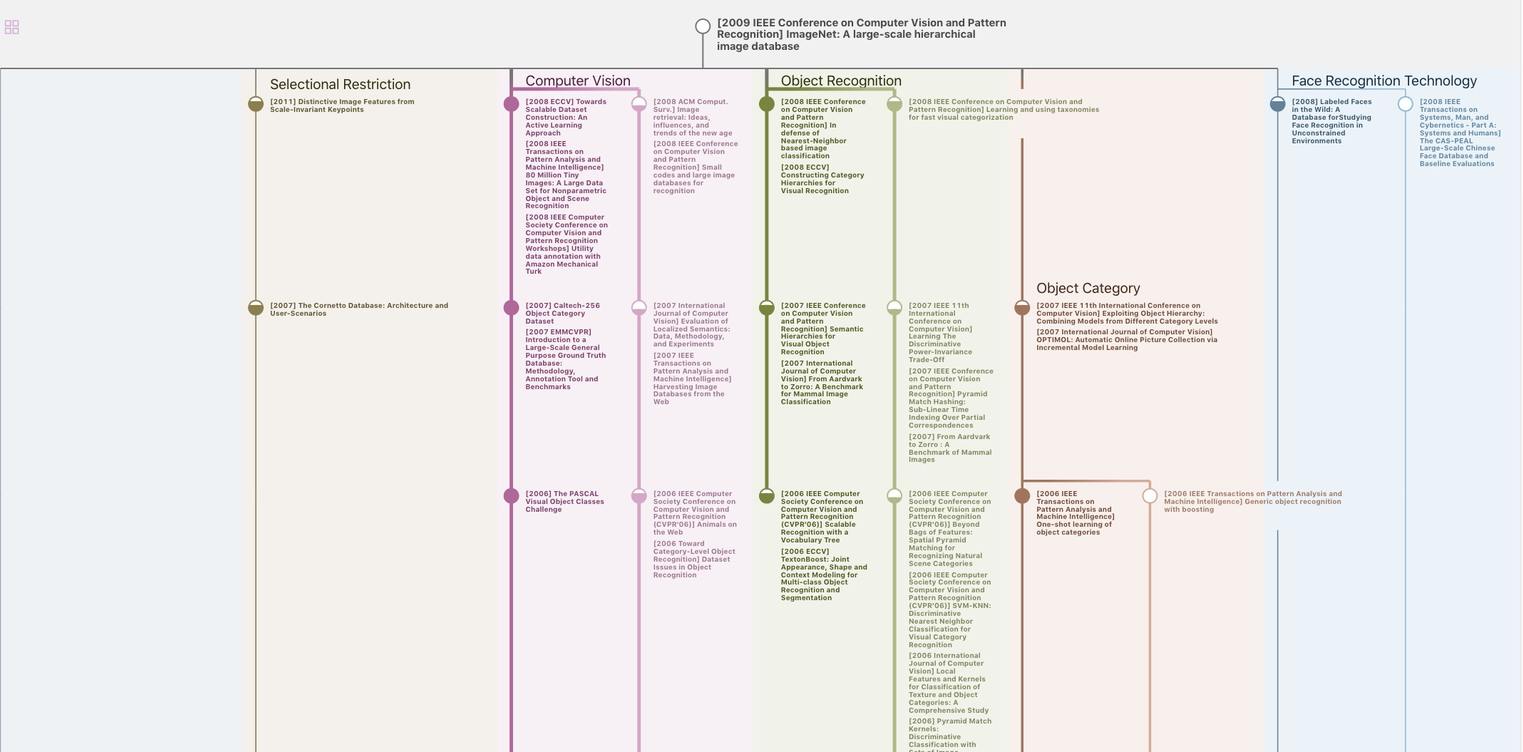
生成溯源树,研究论文发展脉络
Chat Paper
正在生成论文摘要