A Proportional Expected Improvement Criterion-Based Multi-Fidelity Sequential Optimization Method
Structural and multidisciplinary optimization(2023)
摘要
Multi-fidelity surrogate models fusing data from different fidelity systems can significantly reduce the computational cost while ensuring the model accuracy. The focus of this paper is on the sequential design for multi-fidelity models for expensive black-box problem. A Co-kriging-based multi-fidelity sequential optimization method named proportional expected improvement (PEI) is proposed with the objection to be more efficient for global optimization and to be more reasonable to evaluate the costs and benefits of candidate points from different levels of fidelity. The PEI method is an extension of expected improvement (EI) and uses an integrated criterion to determine both location and fidelity level of the subsequent. In the integrated criterion, a proportional factor which is adaptively adjusted according to the sample density is added in EI to adjust the tendency between exploration and exploitation during the search process. Meanwhile, Kullback–Leibler divergence is used to measure the credibility of a point from system with different fidelities, and the cost and constraint of different fidelities are also considered. The effectiveness and advantage of the proposed method were demonstrated by seven analytical functions and then applied to the aerodynamic shape optimization of NACA0012 airfoil. Experiments show that the proportional factor makes the proposed algorithm better search for the global optimum, and the KL divergence can describe the relationship between high and low fidelity more significantly.
更多查看译文
关键词
Co-Kriging,Multi-fidelity,Expected improvement,Sequential sampling,Kullback–Leibler divergence
AI 理解论文
溯源树
样例
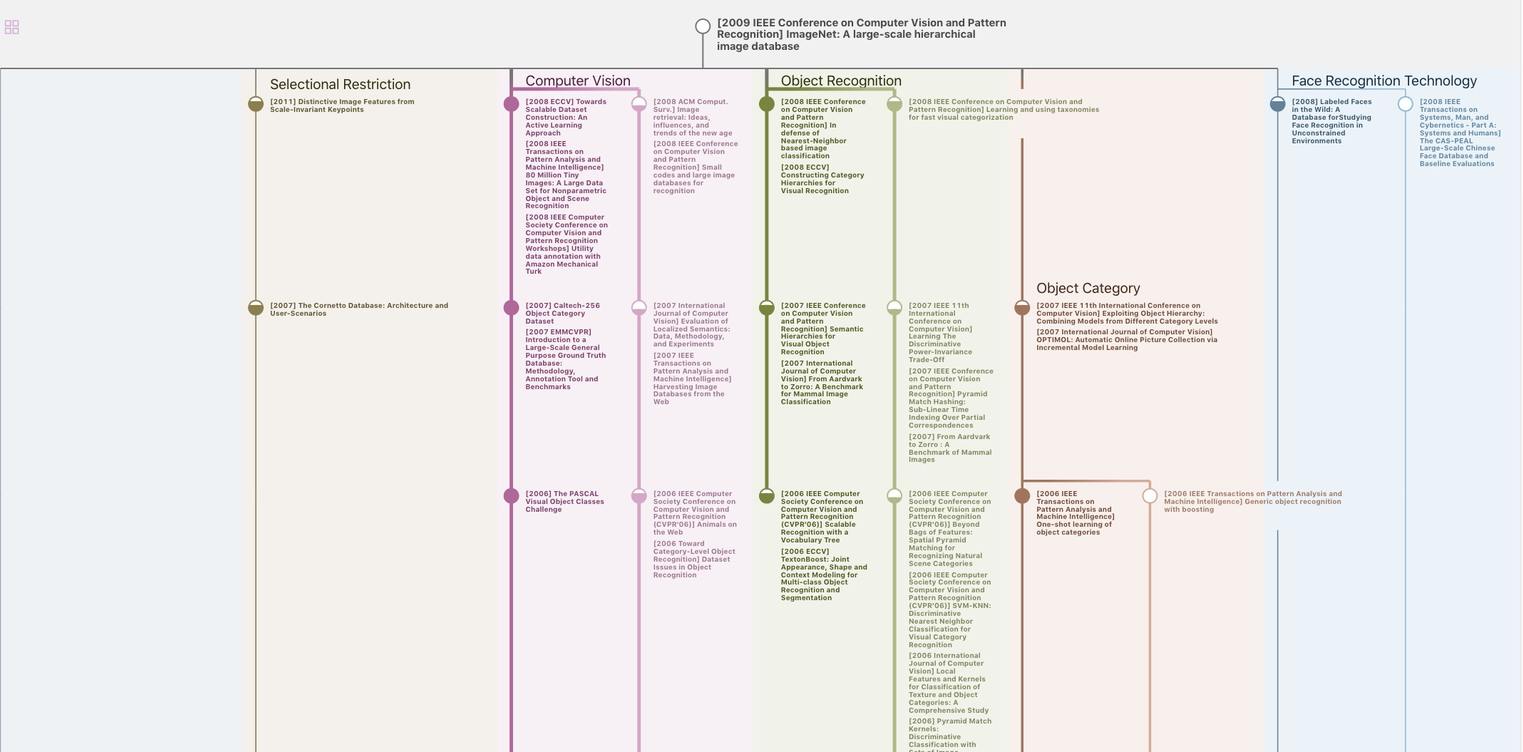
生成溯源树,研究论文发展脉络
Chat Paper
正在生成论文摘要