Data-driven Invariant Subspace Identification for Black-Box Switched Linear Systems
2022 IEEE 61ST CONFERENCE ON DECISION AND CONTROL (CDC)(2022)
摘要
We present an algorithmic framework for the identification of candidate invariant subspaces for switched linear systems. Namely, the framework allows to compute an orthonormal basis in which the matrices of the system are close to block-triangular matrices, based on a finite set of observed one-step trajectories and with a priori confidence level. The link between the existence of an invariant subspace and a common block-triangularization of the system matrices is well known. Under some assumptions on the system, one can also infer the existence of an invariant subspace when the matrices are close to be block-triangular. Our approach relies on quadratic Lyapunov analysis and recent tools in scenario optimization. We present two applications of our results for problems of consensus and opinion dynamics; the first one allows to identify the disconnected components in a switching hidden network, while the second one identifies the stationary opinion vector of a switching gossip process with antagonistic interactions.
更多查看译文
关键词
Dynamical Systems
AI 理解论文
溯源树
样例
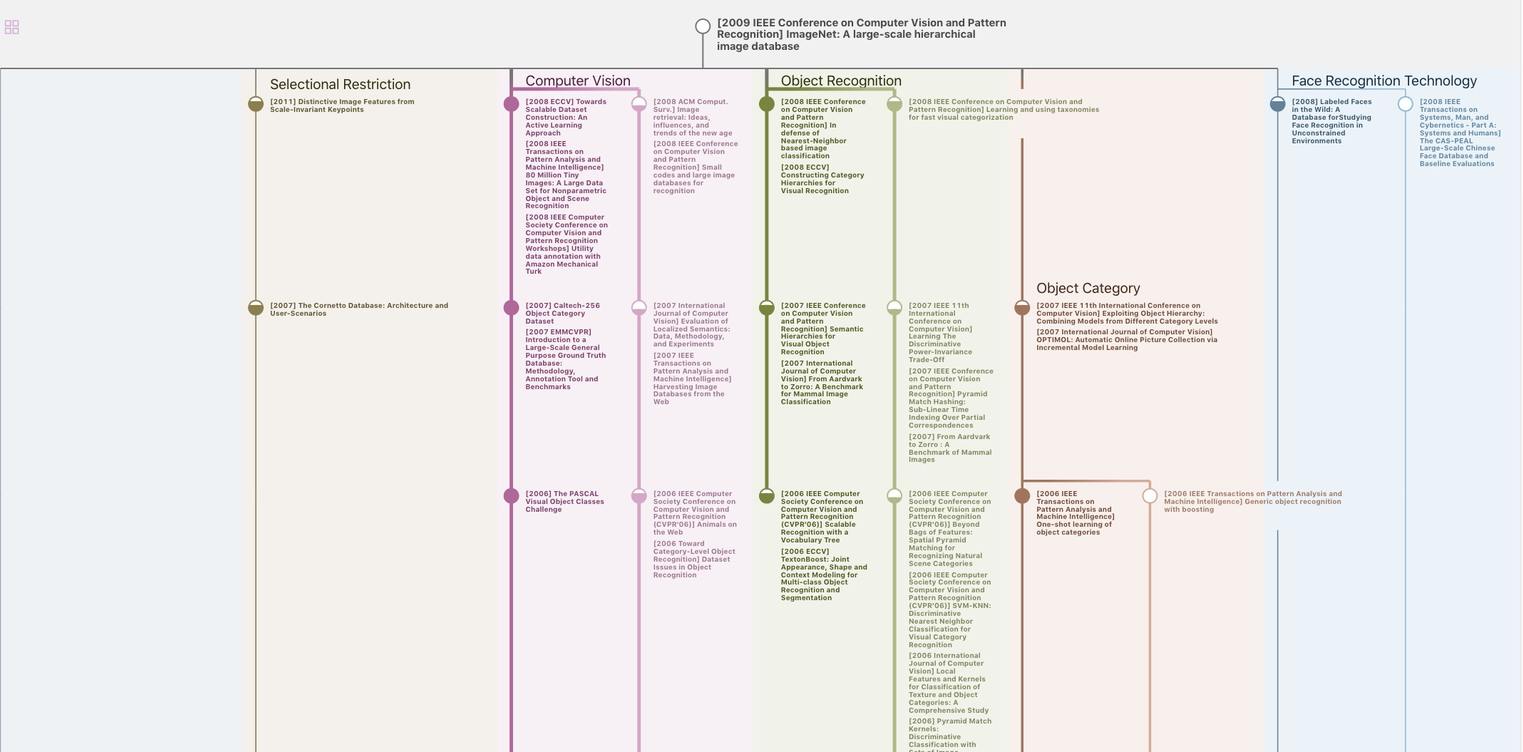
生成溯源树,研究论文发展脉络
Chat Paper
正在生成论文摘要