Structural Robust Label Propagation on Homogeneous Graphs
2022 IEEE International Conference on Data Mining (ICDM)(2022)
摘要
Label propagation and graph neural networks are two main methods for the semi-supervised node classification problem on graphs. They share similar idea of propagating information over the network, exhibiting promising performance on the node classification task. Despite effectiveness, the limitations of these propagation methods are still not well understood. From the perspective of label propagation then training, we observe three major challenges of these propagation methods. The observations from both theoretical analyses and empirical studies reveal that the propagation operations can degrade performance on certain labels and suffers from structure noise, which is described by edges with two nodes belonging to distinct labels. To address the above issues, we propose a new method termed Robust Label Propagation (RLP). RLP contains two novel strategies, Robust Training and Ada-Mixup. Robust Training can utilize more attribute information to have the overall training correction ability and alleviate the impact of structure noise significantly. Ada-Mixup can help RLP mine useful structure information by integrating information before and after the propagation adaptively. Extensive empirical studies on real-world datasets demonstrate that RLP outperforms the mainstream baselines on the node classification task in terms of effectiveness, efficiency and robustness.
更多查看译文
关键词
Label propagation,graph convolutional networks,structure noise,node classification
AI 理解论文
溯源树
样例
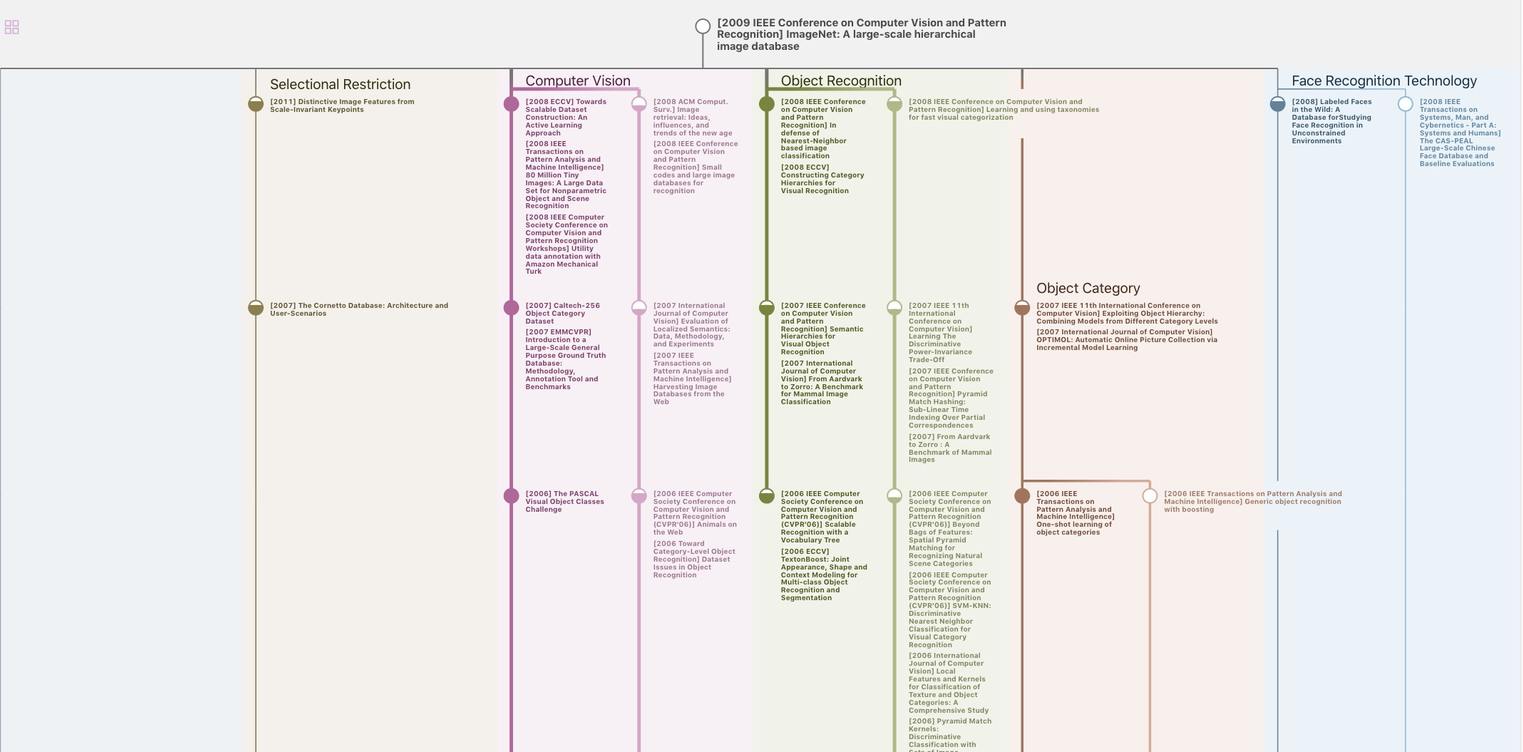
生成溯源树,研究论文发展脉络
Chat Paper
正在生成论文摘要