With Shared Microexponents, A Little Shifting Goes a Long Way
PROCEEDINGS OF THE 2023 THE 50TH ANNUAL INTERNATIONAL SYMPOSIUM ON COMPUTER ARCHITECTURE, ISCA 2023(2023)
摘要
This paper introduces Block Data Representations (BDR), a framework for exploring and evaluating a wide spectrum of narrow-precision formats for deep learning. It enables comparison of popular quantization standards, and through BDR, new formats based on shared microexponents (MX) are identified, which outperform other state-of-the-art quantization approaches, including narrow-precision floating-point and block floating-point. MX utilizes multiple levels of quantization scaling with ultra-fine scaling factors based on shared microexponents in the hardware. The effectiveness of MX is demonstrated on real-world models including large-scale generative pretraining and inferencing, and production-scale recommendation systems.
更多查看译文
关键词
Artificial Intelligence,Compute Efficiency,AI Data Types
AI 理解论文
溯源树
样例
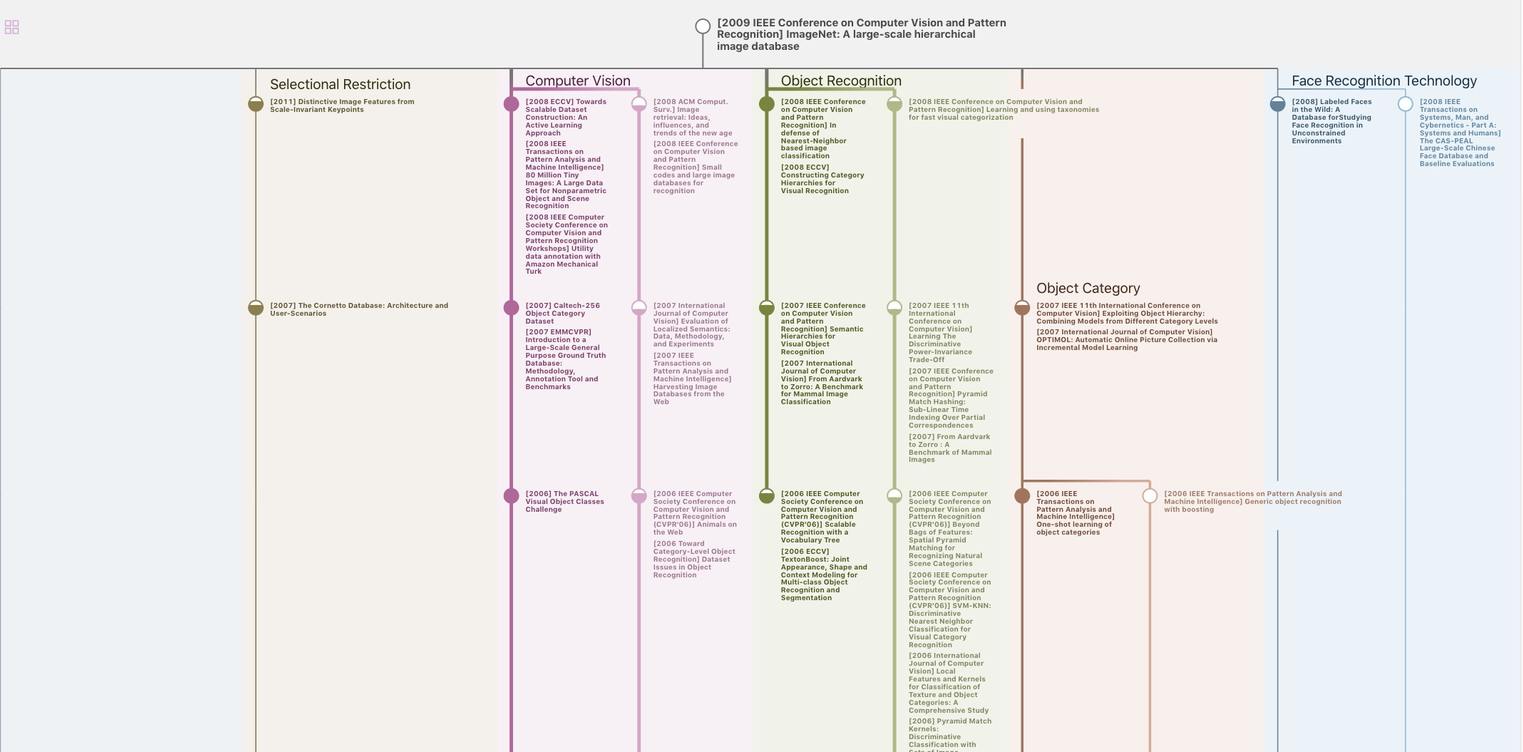
生成溯源树,研究论文发展脉络
Chat Paper
正在生成论文摘要