Learning Mask Scalars for Improved Robust Automatic Speech Recognition
2022 IEEE Spoken Language Technology Workshop (SLT)(2023)
摘要
Improving robustness of streaming automatic speech recognition (ASR) systems using neural network based acoustic frontends is challenging because of causality constraints and the speech-distortions introduced by the frontend. Time-frequency masking based approaches are commonly used, but they need additional hyperparameters – mask scalars – to limit distortion. Mask scalars are typically hand-tuned and chosen conservatively. In this work, we present a technique to predict mask scalars using ASR loss in an end-to-end fashion, with minimal increase in model size and complexity. We evaluate the approach on two robust ASR tasks: multichannel enhancement in the presence of speech and non-speech noise, and acoustic echo cancellation (AEC). Results show that the presented algorithm consistently improves word error rate (WER) over strong baselines that use hand-tuned hyperparameters: up to 16% in noisy conditions, and up to 7% for AEC.
更多查看译文
关键词
speech recognition,time-frequency masking,speech enhancement,acoustic echo cancellation
AI 理解论文
溯源树
样例
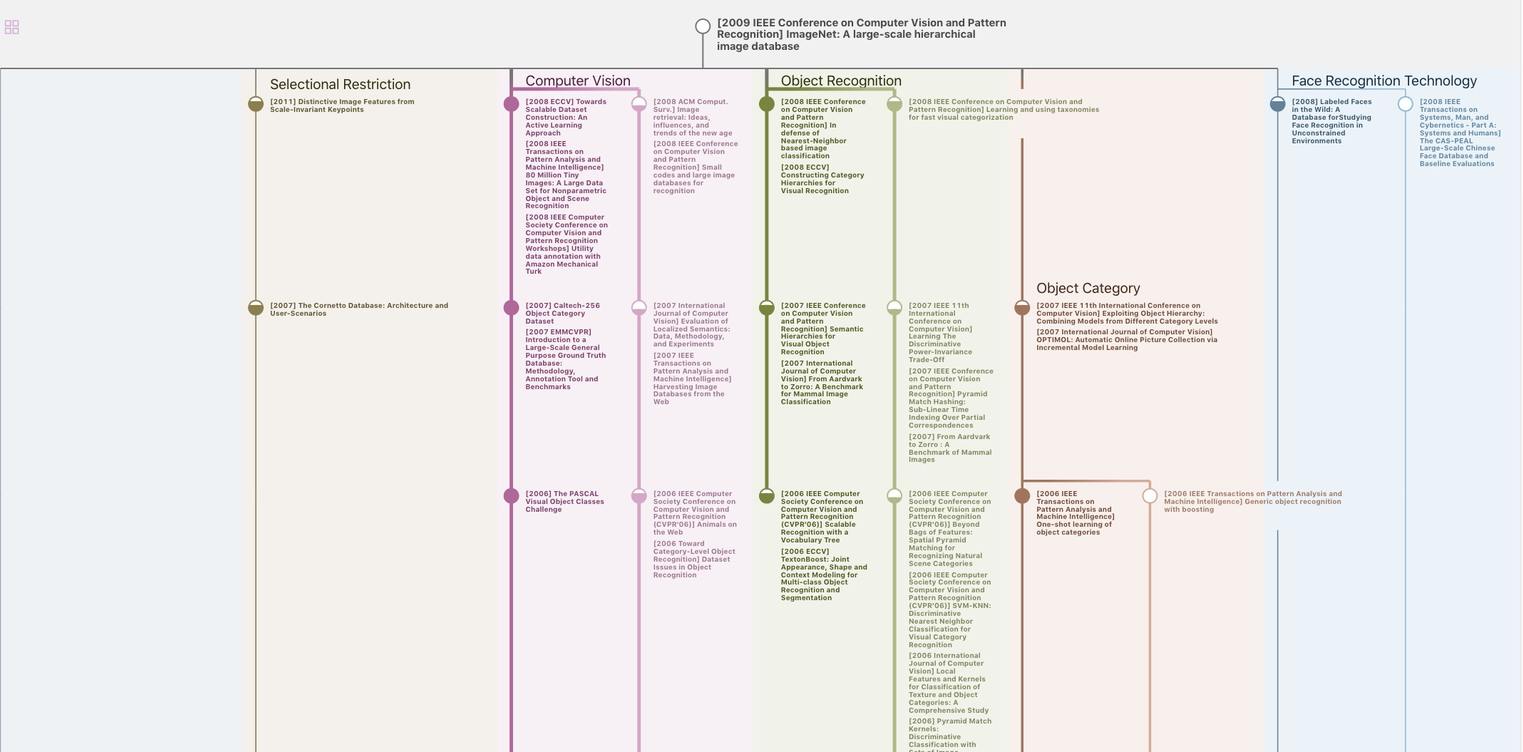
生成溯源树,研究论文发展脉络
Chat Paper
正在生成论文摘要