Time Series Prediction Via Elastic Net Regularization Integrating Partial Autocorrelation
Applied soft computing(2022)
摘要
In this paper, we propose a new elastic net regularization integrating partial autocorrelation coefficients (AEN-PAC) model for time series prediction. This model solves the inaccuracy of variable selection and parameter estimation caused by ignoring the dependence of time series in the adaptive elastic net. The proposed AEN-PAC model adds the partial autocorrelation coefficient to the penalty term of the adaptive elastic net, so that the influence of time on the data series can be well explained. Further, we prove a theorem to demonstrate that our method encourages grouping effects. Then, we convert the optimization problem of the proposed AEN-PAC model into an adaptive lasso model and propose an effective algorithm to solve it. Finally, we conduct a simulation study and empirical analysis on two time series sets. Simulation study shows that the proposed AEN-PAC model selects variable more correctly, compared with other models including Adaptive Elastic Net(AEN), Adaptive Lasso(AL), Elastic Net(EN), and Lasso. In addition, from the perspective of parameter estimation, the parameters estimated by our new model are closer to the real model. For Alibaba stock data and Nike stock data, the prediction errors are 7.07172 and 3.94916 respectively, which are smaller than other models. The results indicating that the proposed AEN-PAC model performs better in time series prediction.
更多查看译文
关键词
Elastic net,Variable selection,Partial autocorrelation coefficient,Time series prediction
AI 理解论文
溯源树
样例
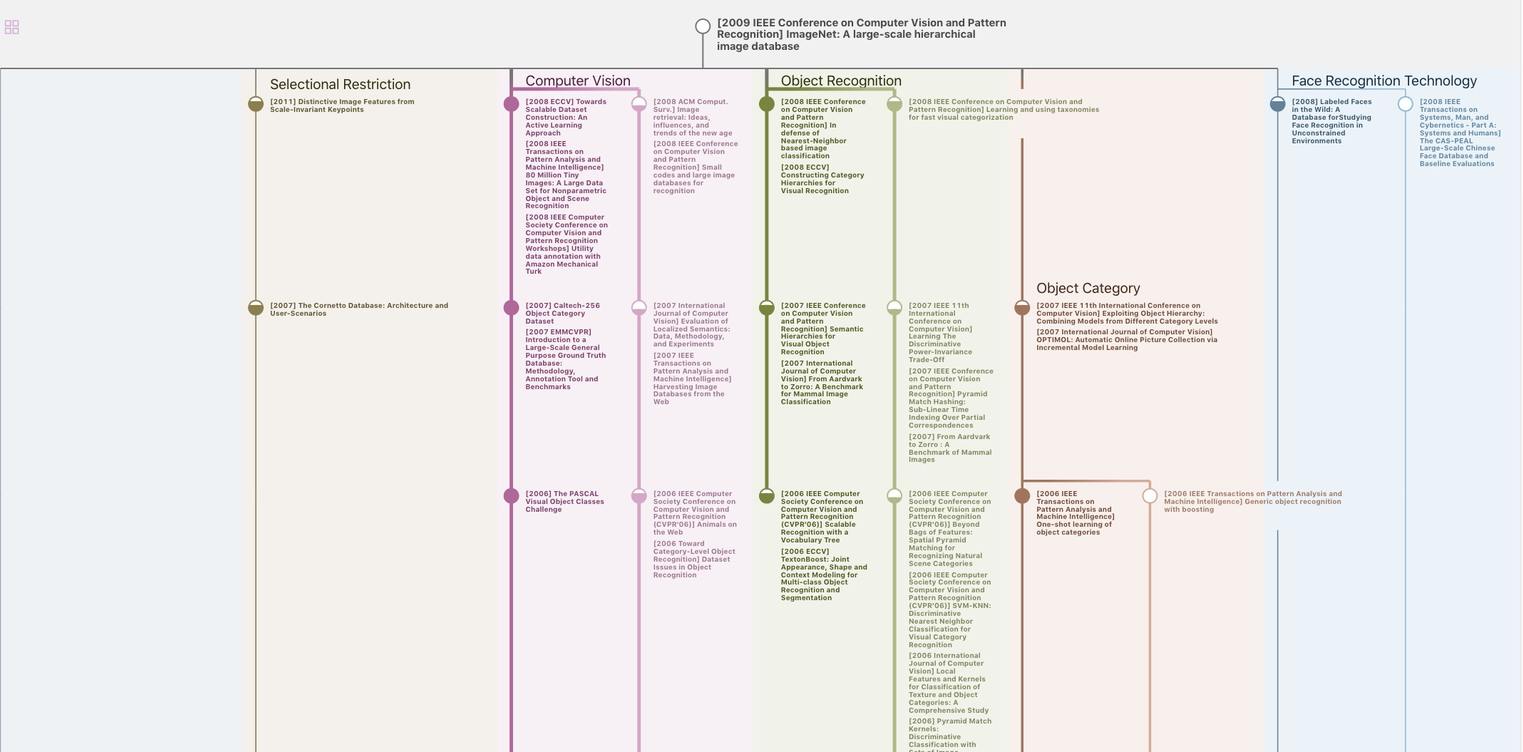
生成溯源树,研究论文发展脉络
Chat Paper
正在生成论文摘要