Significance of Organic Ferroelectric in Harnessing Transient Negative Capacitance Effect at Low Voltage over Oxide Ferroelectric
2022 IEEE International Symposium on Circuits and Systems (ISCAS)(2022)
Indian Inst Technol Roorkee
Abstract
The concept of leveraging the transient negative capacitance (TNC) effect in a ferroelectric (FE) is a relatively new addition to the field of nanoelectronics. Until now, there has been no comparison of organic and oxide FE-based metal-FE-metal (MFM) devices in harnessing the TNC effect. As a result, we introduce an external resistor-MFM(R-MFM) series circuit to investigate the role of organic and oxide FEs in harnessing the TNC effect at low supply voltages. The multidomain Ginzburg-Landau-Khalatnikov theory is used to model the FE materials in a technology computer-aided design environment. We show that: (i) organic FE-based R-MFM series circuit can harness the TNC effect at just 1 V whereas an oxide FE-based R-MFM series circuit cannot; (ii) the coercivity of an organic FE is 77.39% lower than its counterpart, oxide FE; (iii) the remanent polarization of an organic MFM (1.2$\mu$C/cm 2 ) is very close to the channel charge density of a CMOS transistor (1.6$\mu$C/cm 2 ) making it helpful in addressing capacitance matching issues in NC transistor; (iv) an organic FE-based R-MFM series circuit dissipates 78.89% less energy than an oxide FE-based R-MFM series circuit; (v) the TNC effect and time are justified by its dependence on R. Finally, this article suggests that an organic FE-based MFM could be used as a gate stack of any transistor to achieve sub-60 mV/decade switching energy, making it ideal for ultra-low voltage NC transistors.
MoreTranslated text
Key words
Ferroelectric (FE),hafnium oxide,multidomain,negative capacitance (NC),organic ferroelectric,TCAD
求助PDF
上传PDF
View via Publisher
AI Read Science
AI Summary
AI Summary is the key point extracted automatically understanding the full text of the paper, including the background, methods, results, conclusions, icons and other key content, so that you can get the outline of the paper at a glance.
Example
Background
Key content
Introduction
Methods
Results
Related work
Fund
Key content
- Pretraining has recently greatly promoted the development of natural language processing (NLP)
- We show that M6 outperforms the baselines in multimodal downstream tasks, and the large M6 with 10 parameters can reach a better performance
- We propose a method called M6 that is able to process information of multiple modalities and perform both single-modal and cross-modal understanding and generation
- The model is scaled to large model with 10 billion parameters with sophisticated deployment, and the 10 -parameter M6-large is the largest pretrained model in Chinese
- Experimental results show that our proposed M6 outperforms the baseline in a number of downstream tasks concerning both single modality and multiple modalities We will continue the pretraining of extremely large models by increasing data to explore the limit of its performance
Upload PDF to Generate Summary
Must-Reading Tree
Example
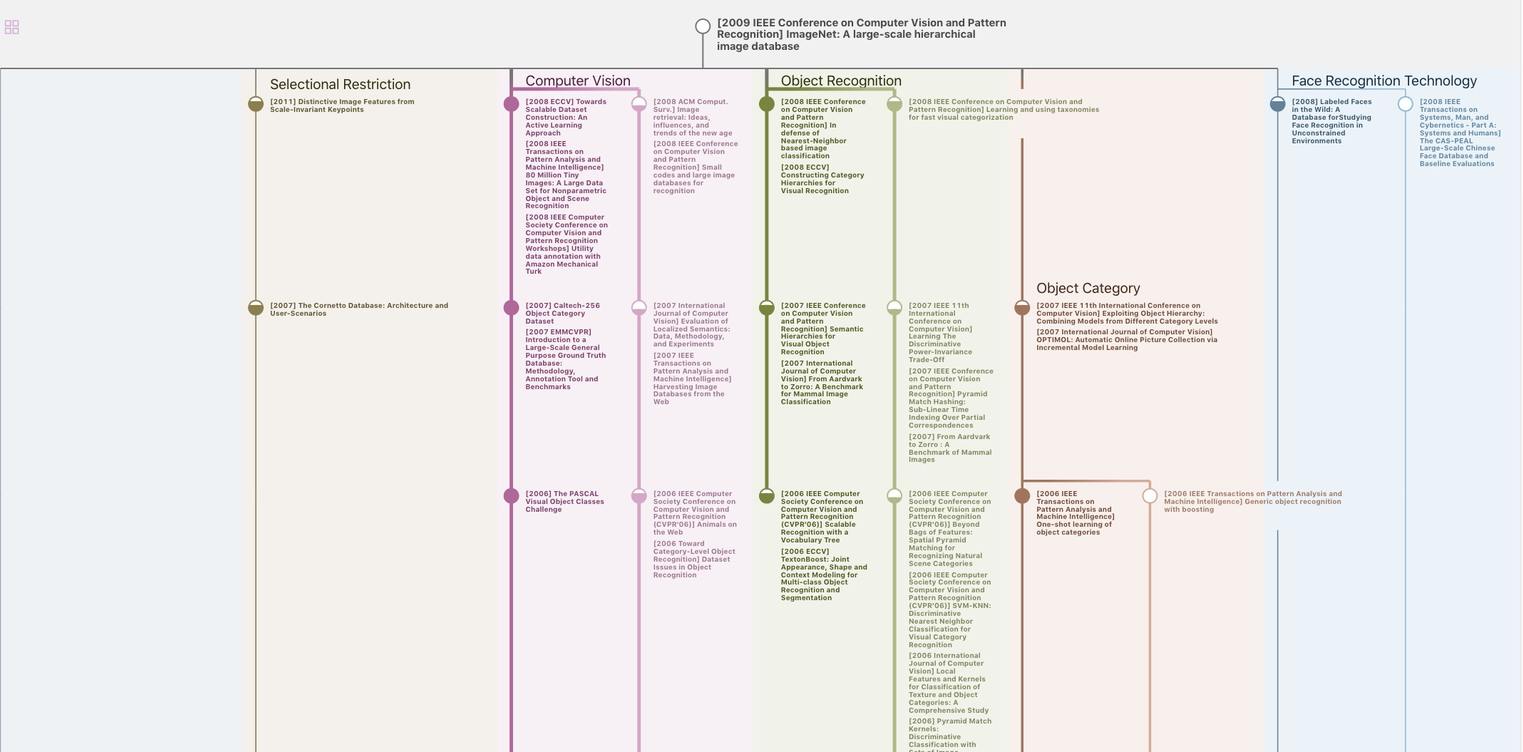
Generate MRT to find the research sequence of this paper
Related Papers
2003
被引用54 | 浏览
2008
被引用1819 | 浏览
2011
被引用283 | 浏览
2020
被引用13 | 浏览
2021
被引用6 | 浏览
2021
被引用3 | 浏览
Data Disclaimer
The page data are from open Internet sources, cooperative publishers and automatic analysis results through AI technology. We do not make any commitments and guarantees for the validity, accuracy, correctness, reliability, completeness and timeliness of the page data. If you have any questions, please contact us by email: report@aminer.cn
Chat Paper
GPU is busy, summary generation fails
Rerequest