Posterior Annealing: Fast Calibrated Uncertainty for Regression
CoRR(2023)
Abstract
Bayesian deep learning approaches that allow uncertainty estimation for regression problems often converge slowly and yield poorly calibrated uncertainty estimates that can not be effectively used for quantification. Recently proposed post hoc calibration techniques are seldom applicable to regression problems and often add overhead to an already slow model training phase. This work presents a fast calibrated uncertainty estimation method for regression tasks, called posterior annealing, that consistently improves the convergence of deep regression models and yields calibrated uncertainty without any post hoc calibration phase. Unlike previous methods for calibrated uncertainty in regression that focus only on low-dimensional regression problems, our method works well on a wide spectrum of regression problems. Our empirical analysis shows that our approach is generalizable to various network architectures including, multilayer perceptrons, 1D/2D convolutional networks, and graph neural networks, on five vastly diverse tasks, i.e., chaotic particle trajectory denoising, physical property prediction of molecules using 3D atomistic representation, natural image super-resolution, and medical image translation using MRI images.
MoreTranslated text
AI Read Science
Must-Reading Tree
Example
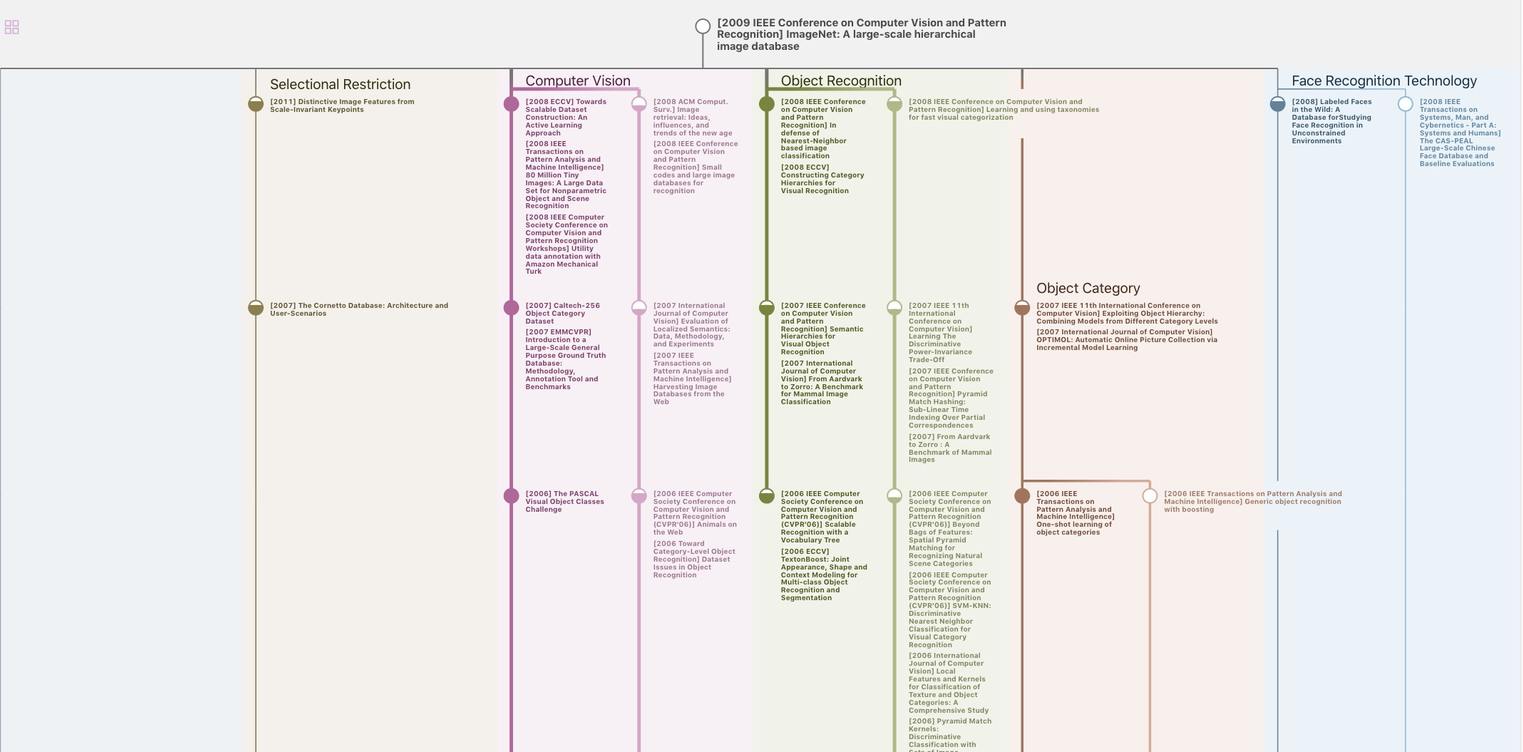
Generate MRT to find the research sequence of this paper
Chat Paper
Summary is being generated by the instructions you defined