Sensitivity of Fecal Immunochemical Test and Risk Factors for Interval Colorectal Cancer in a French Population
Clinics and Research in Hepatology and Gastroenterology(2023)SCI 4区
Brest Univ Hosp | CRCDC BRETAGNE | CHU Cavale Blanche
Abstract
Background: Colorectal cancer (CRC) screening using fecal immunochemical testing (FIT) aims to detect pre-symptomatic colorectal lesions and reduce CRC mortality. Aims: The objectives of this study were to determine the FIT sensitivity for diagnosis of CRC, the impact of diagnostic circumstances on treatment and survival, and risk factors for interval cancer (IC). Methods: This population-based study evaluated the 2016-2017 CRC screening campaign in Finistere, France. CRCs were classified according to diagnostic circumstances: screen-detected CRC (SD-CRC), CRC with delayed diagnosis, IC after negative FIT (FIT-IC), post-colonoscopy CRC, CRC in non-responders and CRC in the excluded population. Results: This study included 909 CRCs: 248 SD-CRCs (6% of positive FIT) and 60 FIT-ICs (0.07% of negative FIT). The FIT sensitivity for CRC was 80.5% (CI95%: 76.1-84.9) at the threshold of 30 mg hemoglobin/g feces used in France. In multivariate analysis, proximal (OR:6.73) and rectal locations (OR:7.52) were associated with being diagnosed with FIT-IC rather than SD-CRC. The FIT positivity threshold maximizing the sum of sensitivity and specificity was found to be 17 mg/g, with 14 additional CRCs diagnosed compared to the current threshold. Conclusions: Our study confirms the good sensitivity of FIT. A decrease of the FIT detection threshold could optimize sensitivity. (c) 2023 Elsevier Masson SAS. All rights reserved.
MoreTranslated text
Key words
Colorectal cancer,Interval cancer,Fecal immunochemical test,Screening
求助PDF
上传PDF
View via Publisher
AI Read Science
AI Summary
AI Summary is the key point extracted automatically understanding the full text of the paper, including the background, methods, results, conclusions, icons and other key content, so that you can get the outline of the paper at a glance.
Example
Background
Key content
Introduction
Methods
Results
Related work
Fund
Key content
- Pretraining has recently greatly promoted the development of natural language processing (NLP)
- We show that M6 outperforms the baselines in multimodal downstream tasks, and the large M6 with 10 parameters can reach a better performance
- We propose a method called M6 that is able to process information of multiple modalities and perform both single-modal and cross-modal understanding and generation
- The model is scaled to large model with 10 billion parameters with sophisticated deployment, and the 10 -parameter M6-large is the largest pretrained model in Chinese
- Experimental results show that our proposed M6 outperforms the baseline in a number of downstream tasks concerning both single modality and multiple modalities We will continue the pretraining of extremely large models by increasing data to explore the limit of its performance
Upload PDF to Generate Summary
Must-Reading Tree
Example
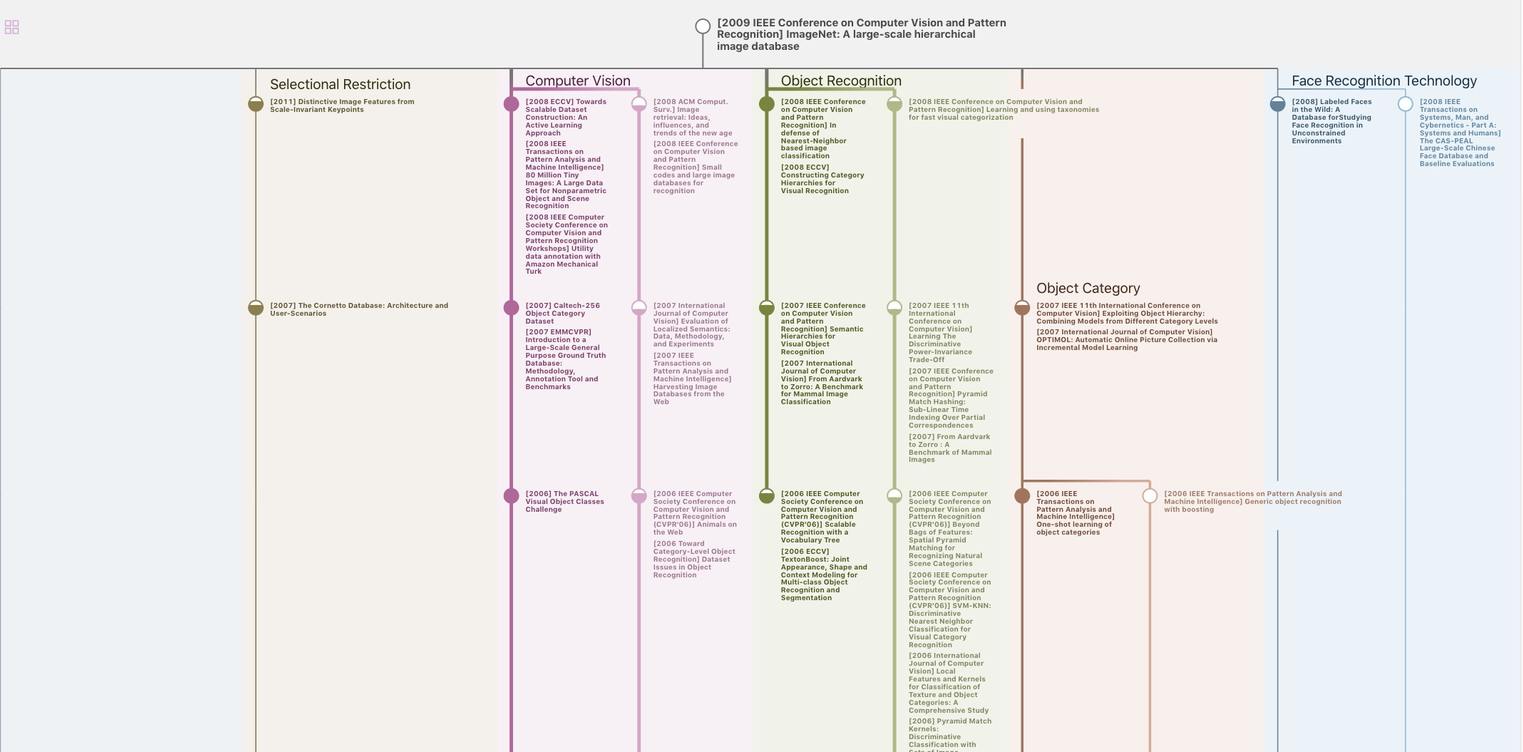
Generate MRT to find the research sequence of this paper
Related Papers
2008
被引用50 | 浏览
2009
被引用14 | 浏览
2001
被引用111 | 浏览
2008
被引用142 | 浏览
Data Disclaimer
The page data are from open Internet sources, cooperative publishers and automatic analysis results through AI technology. We do not make any commitments and guarantees for the validity, accuracy, correctness, reliability, completeness and timeliness of the page data. If you have any questions, please contact us by email: report@aminer.cn
Chat Paper