Joint Learning of Blind Super-Resolution and Crack Segmentation for Realistic Degraded Images
IEEE transactions on instrumentation and measurement(2024)
摘要
This article proposes crack segmentation augmented by super-resolution (SR) with deep neural networks. In the proposed method, an SR network is jointly trained with a binary segmentation network in an end-to-end manner. This joint learning allows the SR network to be optimized for improving segmentation results. For realistic scenarios, the SR network is extended from nonblind to blind for processing a low-resolution (LR) image degraded by unknown blurs. The joint network is improved by our proposed two extra paths that further encourage the mutual optimization between SR and segmentation. Comparative experiments with state-of-the-art (SoTA) segmentation methods demonstrate the superiority of our joint learning, and various ablation studies prove the effects of our contributions.
更多查看译文
关键词
Image segmentation,Task analysis,Multitasking,Kernel,Inspection,Superresolution,Training,Blind super-resolution (SR),crack segmentation,image processing,joint learning,multitask learning
AI 理解论文
溯源树
样例
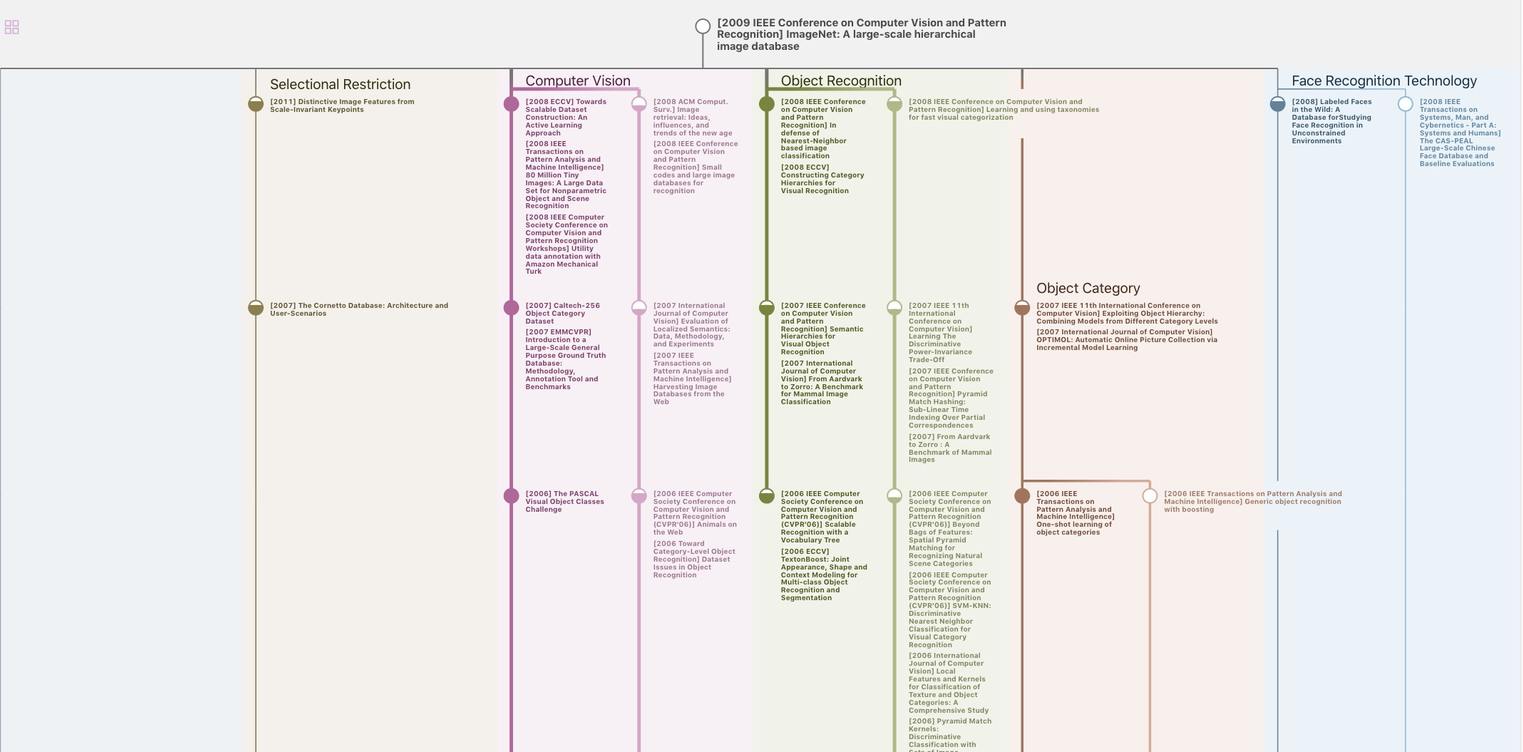
生成溯源树,研究论文发展脉络
Chat Paper
正在生成论文摘要