Back-End and Flexible Substrate Compatible Analog Ferroelectric Field-Effect Transistors for Accurate Online Training in Deep Neural Network Accelerators
ADVANCED INTELLIGENT SYSTEMS(2023)
摘要
Online training of deep neural networks (DNN) can be significantly accelerated by performing in situ vector-matrix multiplication in a crossbar array of analog memories. However, training accuracies often suffer due to nonideal properties of synapses such as nonlinearity, asymmetry, limited bit precision, and dynamic weight update range within a constrained power budget. Herein, a fully scalable process is reported for digital and analog ferroelectric memory transistors with possibilities for both volatile and nonvolatile data retention and <4 V operation that would be suitable as programmable synaptic weight elements. Ferroelectric copolymer P(VDF-TrFE) gate insulator and 2D semiconductor MoS2 as the n-type semiconducting channel material make them suitable for flexible and wearable substrate integration. The ferroelectric-only devices show excellent performance as digital nonvolatile memory operating at <+/- 5 V while the hybrid ferroelectric-dielectric devices show quasi-continuous resistive switching resulting from gradual ferroelectric domain rotation. Analog conductance states of the hybrid devices allow good linearity and symmetry of weight updates and produce a dynamic conductance range of 104 with >16 reproducible conducting states. Network training experiments with these ferroelectric field-effect transistors show >96% classification accuracy with Modified National Institute of Standards and Technology (MNIST) handwritten datasets highlighting their potential for implementation in scaled DNN architectures.
更多查看译文
关键词
analog memory,deep neural network,electronic synapses,ferroelectric field effect transistor,online training
AI 理解论文
溯源树
样例
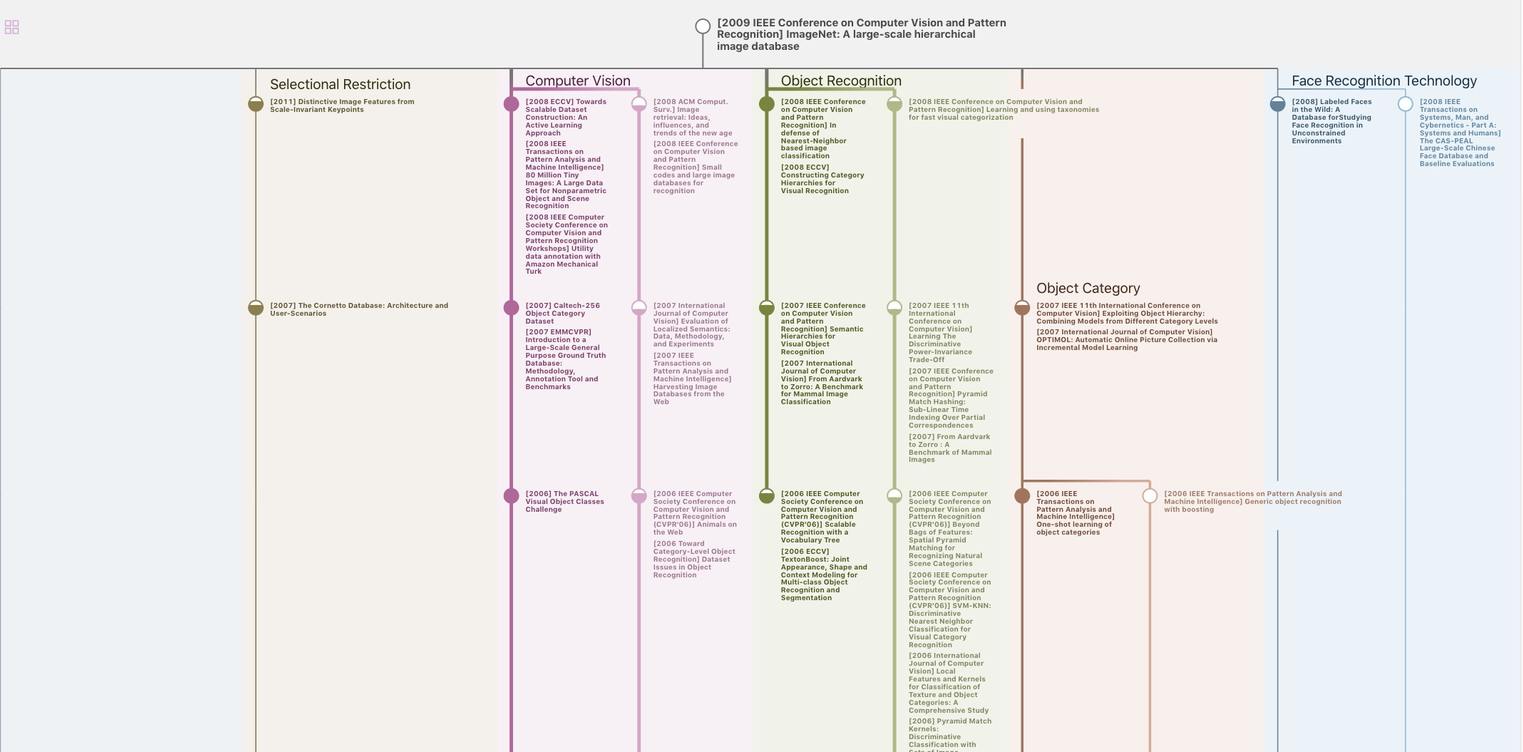
生成溯源树,研究论文发展脉络
Chat Paper
正在生成论文摘要