Impacts of meteorological biases on forecasting surface PM2.5 concentration over the Beijing-Tianjing-Hebei region
crossref(2023)
摘要
<p>Forecast biases in the meteorological fields have long been recognized as the main limitation on accuracy and predictability of air quality forecast. However, the quantitative studies on the impacts of meteorological forecast biases on air quality forecast are insufficient, and its mechanisms in different seasons are still unclear. In this study, series of forecasts from 2-Day (24 ~ 48-hour) to 7-Day (144 ~168-hour) for January, April, July, and October of 2018 are conducted over the Beijing-Tianjing-Hebei (BTH) region and the impacts of meteorological forecast bias on surface PM<sub>2.5</sub> concentration forecast at each leading-time are analyzed. The results show that the forecast biases of surface PM<sub>2.5</sub> concentration caused by meteorological forecast biases keep increasing with the increasing leading-time in all seasons. The impacts of meteorological forecast biases are strongest in spring, with the forecast bias of PM<sub>2.5</sub> concentrations up to 187% in the 7-Day forecast, followed by autumn (123%), summer (112%) and winter (80%). By the contribution analysis of relevant processes and their changes with the increase of leading-time, it is found that the dry deposition, transport and PBL mixing are the main processes contributing to the growth of forecast bias of surface PM<sub>2.5</sub> concentrations. In addition, the correspondence between the contributions of relevant processes and the meteorological factor are examined. It is found that the changes of contributions of processes are closely related to the difference in the forecasted meteorological factors such as surface winds, wind fields at 850hPa, PBL height, shortwave radiation and precipitations at each leading-time. This study highlights the importance of meteorological forecast bias in surface PM<sub>2.5</sub> concentration forecasting, and provides useful information for the improvement of air quality forecast accuracy and supports the policies of air pollution controlling.</p>
更多查看译文
AI 理解论文
溯源树
样例
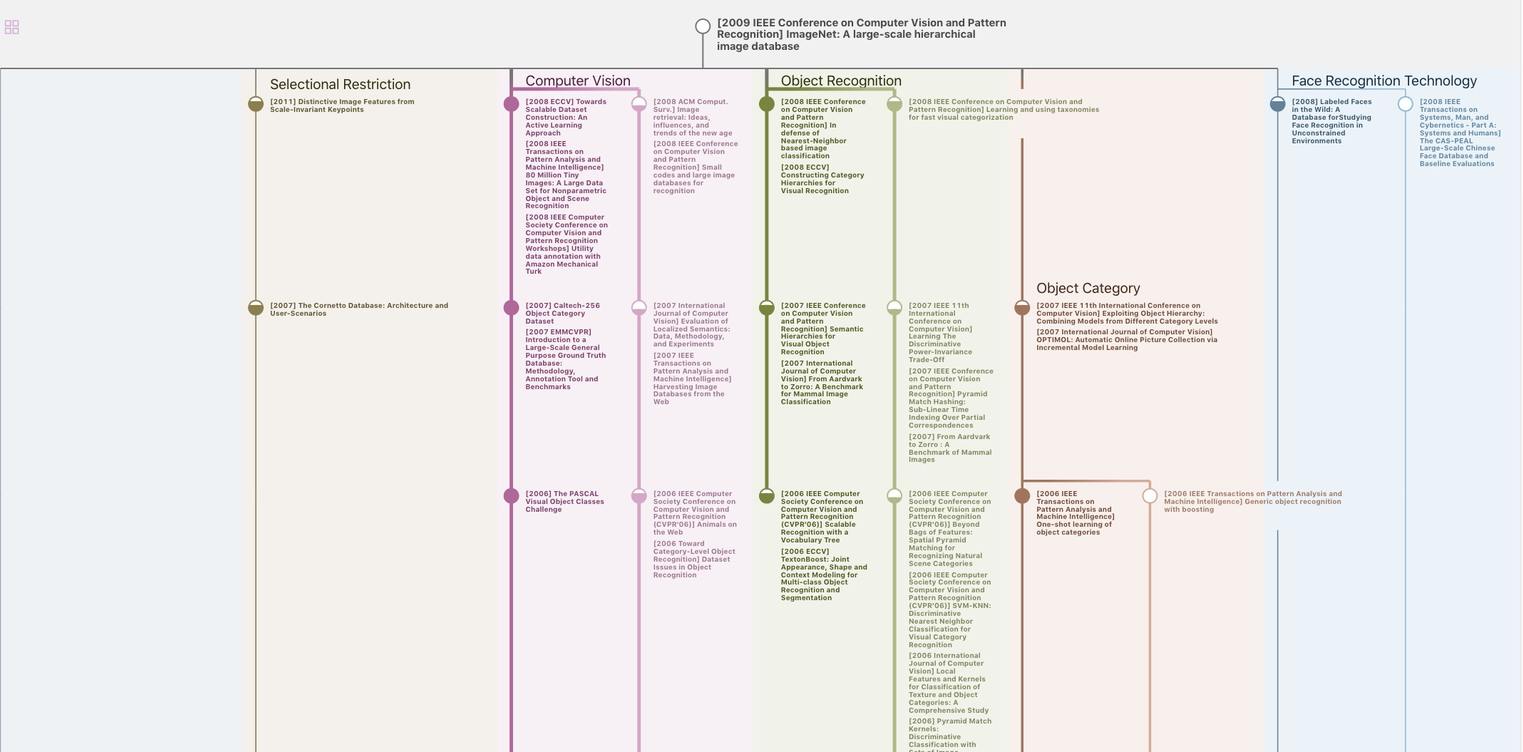
生成溯源树,研究论文发展脉络
Chat Paper
正在生成论文摘要