A Fast, Low-Cost and Simple Method for Predicting Atomic/Inter-Atomic Properties by Combining a Low Dimensional Deep Learning Model with a Fragment Based Graph Convolutional Network
CRYSTALS(2022)
摘要
Calculations with high accuracy for atomic and inter-atomic properties, such as nuclear magnetic resonance (NMR) spectroscopy and bond dissociation energies (BDEs) are valuable for pharmaceutical molecule structural analysis, drug exploration, and screening. It is important that these calculations should include relativistic effects, which are computationally expensive to treat. Non-relativistic calculations are less expensive but their results are less accurate. In this study, we present a computational framework for predicting atomic and inter-atomic properties by using machine-learning in a non-relativistic but accurate and computationally inexpensive framework. The accurate atomic and inter-atomic properties are obtained with a low dimensional deep neural network (DNN) embedded in a fragment-based graph convolutional neural network (F-GCN). The F-GCN acts as an atomic fingerprint generator that converts the atomistic local environments into data for the DNN, which improves the learning ability, resulting in accurate results as compared to experiments. Using this framework, the C-13/H-1 NMR chemical shifts of Nevirapine and phenol O-H BDEs are predicted to be in good agreement with experimental measurement.
更多查看译文
关键词
quantum mechanics,neural network,NMR,bond dissociation energy,machine-learning
AI 理解论文
溯源树
样例
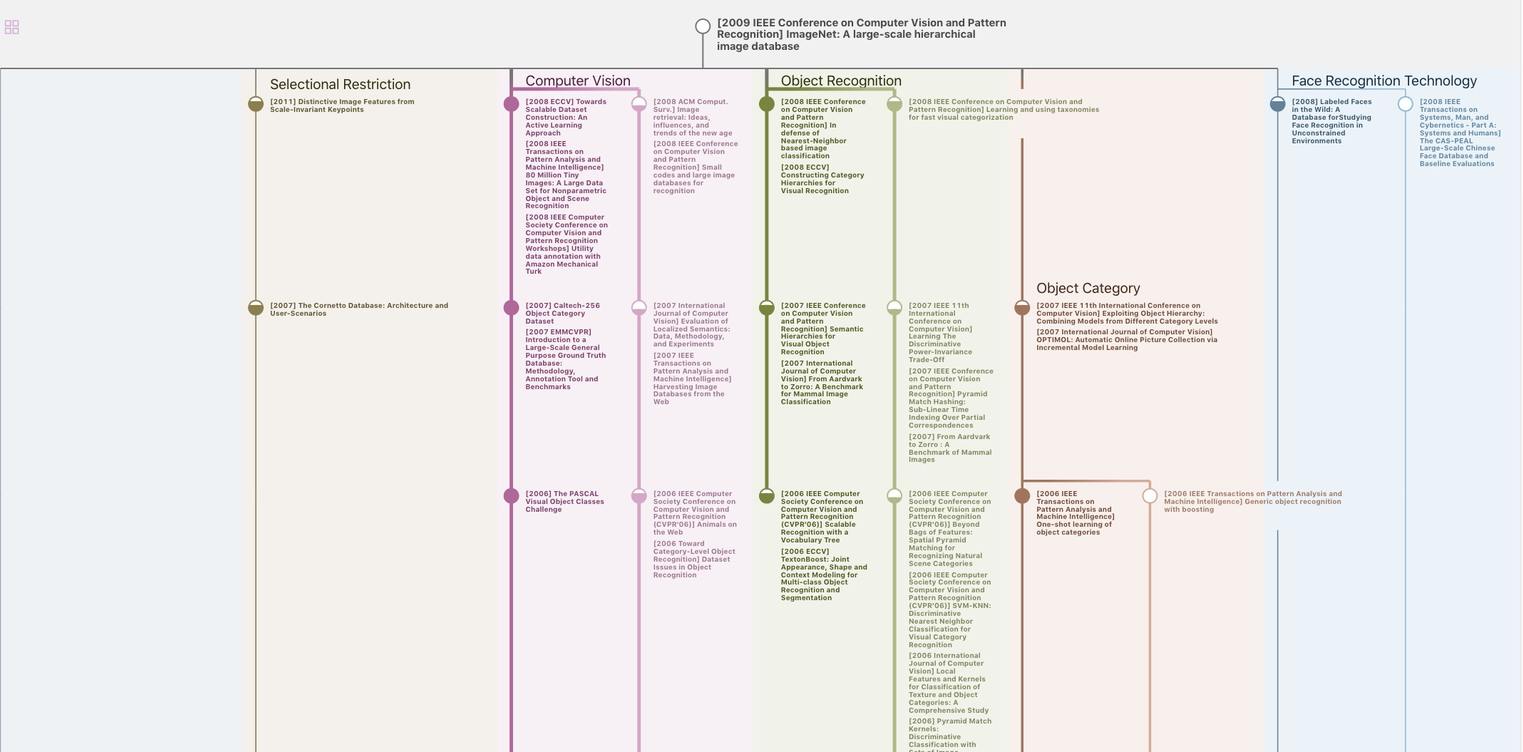
生成溯源树,研究论文发展脉络
Chat Paper
正在生成论文摘要