Robust Bayesian Target Value Optimization
CoRR(2023)
摘要
We consider the problem of finding an input to a stochastic black box function such that the scalar output of the black box function is as close as possible to a target value in the sense of the expected squared error. While the optimization of stochastic black boxes is classic in (robust) Bayesian optimization, the current approaches based on Gaussian processes predominantly focus either on i) maximization/minimization rather than target value optimization or ii) on the expectation, but not the variance of the output, ignoring output variations due to stochasticity in uncontrollable environmental variables. In this work, we fill this gap and derive acquisition functions for common criteria such as the expected improvement, the probability of improvement, and the lower confidence bound, assuming that aleatoric effects are Gaussian with known variance. Our experiments illustrate that this setting is compatible with certain extensions of Gaussian processes, and show that the thus derived acquisition functions can outperform classical Bayesian optimization even if the latter assumptions are violated. An industrial use case in billet forging is presented.
更多查看译文
关键词
Bayesian optimization,Aleatoric uncertainty,Target vector optimization,Gaussian process
AI 理解论文
溯源树
样例
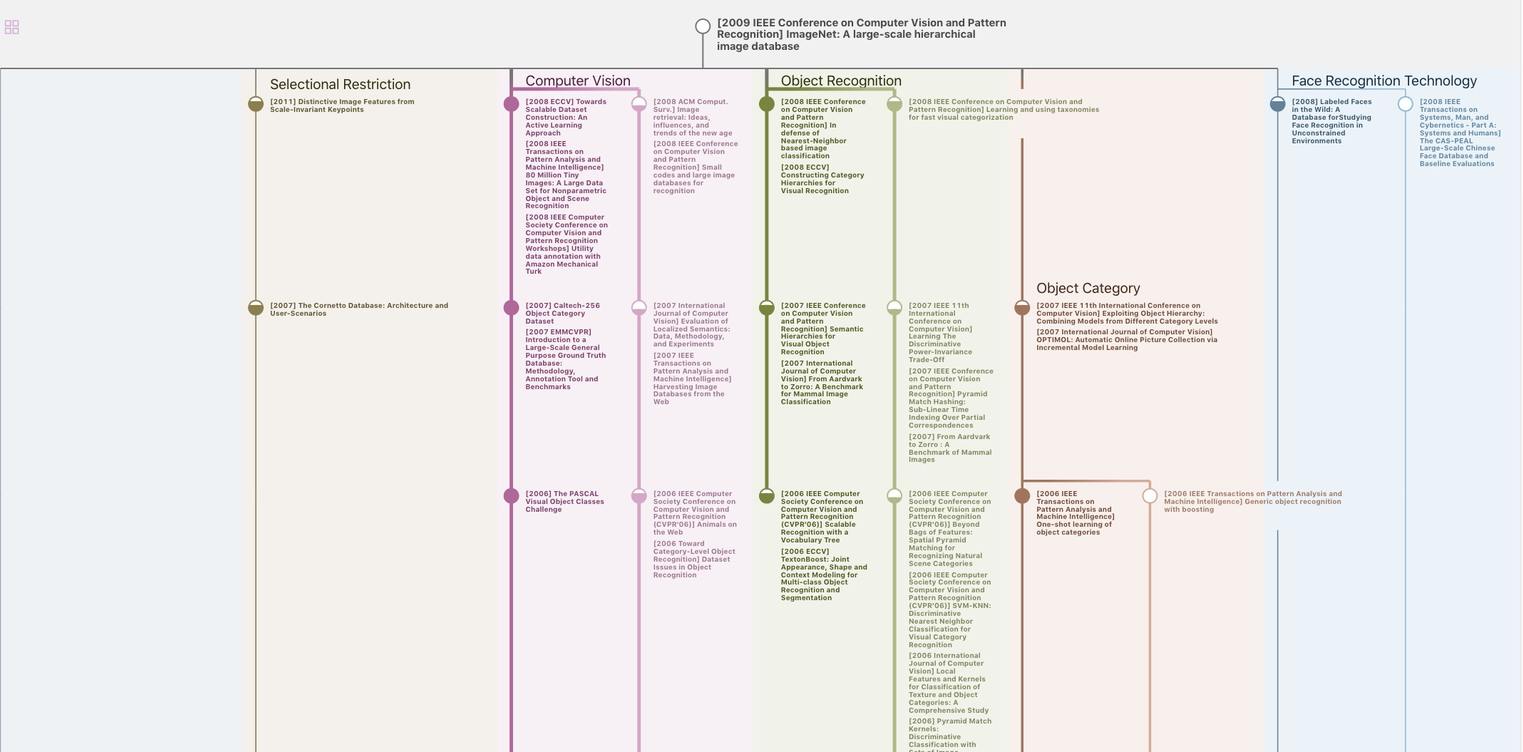
生成溯源树,研究论文发展脉络
Chat Paper
正在生成论文摘要