Key Design Choices for Double-Transfer in Source-Free Unsupervised Domain Adaptation
ICLR 2023(2023)
摘要
Fine-tuning and Domain Adaptation emerged as effective strategies for efficiently transferring deep learning models to new target tasks. However, target domain labels are not accessible in many real-world scenarios. This led to the development of Unsupervised Domain Adaptation (UDA) methods, which only employ unlabeled target samples. Furthermore, efficiency and privacy requirements may also prevent the use of source domain data during the adaptation stage. This challenging setting, known as Source-Free Unsupervised Domain Adaptation (SF-UDA), is gaining interest among researchers and practitioners due to its potential for real-world applications. In this paper, we provide the first in-depth analysis of the main design choices in SF-UDA through a large-scale empirical study across 500 models and 74 domain pairs. We pinpoint the normalization approach, pre-training strategy, and backbone architecture as the most critical factors. Based on our quantitative findings, we propose recipes to best tackle SF-UDA scenarios. Moreover, we show that SF-UDA is competitive also beyond standard benchmarks and backbone architectures, performing on par with UDA at a fraction of the data and computational cost. In the interest of reproducibility, we include the full experimental results and code as supplementary material.
更多查看译文
关键词
Transfer Learning,Unsupervised Domain Adaptation
AI 理解论文
溯源树
样例
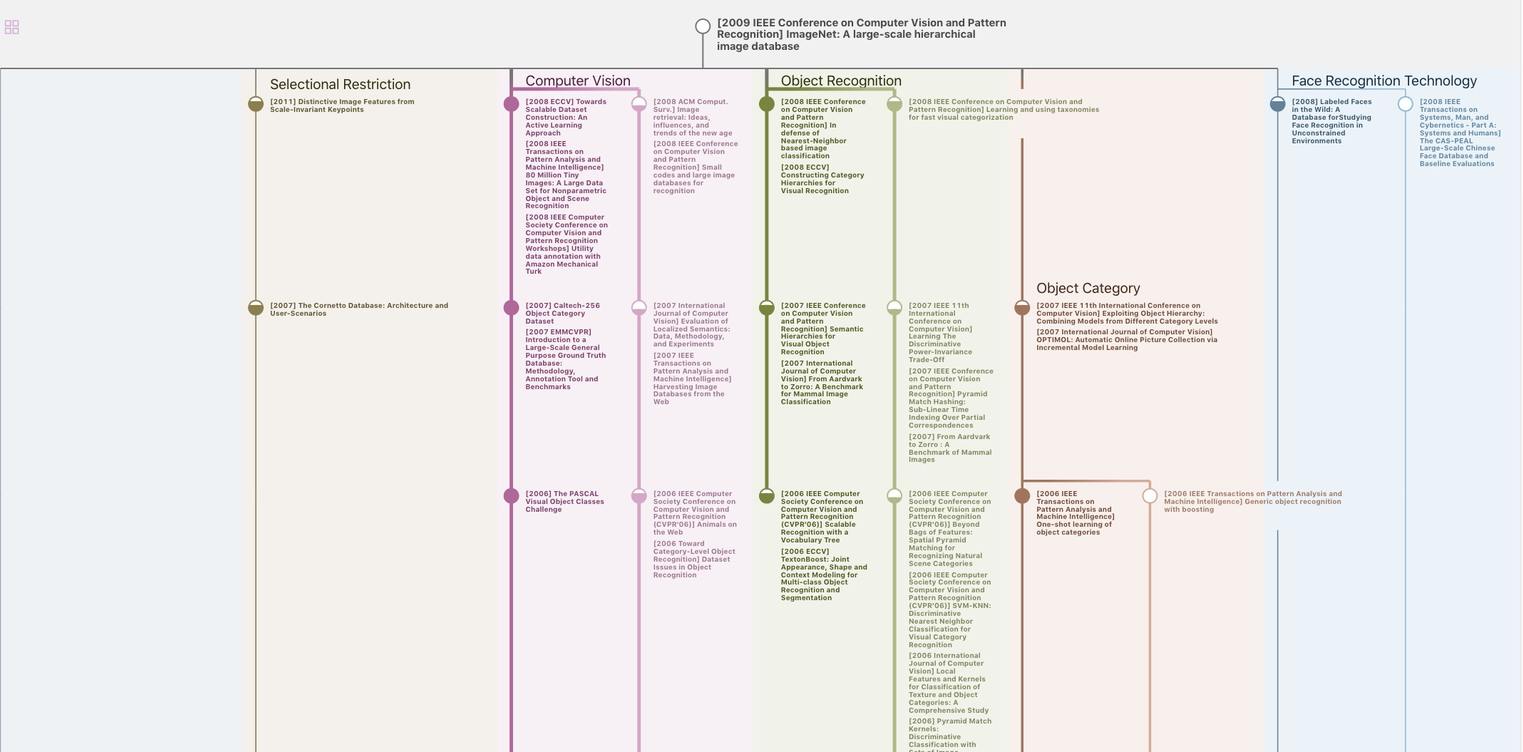
生成溯源树,研究论文发展脉络
Chat Paper
正在生成论文摘要