NEAREST SUBSPACE SEARCH IN THE SIGNED CUMULATIVE DISTRIBUTION TRANSFORM SPACE FOR 1D SIGNAL CLASSIFICATION
2022 IEEE INTERNATIONAL CONFERENCE ON ACOUSTICS, SPEECH AND SIGNAL PROCESSING (ICASSP)(2022)
摘要
This paper presents a new method to classify 1D signals using the signed cumulative distribution transform (SCDT). The proposed method exploits certain linearization properties of the SCDT to render the problem easier to solve in the SCDT space. The method uses the nearest subspace search technique in the SCDT domain to provide a non-iterative, effective, and simple to implement classification algorithm. Experiments show that the proposed technique outperforms the state-of-the-art neural networks using a very low number of training samples and is also robust to out-of-distribution examples on simulated data. We also demonstrate the efficacy of the proposed technique in real-world applications by applying it to an ECG classification problem.
更多查看译文
关键词
SCDT,nearest subspace,1D signal classification,generative model
AI 理解论文
溯源树
样例
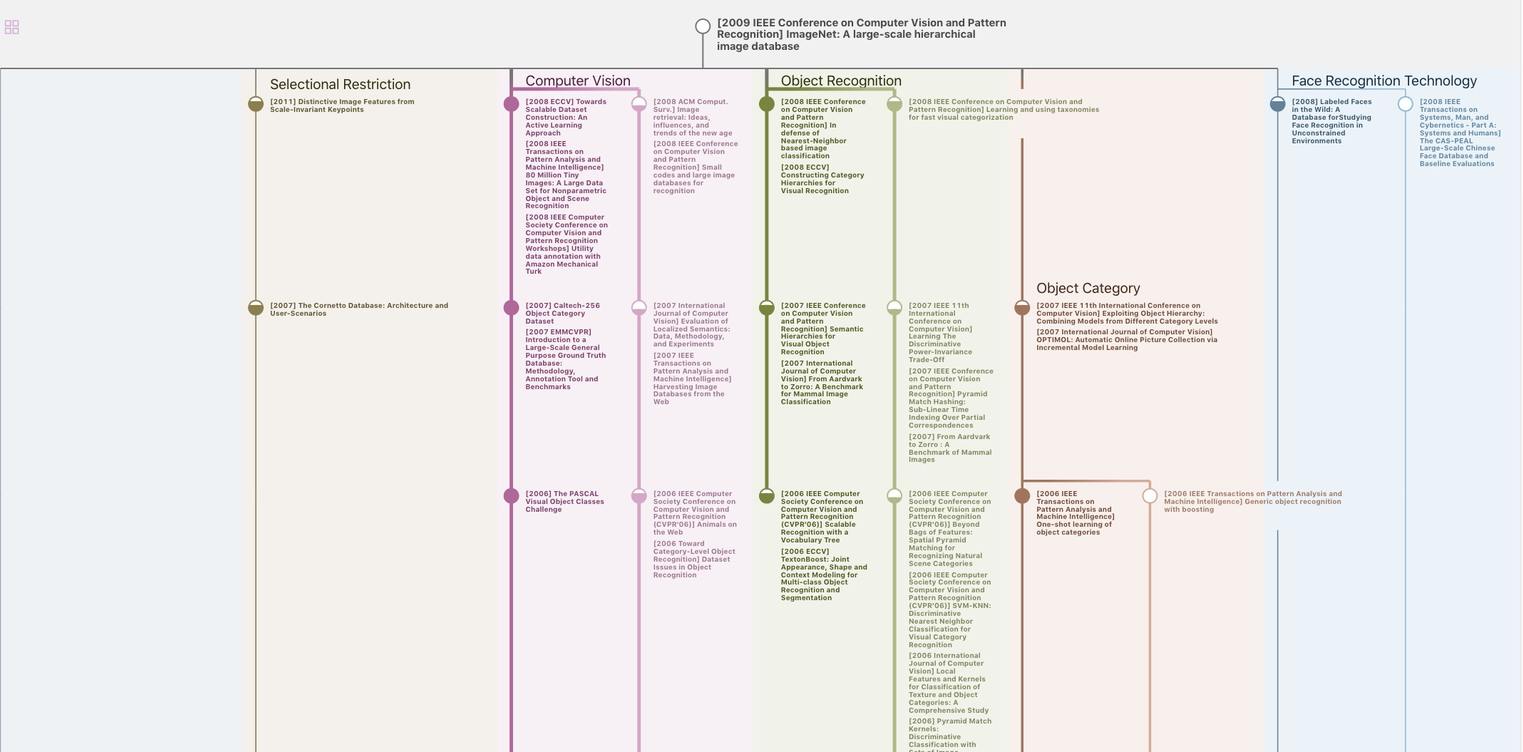
生成溯源树,研究论文发展脉络
Chat Paper
正在生成论文摘要