Improving Interpretability via Explicit Word Interaction Graph Layer
arxiv(2023)
摘要
Recent NLP literature has seen growing interest in improving model interpretability. Along this direction, we propose a trainable neural network layer that learns a global interaction graph between words and then selects more informative words using the learned word interactions. Our layer, we call WIGRAPH, can plug into any neural network-based NLP text classifiers right after its word embedding layer. Across multiple SOTA NLP models and various NLP datasets, we demonstrate that adding the WIGRAPH layer substantially improves NLP models' interpretability and enhances models' prediction performance at the same time.
更多查看译文
关键词
interpretability,interaction,word
AI 理解论文
溯源树
样例
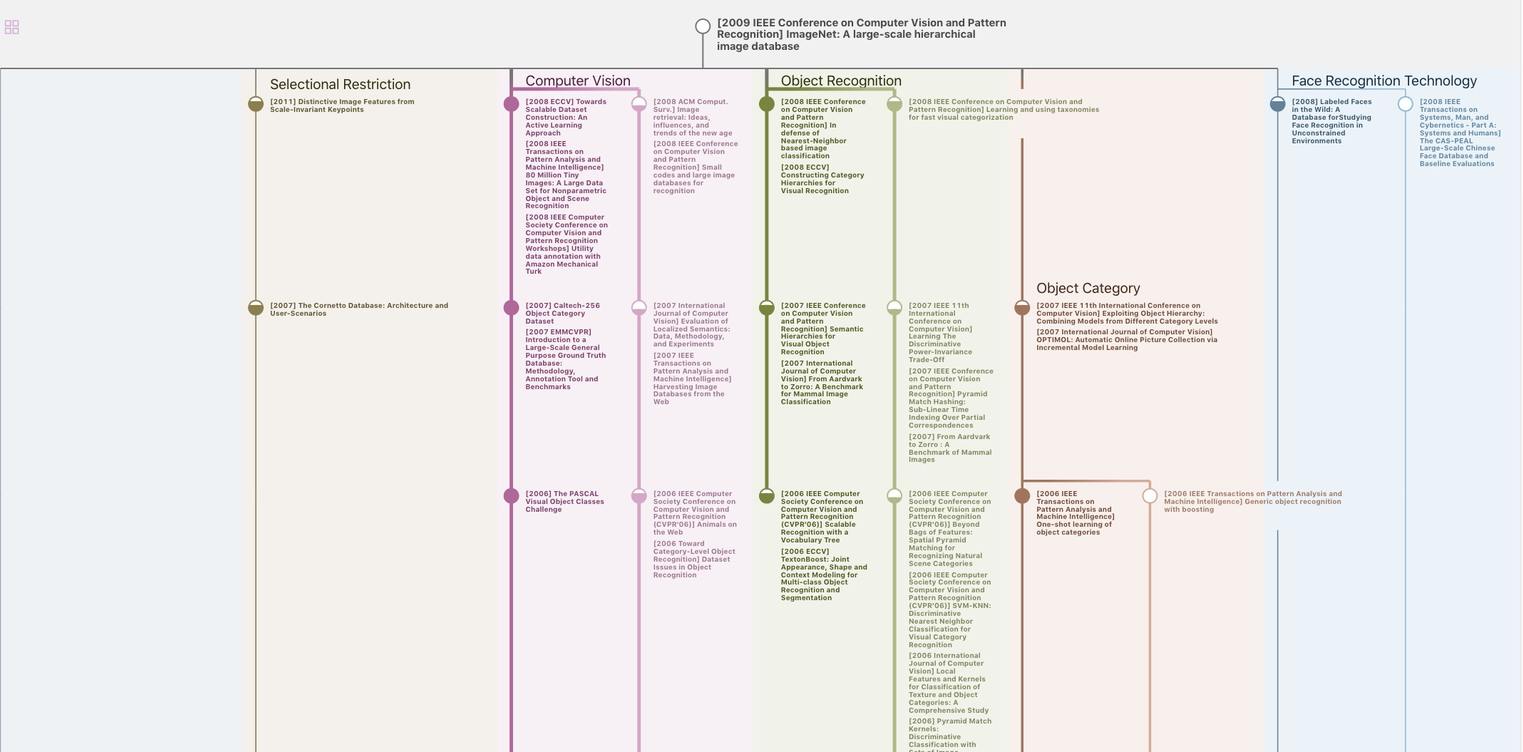
生成溯源树,研究论文发展脉络
Chat Paper
正在生成论文摘要