Hashtag-Guided Low-Resource Tweet Classification
WWW 2023(2023)
摘要
Social media classification tasks (e.g., tweet sentiment analysis, tweet stance detection) are challenging because social media posts are typically short, informal, and ambiguous. Thus, training on tweets is challenging and demands large-scale human-annotated labels, which are time-consuming and costly to obtain. In this paper, we find that providing hashtags to social media tweets can help alleviate this issue because hashtags can enrich short and ambiguous tweets in terms of various information, such as topic, sentiment, and stance. This motivates us to propose a novel Hashtag-guided Tweet Classification model (HashTation), which automatically generates meaningful hashtags for the input tweet to provide useful auxiliary signals for tweet classification. To generate high-quality and insightful hashtags, our hashtag generation model retrieves and encodes the post-level and entity-level information across the whole corpus. Experiments show that HashTation achieves significant improvements on seven low-resource tweet classification tasks, in which only a limited amount of training data is provided, showing that automatically enriching tweets with model-generated hashtags could significantly reduce the demand for large-scale human-labeled data. Further analysis demonstrates that HashTation is able to generate high-quality hashtags that are consistent with the tweets and their labels. The code is available at https://github.com/shizhediao/HashTation.
更多查看译文
关键词
classification,hashtag-guided,low-resource
AI 理解论文
溯源树
样例
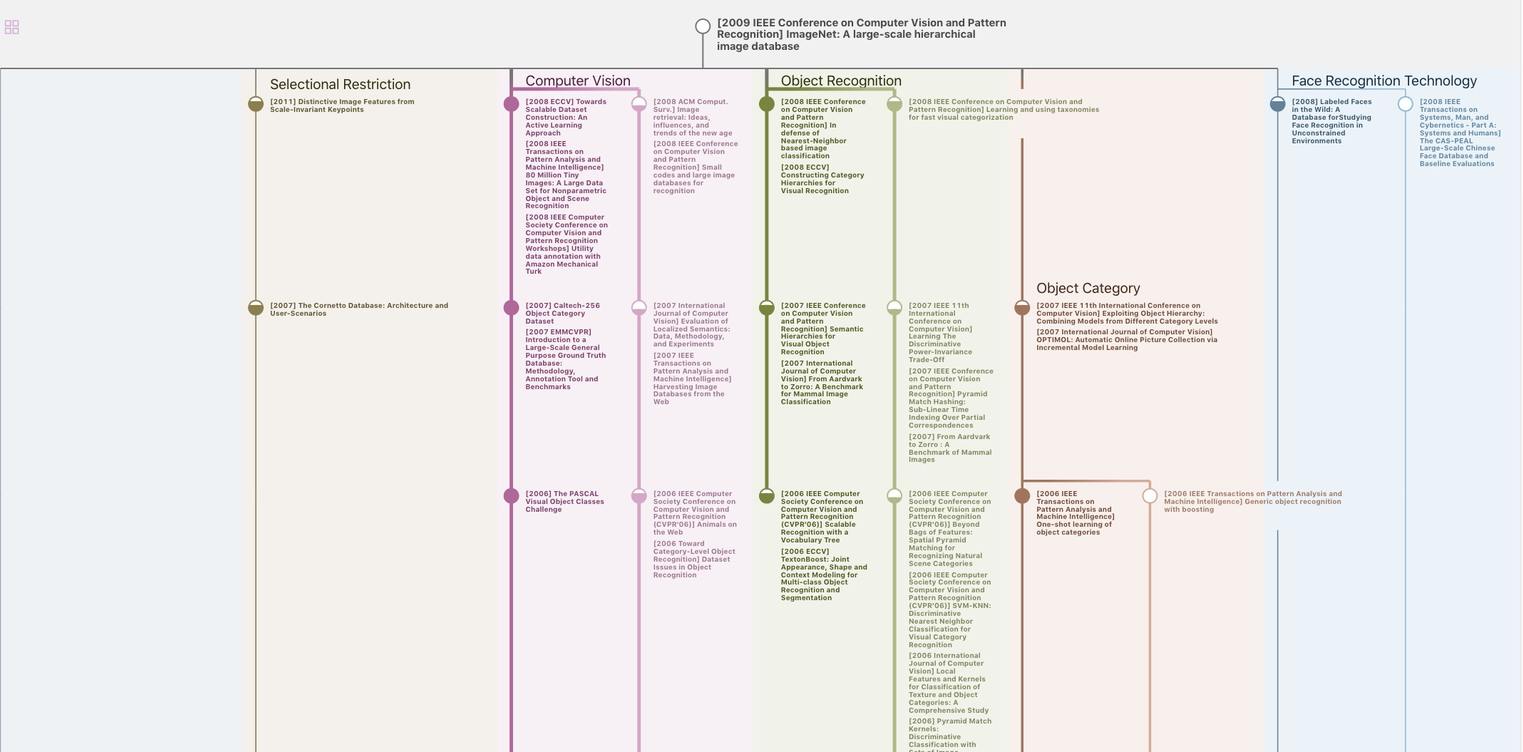
生成溯源树,研究论文发展脉络
Chat Paper
正在生成论文摘要