Graph Neural Network Based Surrogate Model of Physics Simulations for Geometry Design
arxiv(2023)
摘要
Computational Intelligence (CI) techniques have shown great potential as a surrogate model of expensive physics simulation, with demonstrated ability to make fast predictions, albeit at the expense of accuracy in some cases. For many scientific and engineering problems involving geometrical design, it is desirable for the surrogate models to precisely describe the change in geometry and predict the consequences. In that context, we develop graph neural networks (GNNs) as fast surrogate models for physics simulation, which allow us to directly train the models on 2/3D geometry designs that are represented by an unstructured mesh or point cloud, without the need for any explicit or hand-crafted parameterization. We utilize an encoder-processor-decoder-type architecture which can flexibly make prediction at both node level and graph level. The performance of our proposed GNN-based surrogate model is demonstrated on 2 example applications: feature designs in the domain of additive engineering and airfoil design in the domain of aerodynamics. The models show good accuracy in their predictions on a separate set of test geometries after training, with almost instant prediction speeds, as compared to O(hour) for the high-fidelity simulations required otherwise.
更多查看译文
关键词
physics simulations,neural network,geometry design
AI 理解论文
溯源树
样例
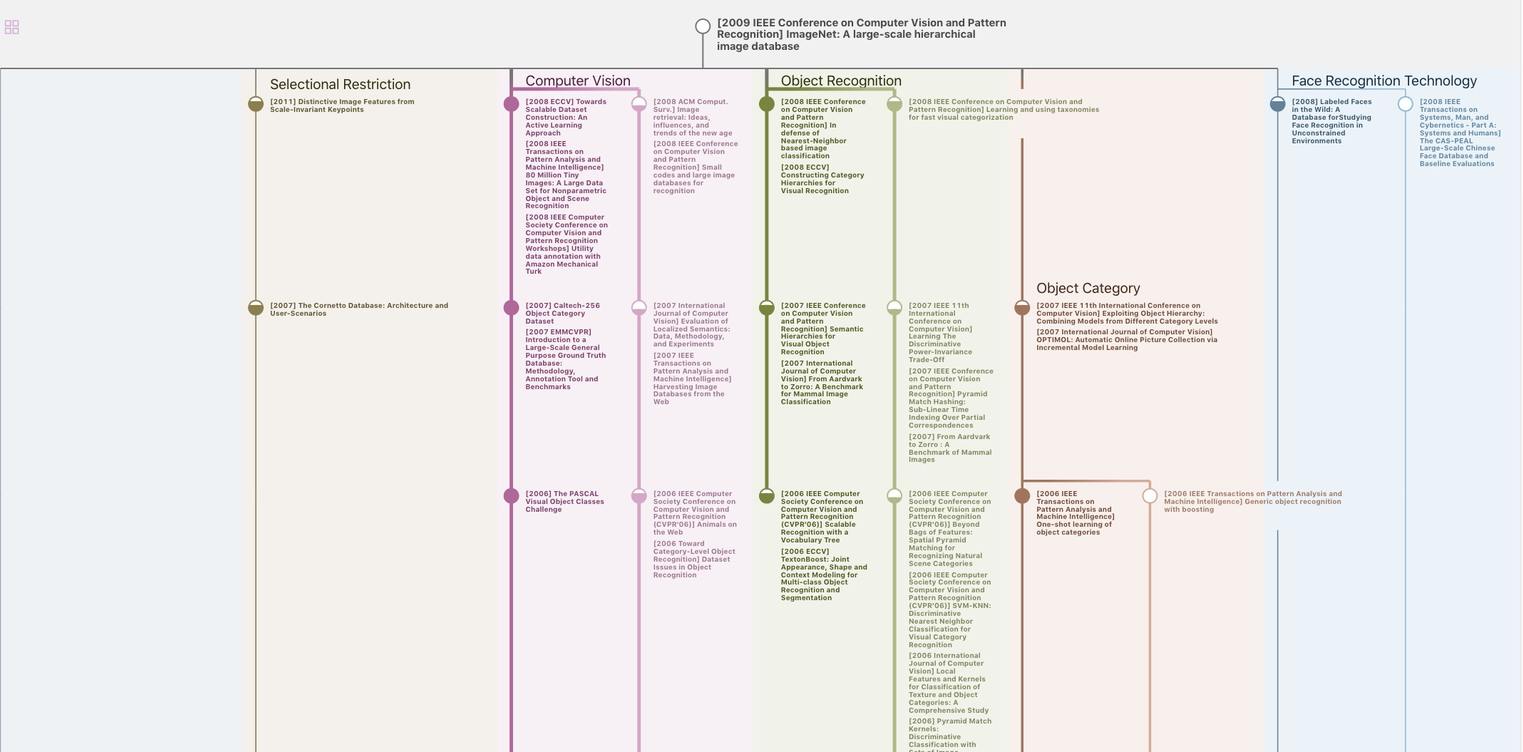
生成溯源树,研究论文发展脉络
Chat Paper
正在生成论文摘要