Knowledge Augmented Machine Learning with Applications in Autonomous Driving: A Survey.
CoRR(2022)
摘要
The availability of representative datasets is an essential prerequisite for many successful artificial intelligence and machine learning models. However, in real life applications these models often encounter scenarios that are inadequately represented in the data used for training. There are various reasons for the absence of sufficient data, ranging from time and cost constraints to ethical considerations. As a consequence, the reliable usage of these models, especially in safety-critical applications, is still a tremendous challenge. Leveraging additional, already existing sources of knowledge is key to overcome the limitations of purely data-driven approaches. Knowledge augmented machine learning approaches offer the possibility of compensating for deficiencies, errors, or ambiguities in the data, thus increasing the generalization capability of the applied models. Even more, predictions that conform with knowledge are crucial for making trustworthy and safe decisions even in underrepresented scenarios. This work provides an overview of existing techniques and methods in the literature that combine data-driven models with existing knowledge. The identified approaches are structured according to the categories knowledge integration, extraction and conformity. In particular, we address the application of the presented methods in the field of autonomous driving.
更多查看译文
关键词
knowledge,autonomous,driving,machine learning
AI 理解论文
溯源树
样例
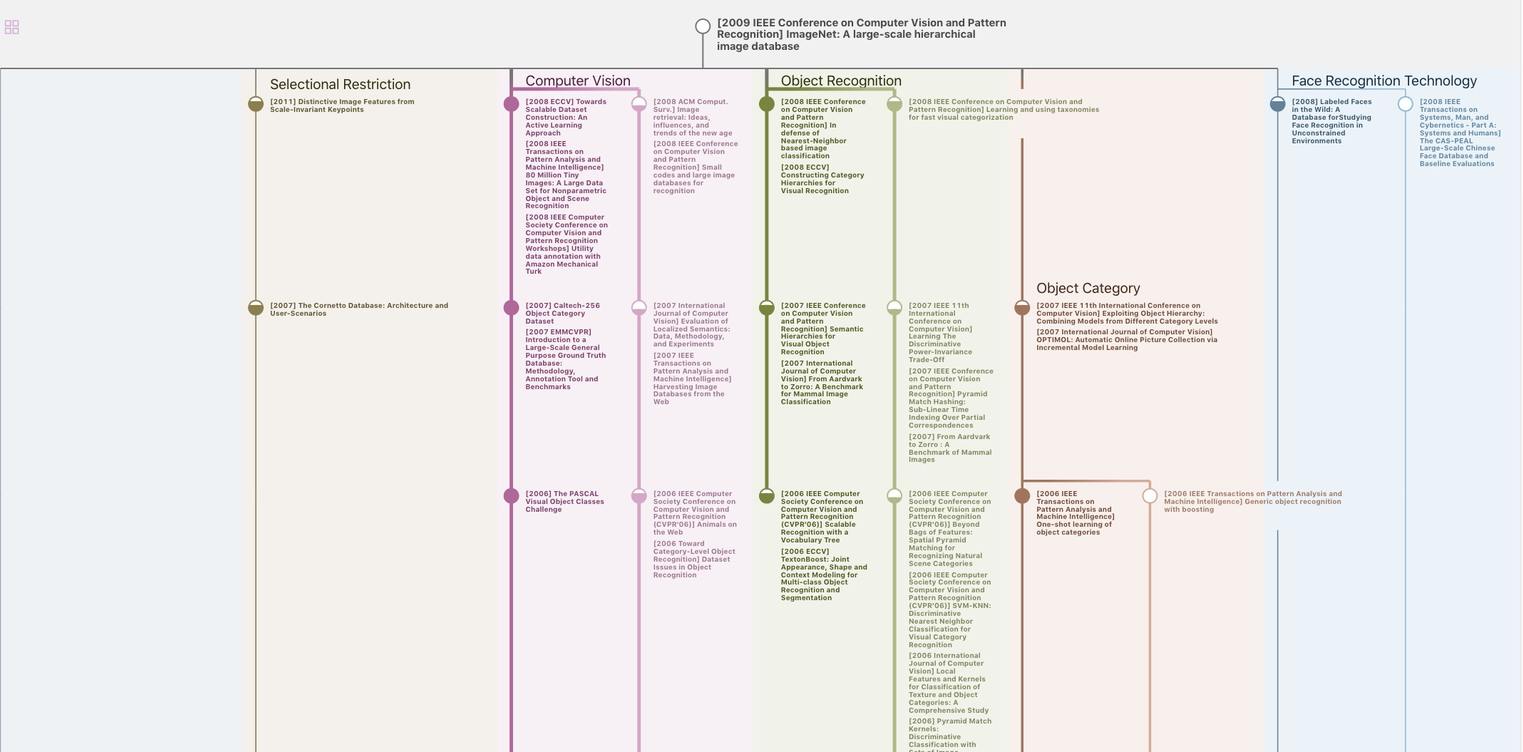
生成溯源树,研究论文发展脉络
Chat Paper
正在生成论文摘要