One-shot Empirical Privacy Estimation for Federated Learning
ICLR 2024(2024)
摘要
Privacy estimation techniques for differentially private (DP) algorithms areuseful for comparing against analytical bounds, or to empirically measureprivacy loss in settings where known analytical bounds are not tight. However,existing privacy auditing techniques usually make strong assumptions on theadversary (e.g., knowledge of intermediate model iterates or the training datadistribution), are tailored to specific tasks, model architectures, or DPalgorithm, and/or require retraining the model many times (typically on theorder of thousands). These shortcomings make deploying such techniques at scaledifficult in practice, especially in federated settings where model trainingcan take days or weeks. In this work, we present a novel "one-shot" approachthat can systematically address these challenges, allowing efficient auditingor estimation of the privacy loss of a model during the same, single trainingrun used to fit model parameters, and without requiring any a priori knowledgeabout the model architecture, task, or DP training algorithm. We show that ourmethod provides provably correct estimates for the privacy loss under theGaussian mechanism, and we demonstrate its performance on well-established FLbenchmark datasets under several adversarial threat models.
更多查看译文
关键词
differential privacy,federated learning,empirical privacy
AI 理解论文
溯源树
样例
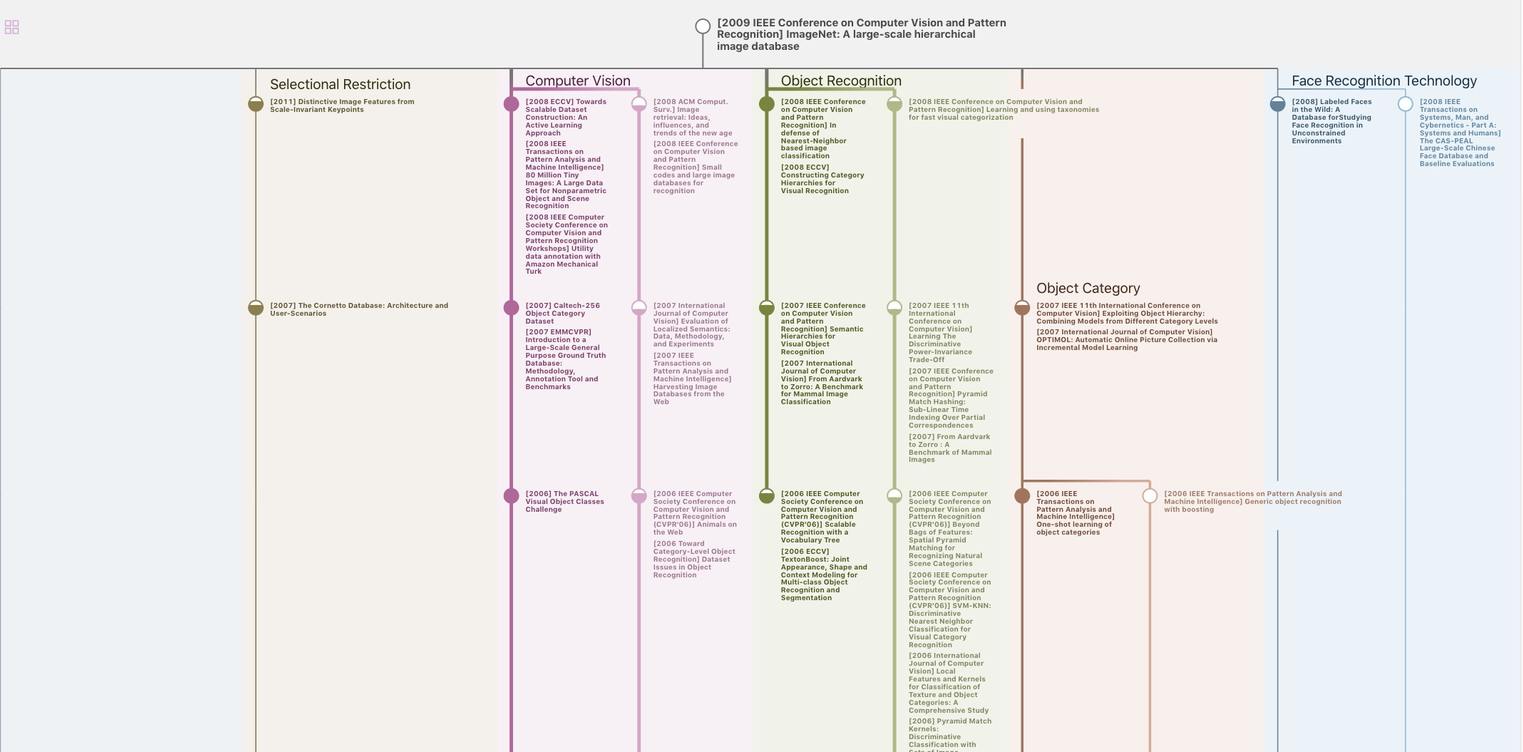
生成溯源树,研究论文发展脉络
Chat Paper
正在生成论文摘要