HOMDA: High-Order Moment-Based Domain Alignment for Unsupervised Domain Adaptation.
Knowledge-based systems(2023)
摘要
Unsupervised domain adaptation aims to annotate unlabeled target domain samples by utilizing transferable knowledge learned from the source domain. Optimal transport (OT) has become a popular probability metric to measure the distribution discrepancy across domains. However, most OT-based methods inevitably suffer from false matching problem, resulting in poor domain alignment. Furthermore, because the domain shift cannot be completely eliminated, some target domain data distributed near cluster edges or far from their corresponding class centers are highly likely to be misclassified by the decision hyperplane learned from the source samples. We propose a High-Order Moment-Based Domain Alignment (HOMDA) method that uses an improved partial optimal transport (POT) strategy and a discriminative centroid-wise clustering regularization scheme to solve these issues. The improved POT strategy performs fine-grained domain alignment by limiting the amount of masses during mapping. The centroid-wise clustering scheme can promote the shared features with better intra-class compactness and inter-class separability, effectively reducing negative transfer caused by hard-aligned target samples. To better capture discriminative representations, HOMDA also includes a high-order statistics mixer. Extensive experiments show that our proposal can improve adaptation performance significantly and is competitive with state-of-the-art methods.
更多查看译文
关键词
Transfer learning,Domain adaptation,Optimal transport,High-order moment,Discriminative feature learning
AI 理解论文
溯源树
样例
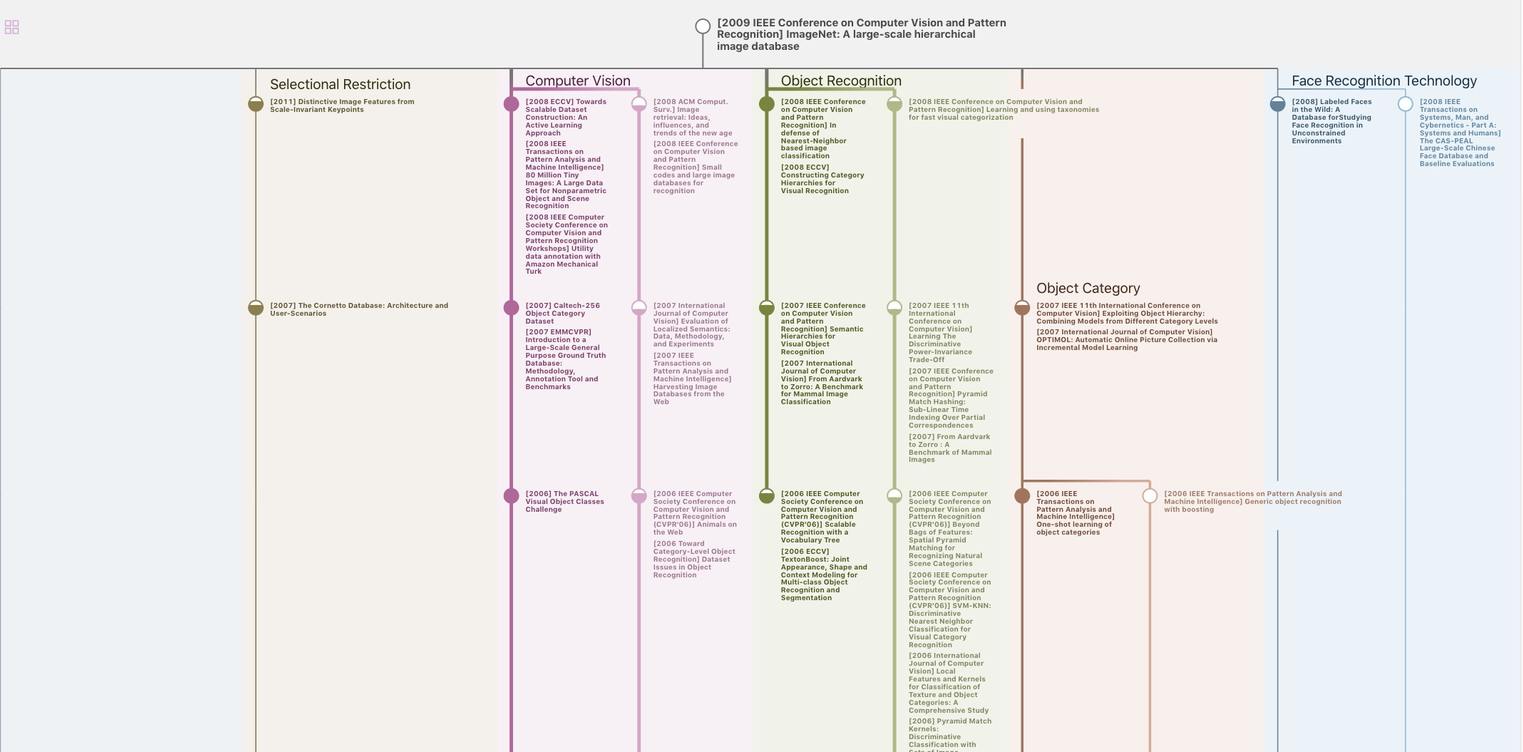
生成溯源树,研究论文发展脉络
Chat Paper
正在生成论文摘要