Social Determinants of Health and Selection Bias in Genome‐wide Association Studies
World Psychiatry(2023)
摘要
World PsychiatryVolume 22, Issue 1 p. 160-161 Letter to the EditorFree Access Social determinants of health and selection bias in genome-wide association studies Kira E. Riehm, Kira E. Riehm Department of Epidemiology, Mailman School of Public Health, Columbia University, New York, NY, USASearch for more papers by this authorKatherine M. Keyes, Katherine M. Keyes Department of Epidemiology, Mailman School of Public Health, Columbia University, New York, NY, USASearch for more papers by this authorEzra S. Susser, Ezra S. Susser Department of Epidemiology, Mailman School of Public Health, Columbia University, New York, NY, USA New York State Psychiatric Institute, New York, NY, USASearch for more papers by this author Kira E. Riehm, Kira E. Riehm Department of Epidemiology, Mailman School of Public Health, Columbia University, New York, NY, USASearch for more papers by this authorKatherine M. Keyes, Katherine M. Keyes Department of Epidemiology, Mailman School of Public Health, Columbia University, New York, NY, USASearch for more papers by this authorEzra S. Susser, Ezra S. Susser Department of Epidemiology, Mailman School of Public Health, Columbia University, New York, NY, USA New York State Psychiatric Institute, New York, NY, USASearch for more papers by this author First published: 14 January 2023 https://doi.org/10.1002/wps.21047 K.E. Riehm is supported by the National Institute of Mental Health (NIMH) Psychiatric Epidemiology Training Program (5T32MH013043-50) at Columbia University. AboutSectionsPDF ToolsRequest permissionExport citationAdd to favoritesTrack citation ShareShare Give accessShare full text accessShare full-text accessPlease review our Terms and Conditions of Use and check box below to share full-text version of article.I have read and accept the Wiley Online Library Terms and Conditions of UseShareable LinkUse the link below to share a full-text version of this article with your friends and colleagues. Learn more.Copy URL The rapid pace of research continues to shed light on the complex genetic architecture that contributes to psychiatric disorder risk. The pace has been accelerated in part by the collection and analysis of increasingly larger samples of participants for genome-wide association studies (GWAS). Large-scale GWAS typically report very small, statistically significant associations with numerous common variants, and increasingly produce polygenic risk scores (PRS) by combining associations of these variants with disorders in weighted or otherwise transformed summation scores. However, there are still fundamental limitations in how GWAS findings in psychiatry are generated and interpreted. Two central limitations to scientific progress are the insufficient consideration of the social determinants of health and the selection bias in GWAS samples. These issues are also relevant to associations discovered in next generation fine mapping. Our views in this respect largely derive from psychiatric epidemiology, a field in which these issues have been prominent over a long period. The goals of GWAS include identifying novel risk loci, quantifying genetic risk, and improving our understanding of the underlying pathophysiological mechanisms of mental disorders. The results tend to be presented in ways that are forward-looking, with claims that findings from GWAS could eventually be translated into useful applications. Implications for individualized risk prediction or “precision medicine” in clinical settings are often highlighted. The success of GWAS in identifying large numbers of genetic variants that could be markers for risk loci depends heavily on the size of discovery samples, exemplified by the strong correlation between discovery sample size and number of statistically significant associations with genetic variants that are identified1. Indeed, it has been shown for some traits that the increasing rate of identification of statistically significant genetic loci does not appear to plateau, even as sample sizes surpass a million observations1. Given that some unknown proportion of these loci may signal a valid association, the study design of many GWAS has placed a strong emphasis on accruing larger and larger samples, with some authors calling – somewhat controversially – for prioritization of increasing sample sizes over other important features of study design2. There is no doubt that aggregating larger samples could be helpful in achieving the goals of GWAS. At the same time, however, an undue focus on sample size as the overriding priority in study design could undermine progress. We propose that more attention to the two fundamental features we discuss here – social determinants of health and selection bias – is essential to advance the potential of GWAS for understanding pathophysiology, and ultimately for contributing to clinical care and population mental health. The prevalence of social factors within and across samples directly affects validity of GWAS findings with respect to mental disorders. The value of GWAS lies in their potential to identify genetic alleles that may influence a given phenotypic outcome. In the search for such alleles, however, GWAS rarely consider how the patterning of social determinants of health in their sample may influence observed results. The sample composition with respect to social determinants will have