SuperFormer: Continual Learning Superposition Method for Text Classification
Neural networks(2023)
摘要
One of the biggest challenges in continual learning domains is the tendency of machine learning models to forget previously learned information over time. While overcoming this issue, the existing approaches often exploit large amounts of additional memory and apply model forgetting mitigation mechanisms which substantially prolong the training process. Therefore, we propose a novel SuperFormer method that alleviates model forgetting, while spending negligible additional memory and time. We tackle the continual learning challenges in a learning scenario, where we learn different tasks in a sequential order. We compare our method against several prominent continual learning methods, i.e., EWC, SI, MAS, GEM, PSP, etc. on a set of text classification tasks. We achieve the best average performance in terms of AUROC and AUPRC (0.7% and 0.9% gain on average, respectively) and the lowest training time among all the methods of comparison. On average, our method reduces the total training time by a factor of 5.4-8.5 in comparison to similarly performing methods. In terms of the additional memory, our method is on par with the most memory-efficient approaches.
更多查看译文
关键词
Deep learning,Continual learning,Superposition,Transformers
AI 理解论文
溯源树
样例
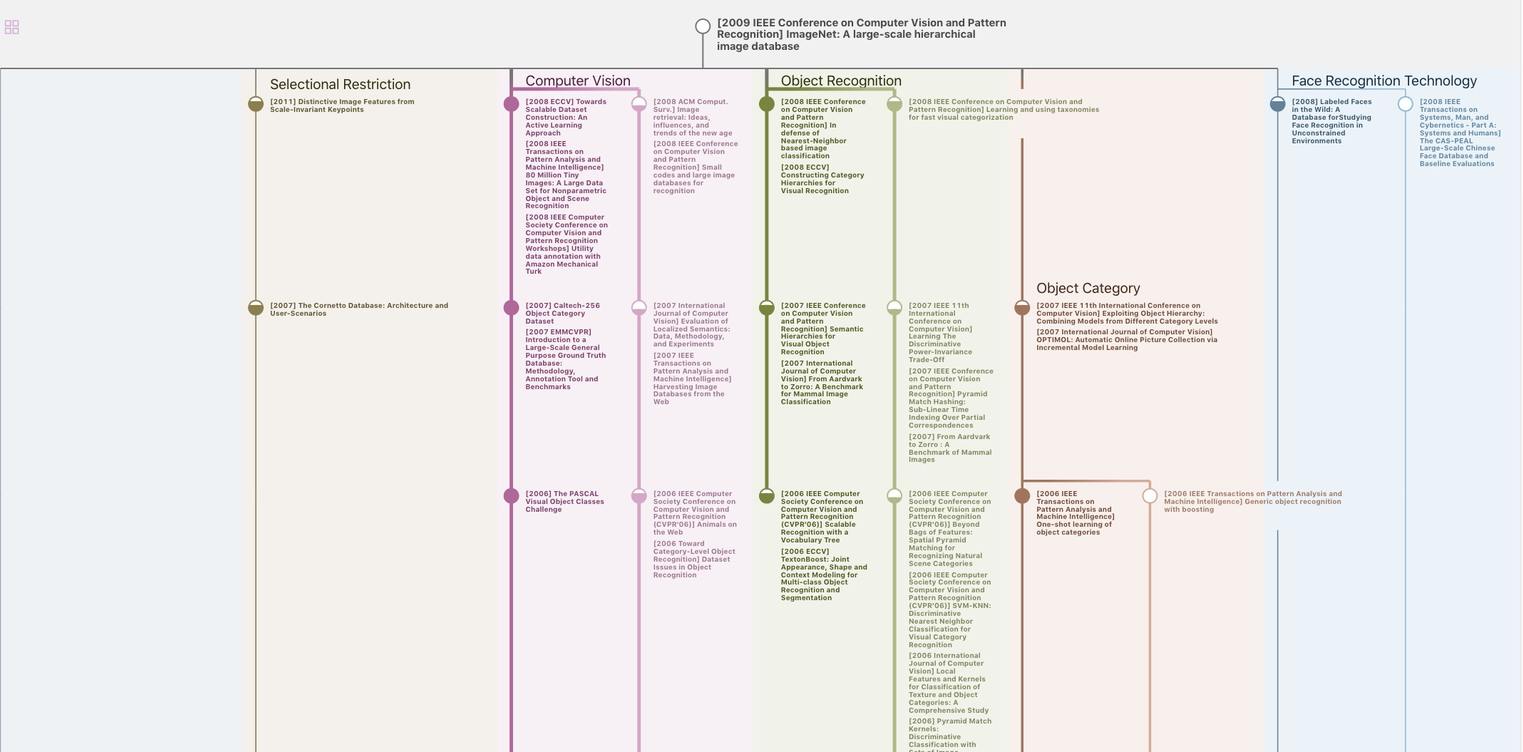
生成溯源树,研究论文发展脉络
Chat Paper
正在生成论文摘要