Defending against Adversarial Audio via Diffusion Model
ICLR 2023(2023)
摘要
Deep learning models have been widely used in commercial acoustic systems in recent years. However, adversarial audio examples can cause abnormal behaviors for those acoustic systems, while being hard for humans to perceive. Various methods, such as transformation-based defenses and adversarial training, have been proposed to protect acoustic systems from adversarial attacks, but they are less effective against adaptive attacks. Furthermore, directly applying the methods from the image domain can lead to suboptimal results because of the unique properties of audio data. In this paper, we propose an adversarial purification-based defense pipeline, AudioPure, for acoustic systems via off-the-shelf diffusion models. Taking advantage of the strong generation ability of diffusion models, AudioPure first adds a small amount of noise to the adversarial audio and then runs the reverse sampling step to purify the noisy audio and recover clean audio. AudioPure is a plug-and-play method that can be directly applied to any pretrained classifier without any fine-tuning or re-training. We conduct extensive experiments on the speech command recognition task to evaluate the robustness of AudioPure. Our method is effective against diverse adversarial attacks (e.g. L2 or L∞-norm). It outperforms the existing methods under both strong adaptive white-box and black-box attacks bounded by L2 or L∞-norm (up to +54% in robust accuracy). Besides, we also evaluate the certified robustness for perturbations bounded by L2-norm via randomized smoothing. Our pipeline achieves a higher certified accuracy than baselines.
更多查看译文
关键词
Adversarial attack and defense,AI security,speech recognition,diffusion models
AI 理解论文
溯源树
样例
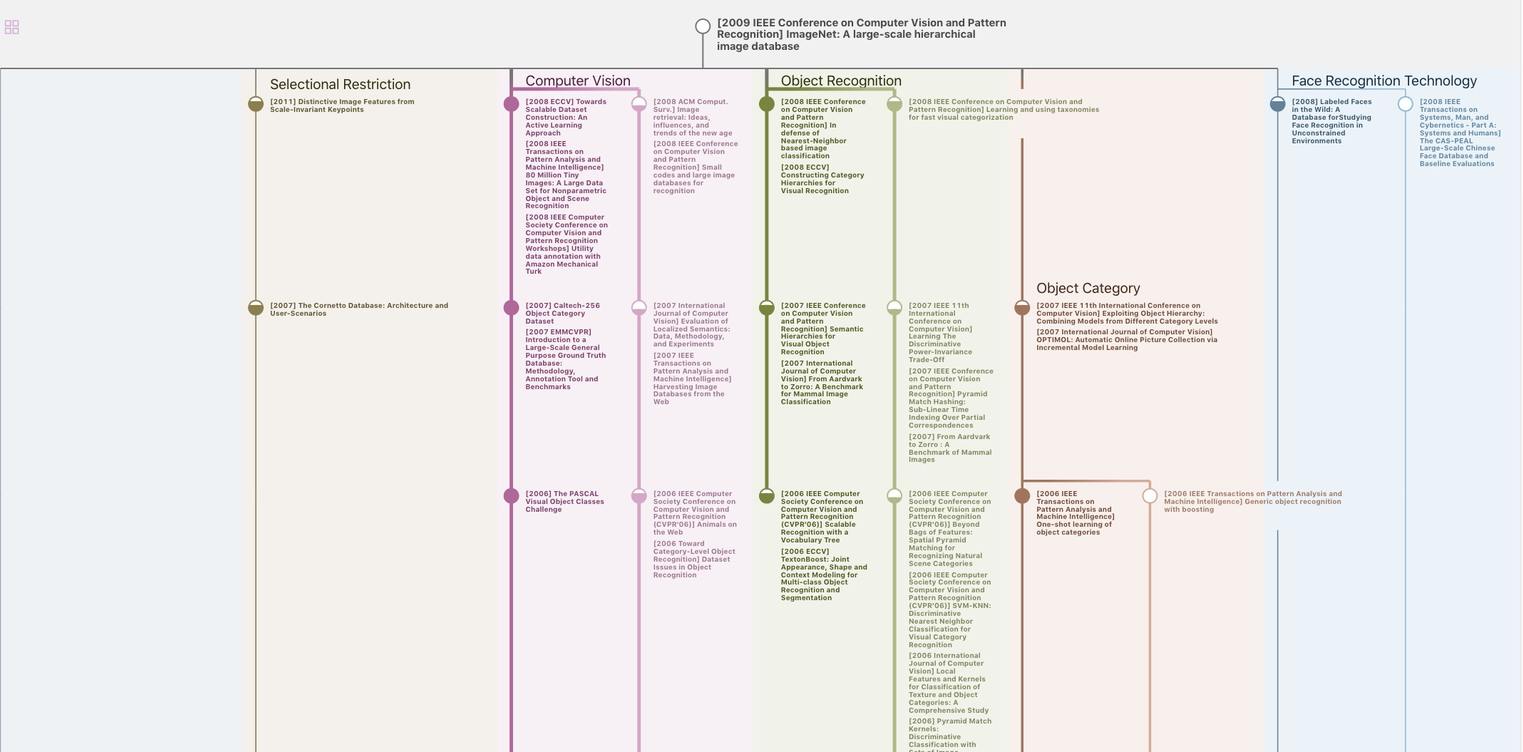
生成溯源树,研究论文发展脉络
Chat Paper
正在生成论文摘要