Physics-based parameterized neural ordinary differential equations: prediction of laser ignition in a rocket combustor
arxiv(2023)
摘要
In this work, we present a novel physics-based data-driven framework for reduced-order modeling of laser ignition in a model rocket combustor based on parameterized neural ordinary differential equations (PNODE). Deep neural networks are embedded as functions of high-dimensional parameters of laser ignition to predict various terms in a 0D flow model including the heat source function, pre-exponential factors, and activation energy. Using the governing equations of a 0D flow model, our PNODE needs only a limited number of training samples and predicts trajectories of various quantities such as temperature, pressure, and mass fractions of species while satisfying physical constraints. We validate our physics-based PNODE on solution snapshots of high-fidelity Computational Fluid Dynamics (CFD) simulations of laser-induced ignition in a prototype rocket combustor. We compare the performance of our physics-based PNODE with that of kernel ridge regression and fully connected neural networks. Our results show that our physics-based PNODE provides solutions with lower mean absolute errors of average temperature over time, thus improving the prediction of successful laser ignition with high-dimensional parameters.
更多查看译文
关键词
neural ordinary differential equations,rocket combustor,laser ignition,differential equations,physics-based
AI 理解论文
溯源树
样例
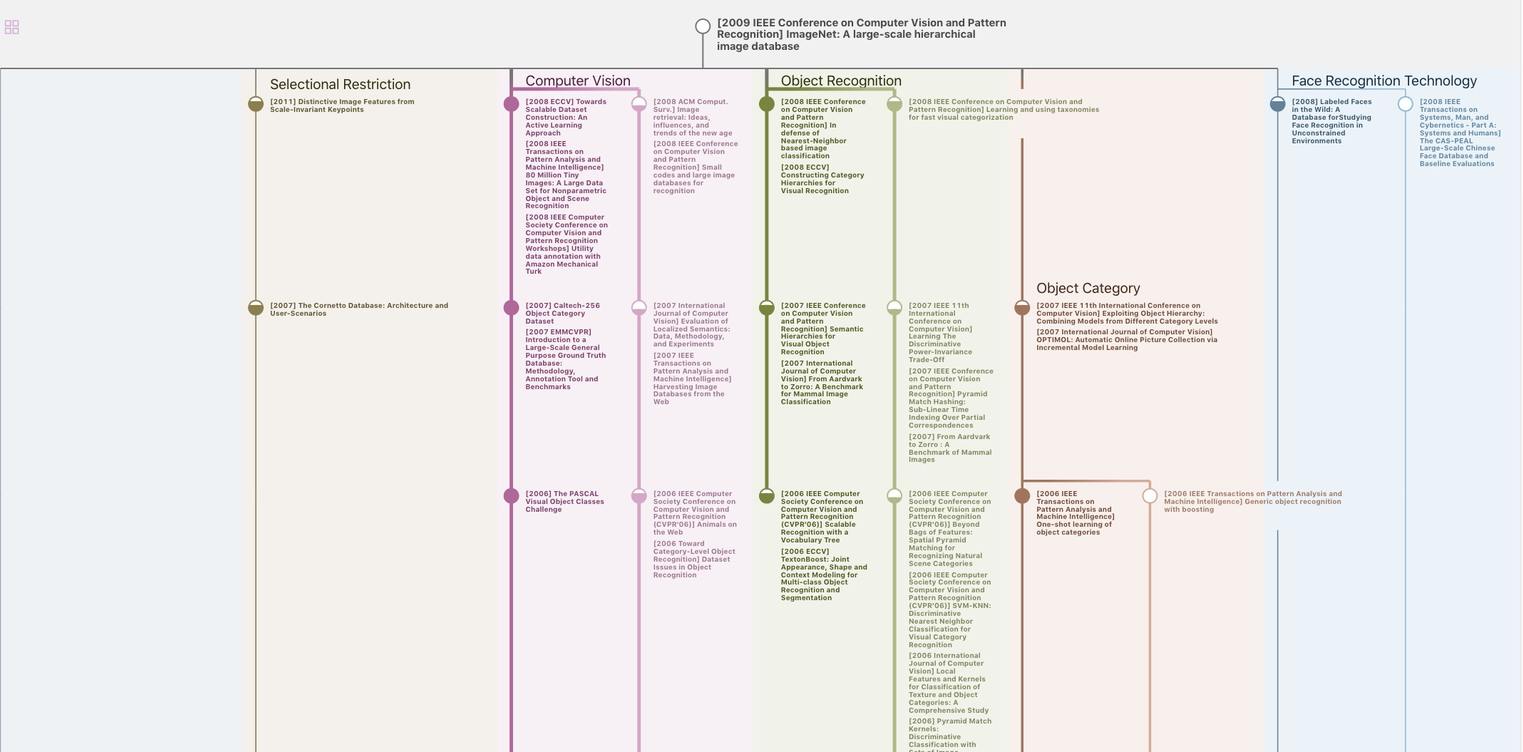
生成溯源树,研究论文发展脉络
Chat Paper
正在生成论文摘要