Active learning for structural reliability analysis with multiple limit state functions through variance-enhanced PC-Kriging surrogate models
CoRR(2023)
摘要
Existing active strategies for training surrogate models yield accurate
structural reliability estimates by aiming at design space regions in the
vicinity of a specified limit state function. In many practical engineering
applications, various damage conditions, e.g. repair, failure, should be
probabilistically characterized, thus demanding the estimation of multiple
performance functions. In this work, we investigate the capability of active
learning approaches for efficiently selecting training samples under a limited
computational budget while still preserving the accuracy associated with
multiple surrogated limit states. Specifically, PC-Kriging-based surrogate
models are actively trained considering a variance correction derived from
leave-one-out cross-validation error information, whereas the sequential
learning scheme relies on U-function-derived metrics. The proposed active
learning approaches are tested in a highly nonlinear structural reliability
setting, whereas in a more practical application, failure and repair events are
stochastically predicted in the aftermath of a ship collision against an
offshore wind substructure. The results show that a balanced computational
budget administration can be effectively achieved by successively targeting the
specified multiple limit state functions within a unified active learning
scheme.
更多查看译文
关键词
structural reliability analysis,surrogate models,variance-enhanced,pc-kriging
AI 理解论文
溯源树
样例
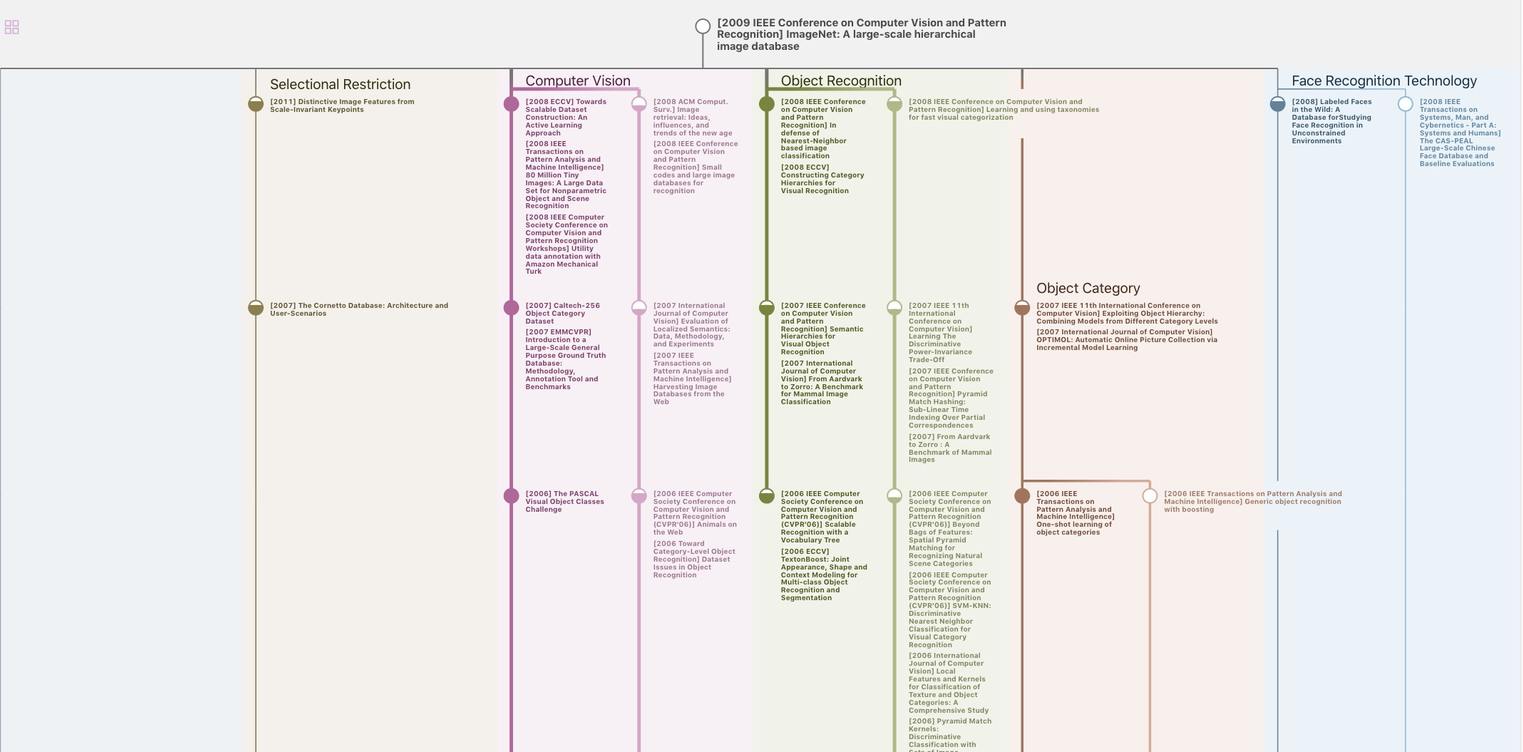
生成溯源树,研究论文发展脉络
Chat Paper
正在生成论文摘要