Yet another fast variant of Newton's method for nonconvex optimization
arxiv(2023)
摘要
A second-order algorithm is proposed for minimizing smooth nonconvex functions that alternates between regularized Newton and negative curvature steps. In most cases, the Hessian matrix is regularized with the square root of the current gradient and an additional term taking moderate negative curvature into account, a negative curvature step being taken only exceptionnally. As a consequence, the proposed method only requires the solution of a single linear system at nearly all iterations. We establish that at most $\mathcal{O}\left( |\log\epsilon|\,\epsilon^{-3/2}\right)$ evaluations of the problem's objective function and derivatives are needed for this algorithm to obtain an $\epsilon$-approximate first-order minimizer, and at most $\mathcal{O}\left(|\log\epsilon|\,\epsilon^{-3}\right)$ to obtain a second-order one. Initial numerical experiments with two variants of the new method are finally presented.
更多查看译文
关键词
nonconvex optimization,newton,fast variant
AI 理解论文
溯源树
样例
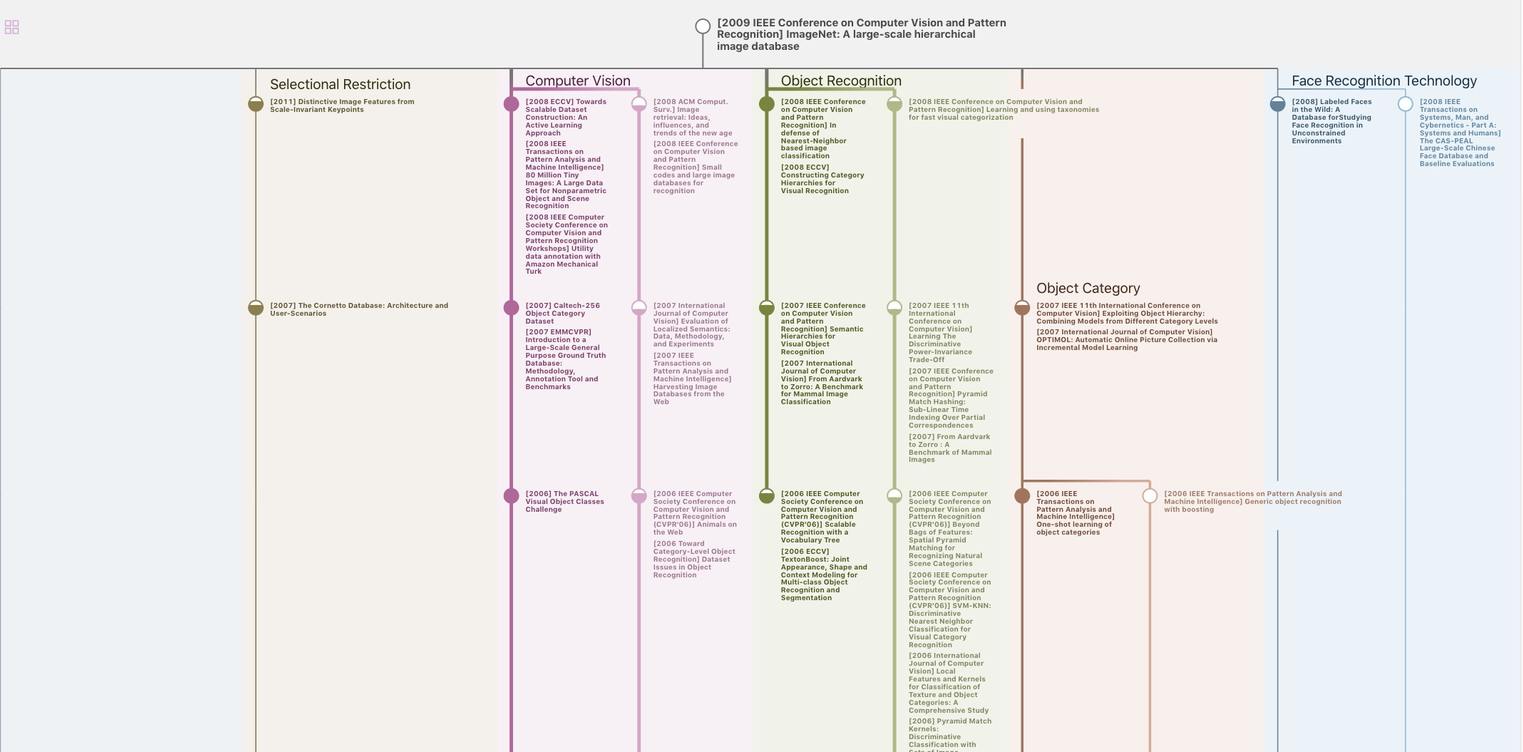
生成溯源树,研究论文发展脉络
Chat Paper
正在生成论文摘要