Online sparse sliced inverse regression for high-dimensional streaming data
INTERNATIONAL JOURNAL OF WAVELETS MULTIRESOLUTION AND INFORMATION PROCESSING(2023)
摘要
Due to the demand for tackling the problem of streaming data with high-dimensional covarites, we propose an online sparse sliced inverse regression (OSSIR) method for online sufficient dimension reduction (SDR). The existing online SDR methods focus on the case when p (dimension of covariates) is small. In this paper, we adapt the sparse sliced inverse regression to cope with high-dimensional streaming data where the dimension p is large. There are two important steps in our method, one is to extend the online principal component analysis to iteratively obtain the eigenvalues and eigenvectors of the kernel matrix, the other is to use the truncated gradient to perform online L-1 regularization. Theoretical properties of the proposed online learner are established. By comparing with several existing methods in simulations and real data applications, we demonstrate the effectiveness and efficiency of our method.
更多查看译文
关键词
Sliced inverse regression,online learning,online PCA,sparsity,truncated gradient
AI 理解论文
溯源树
样例
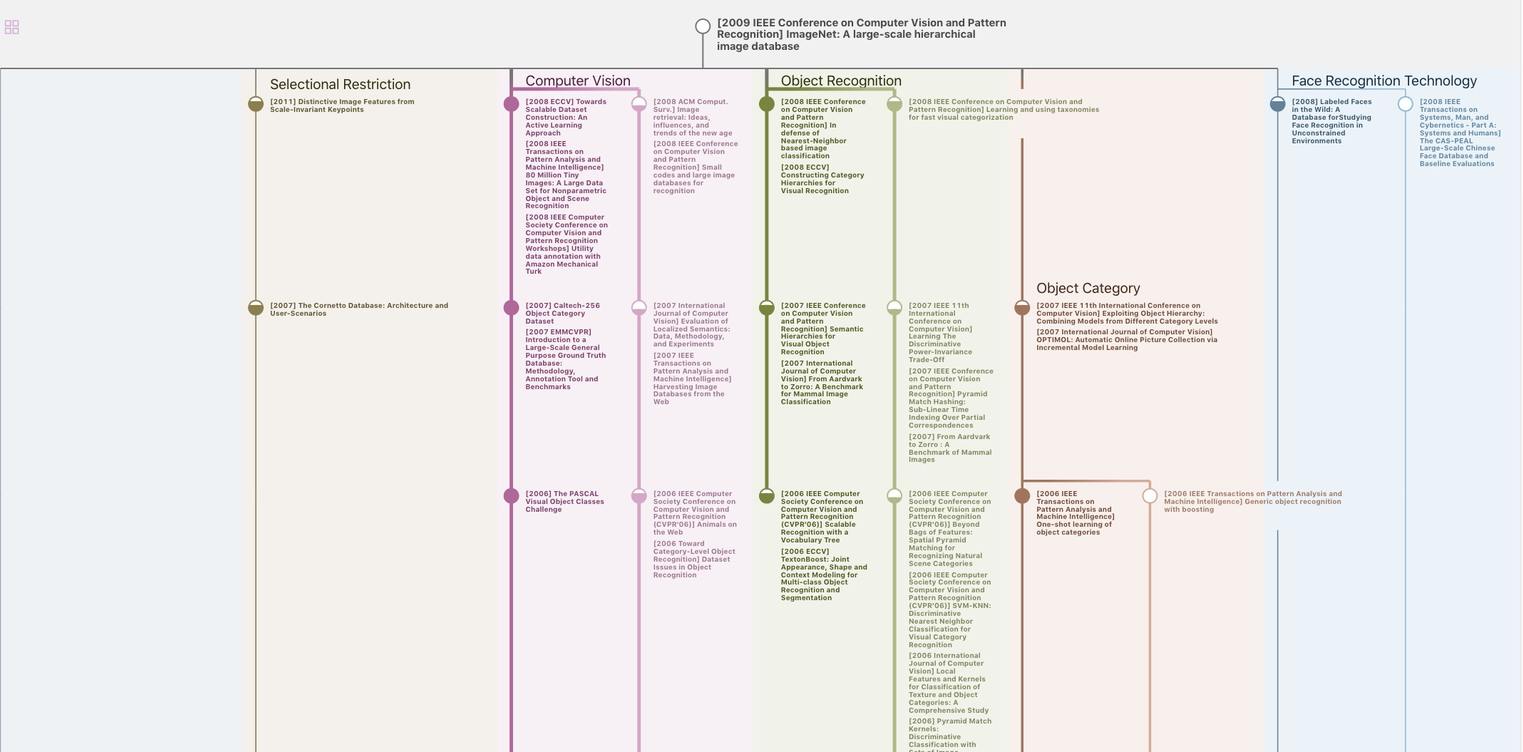
生成溯源树,研究论文发展脉络
Chat Paper
正在生成论文摘要