Parametric Estimation of Equipment Failure Risk with Machine Learning and Constrained Optimization
JOURNAL OF PERFORMANCE OF CONSTRUCTED FACILITIES(2023)
摘要
Building equipment failures can have a significant impact on facility operations and performance. Accurately estimating this risk of failure is a crucial aspect of maintenance decision-making for facility managers. However, developing a reliable failure model typically requires a substantial data set of past failures, which is often unavailable or not applicable for components operating in situ. This paper presents a novel machine learning method to estimate equipment failure probabilities based on more easily obtained condition inspection observations rather than relying on limited failure event data sets. The method starts with a neural network to develop an accurate degradation model from equipment inspection data. Next, the model generates estimated failure ages from known equipment condition states, thus alleviating the reliance on observed equipment failures. This paper proposes a new failure likelihood function, which is used to select parameters to characterize the failure process. The resulting parametric model can estimate failure probabilities for a given component based on time in service. This method is validated against various types of building equipment, and a case study demonstrates the use of the model to plan repair operations under failure risk uncertainty.
更多查看译文
关键词
Failure probability,Machine learning,Equipment degradation,Neural networks
AI 理解论文
溯源树
样例
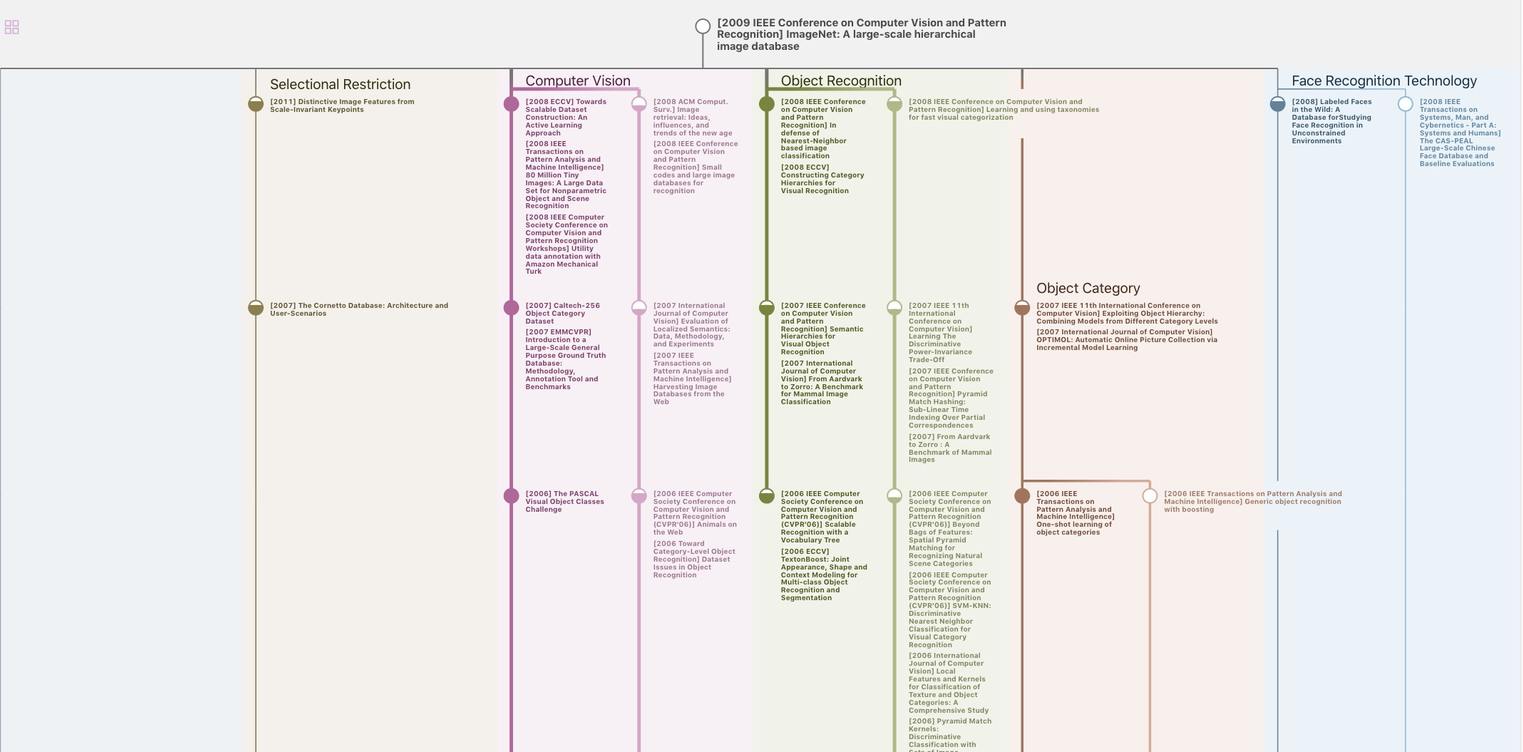
生成溯源树,研究论文发展脉络
Chat Paper
正在生成论文摘要