LWS: A Framework for Log-based Workload Simulation in Session-based SUT
CoRR(2023)
Abstract
Artificial intelligence for IT Operations (AIOps) plays a critical role in operating and managing cloud-native systems and microservice-based applications but is limited by the lack of high-quality datasets with diverse scenarios. Realistic workloads are the premise and basis of generating such AIOps datasets, with the session-based workload being one of the most typical examples. Due to privacy concerns, complexity, variety, and requirements for reasonable intervention, it is difficult to copy or generate such workloads directly, showing the importance of effective and intervenable workload simulation. In this paper, we formulate the task of workload simulation and propose a framework for Log-based Workload Simulation (LWS) in session-based systems. LWS extracts the workload specification including the user behavior abstraction based on agglomerative clustering as well as relational models and the intervenable workload intensity from session logs. Then LWS combines the user behavior abstraction with the workload intensity to generate simulated workloads. The experimental evaluation is performed on an open-source cloud-native application with both well-designed and public real-world workloads, showing that the simulated workload generated by LWS is effective and intervenable, which provides the foundation of generating high-quality AIOps datasets.
MoreTranslated text
Key words
workload simulation,sut,log-based,session-based
AI Read Science
Must-Reading Tree
Example
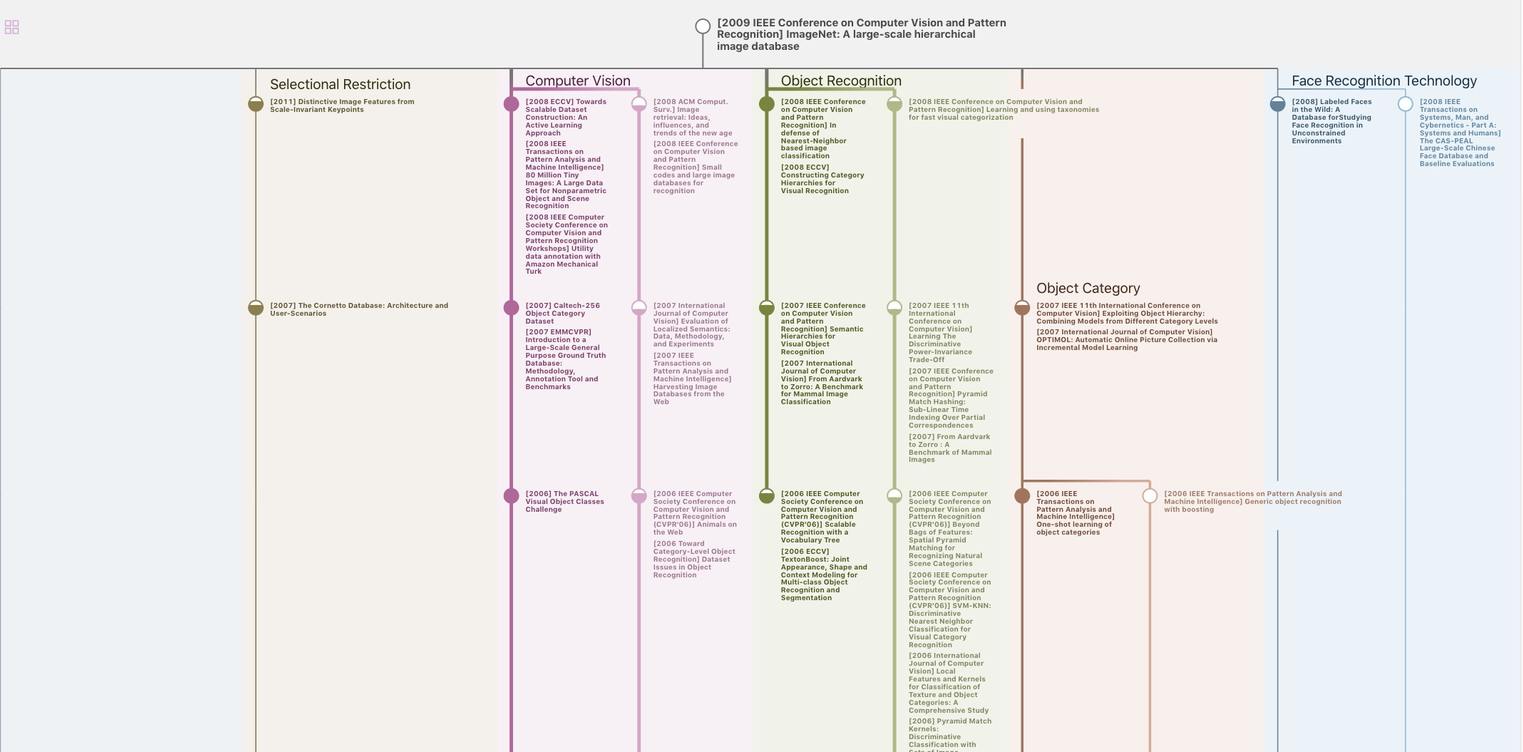
Generate MRT to find the research sequence of this paper
Chat Paper
Summary is being generated by the instructions you defined