An Interpretable Machine Learning Approach to Estimate the Influence of Inflammation Biomarkers on Cardiovascular Risk Assessment
COMPUTER METHODS AND PROGRAMS IN BIOMEDICINE(2023)
摘要
Background and objective: Cardiovascular disease has a huge impact on health care services, originating unsustainable costs at clinical, social, and economic levels. In this context, patients' risk stratification tools are central to support clinical decisions contributing to the implementation of effective preventive health care. Although useful, these tools present some limitations, in particular, some lack of performance as well as the impossibility to consider new risk factors potentially important in the prognosis of severe car-diac events. Moreover, the actual use of these tools in the daily practice requires the physicians' trust. The main goal of this work addresses these two issues: (i) evaluate the importance of inflammation biomark-ers when combined with a risk assessment tool; (ii) incorporation of personalization and interpretability as key elements of that assessment.Methods: Firstly, machine learning based models were created to assess the potential of the inflam-mation biomarkers applied in secondary prevention, namely in the prediction of the six month risk of death/myocardial infarction. Then, an approach based on three main phases was created: (i) set of inter-pretable rules supported by clinical evidence; (ii) selection based on a machine learning classifier able to identify for a given patient the most suitable subset of rules; (iii) an ensemble scheme combining the previous subset of rules in the estimation of the patient cardiovascular risk. All the results were statis-tically validated ( t-test, Wilcoxon-signed rank test) according to a previous verification of data normality (Shapiro-Wilk).Results: The proposed methodology was applied to a real acute coronary syndrome patients dataset ( N = 1544) from the Cardiology Unit of Coimbra Hospital and Universitary centre. The first assessment was based on the GRACE tool and a Random Forest classifier, the incorporation of inflammation biomark-ers achieved SE = 0.83; SP = 0.84 whereas the original GRACE risk factors reached SE = 0.75; SP = 0.85. In the second phase, the proposed approach with inflammation biomarkers achieved SE = 0.763 and SP = 0.778. Conclusions: This approach confirms the potential of combining inflammation markers with the GRACE score, increasing SE and SP, when compared with the original GRACE. Additionally, it assures inter-pretability and personalization, which are critical issues to allow its application in the daily clinical prac-tice.(c) 2023 The Author(s). Published by Elsevier B.V. This is an open access article under the CC BY-NC-ND license ( http://creativecommons.org/licenses/by-nc-nd/4.0/ )
更多查看译文
关键词
Inflammation biomarkers,GRACE,Data Fusion,Interpretability,Personalization
AI 理解论文
溯源树
样例
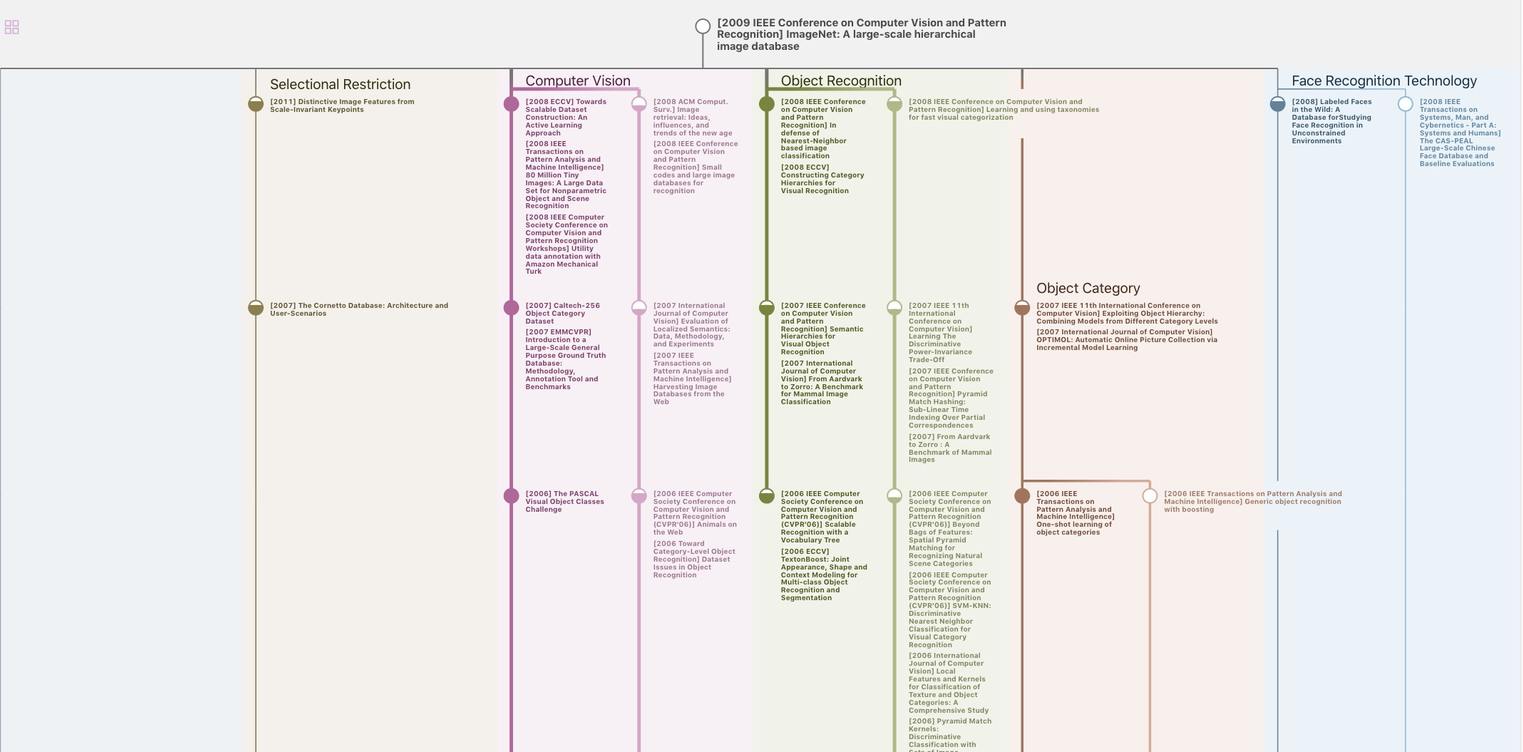
生成溯源树,研究论文发展脉络
Chat Paper
正在生成论文摘要