Temporal Coherent Test-Time Optimization for Robust Video Classification
ICLR 2023(2023)
摘要
Deep neural networks are likely to fail when the test data is corrupted in real-world deployment (e.g., blur, weather, etc.). Test-time optimization is an effective way that adapts models to generalize to corrupted data during testing, which has been shown in the image domain. However, the techniques for improving video classification corruption robustness remain few. In this work, we propose a Temporal Coherent Test-time Optimization framework (TeCo) to utilize spatio-temporal information in test-time optimization for robust video classification. To exploit information in video with self-supervised learning, TeCo uses global content from video clips and optimizes models for entropy minimization. TeCo minimizes the entropy of the prediction based on the global content from video clips. Meanwhile, it also feeds local content to regularize the temporal coherence at the feature level. TeCo retains the generalization ability of various video classification models and achieves significant improvements in corruption robustness across Mini Kinetics-C and Mini SSV2-C. Furthermore, TeCo sets a new baseline in video classification corruption robustness via test-time optimization.
更多查看译文
关键词
Video Classification,Robustness,Test Time Optimization
AI 理解论文
溯源树
样例
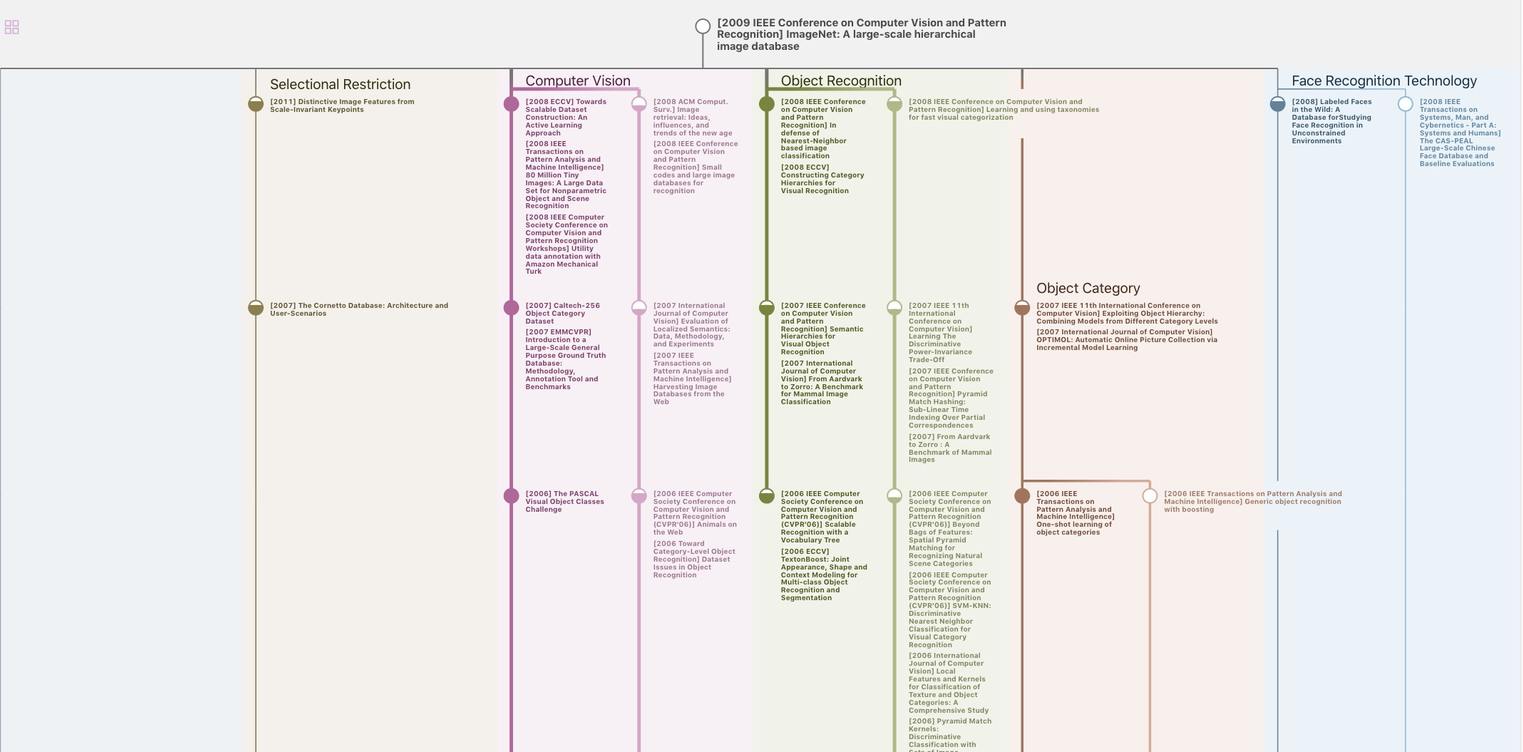
生成溯源树,研究论文发展脉络
Chat Paper
正在生成论文摘要