a major impact on the magnitude of the effects detected for individual genetic loci, as well as for PRS. The impact of sample composition on effect size follows directly from the basic logic of epidemiology, and the potential to influence results has been evident in empirical studies – for example, in one study that considered characteristics such as acculturation and age at immigration, and their relationships with outcomes such as body mass index and blood pressure3. Emerging study designs, such as genome-wide environment interaction studies (GWEIS), combined with movements towards large-scale measurement of social determinants of health via electronic health records and linkage to biobanks, represent potentially important steps toward ameliorating bias as well as detecting environmental influences on disorders4. An ill-considered implementation of GWEIS, however, may have the unintended consequence of reducing the social environment to an uninformative measure. For example, decades of psychiatric epidemiological research make clear that basic checklists, or worse, single questions about “stressful life events” are of limited (if not zero) utility for characterizing the social environment. Moreover, GWAS rarely consider in any depth the theories that could explain the social patterning of mental health in their samples. Taking a social relational approach to studying the environment is critical. Social determinants of health include not only individual characteristics (e.g., income, highest completed education) but also structural determinants and social arrangements (e.g., class location). Recent studies suggest that important relationships between genetic risk and social context may be present even at the neighborhood level (e.g., collective efficacy)5, and the influence of sociocultural context is likely to be greater at higher levels (e.g., racialized minority vs. dominant majority; nations with plentiful vs. scarce resources). Consequently, GWEIS limited to the narrow range captured by standard biological measures of the “exposome” will also be rather uninformative in this regard. We hope that current efforts to include social concepts and their measures will prove successful6. Altogether, grounding our study designs in evidence-based social theories will accelerate progress toward meaningful gene-by-environment investigations. With regards to selection bias, increasing sample size allows valid signals of causation to emerge only when models are not misspecified. Otherwise, the meaning of the signals obtained in GWAS, and what these associations fundamentally represent – whether a true association, false positive, interaction with other genes, or something else – remains unclear. Selection bias occurs when individuals in a study population differ systematically from, and are not representative of, the target population (the population that you want to make inferences about). When selection bias is present, large sample sizes will amplify biased results, which is often the case in studies using “well” or “normal” controls as well as those with minimal phenotyping7. The importance of remaining attuned to this aspect of study design therefore increases – not decreases – as sample size increases. In other words, while increases in sample size reduce random error, they amplify systematic error. For example, it is now generally accepted that GWAS findings are not directly transportable between populations of different ancestry. This is not only because of allelic variation across populations, but also because socially constructed categories such as “race” may intersect and interact with genes or with other causal factors in the environment8. As a result, researchers must be highly attentive to the causal architecture that underlies the PRS or other genetic measurements. At a minimum, specifying target populations and reporting what is known about potential selection bias from those populations, as well as specifying hypotheses about effect measure modifiers of PRSs, is essential for valid inferences based on GWAS results9. In sum, the fundamental goal of psychiatric epidemiology is disease prevention. Genetic psychiatry has immense potential to contribute to this cause, but must match the enthusiasm for large sample sizes with an equal consideration for study design and interpretation. A paradigm shift – away from an overriding focus on sample size, and toward comprehensive assessments of social determinants of health in genetic discovery samples – will better advance our understanding of the genetic architecture of mental disorders and of its implications for prevention. References 1Visscher PM, Wray NR, Zhang Q et al. Am J Hum Genet 2017; 101: 5- 22. 2Mitchell BL, Thorp JG, Wu Y et al. JAMA Psychiatry 2021; 78: 1152- 60. 3Hollister BM, Farber-Eger E, Aldrich MC et al. Front Genet 2019; 10: 428. 4Aschard H, Lutz S, Maus B et al. Hum Genet 2012; 131: 1591- 613. 5McDaniel T, Wilson DK, Coulon MS et al. Ann Behav Med 2020; 55: 708- 19. 6Robinson O, Tamayo I, De Castro M et al. Environ Health Perspect 2018; 126:077005. 7Schwartz S, Susser E. Psychol Med 2011; 41: 1127- 31. 8Borrell LN, Elhawary JR, Fuentes-Afflick E et al. N Engl J Med 2021; 384: 474- 80. 9Westreich D, Edwards JK, Lesko CR et al. Am J Epidemiol 2019; 188: 438- 43. Volume22, Issue1February 2023Pages 160-161 ReferencesRelatedInformation
更多查看译文
关键词
Polygenic Risk Scores,Genome-Wide Association,Human Genomics
AI 理解论文
溯源树
样例
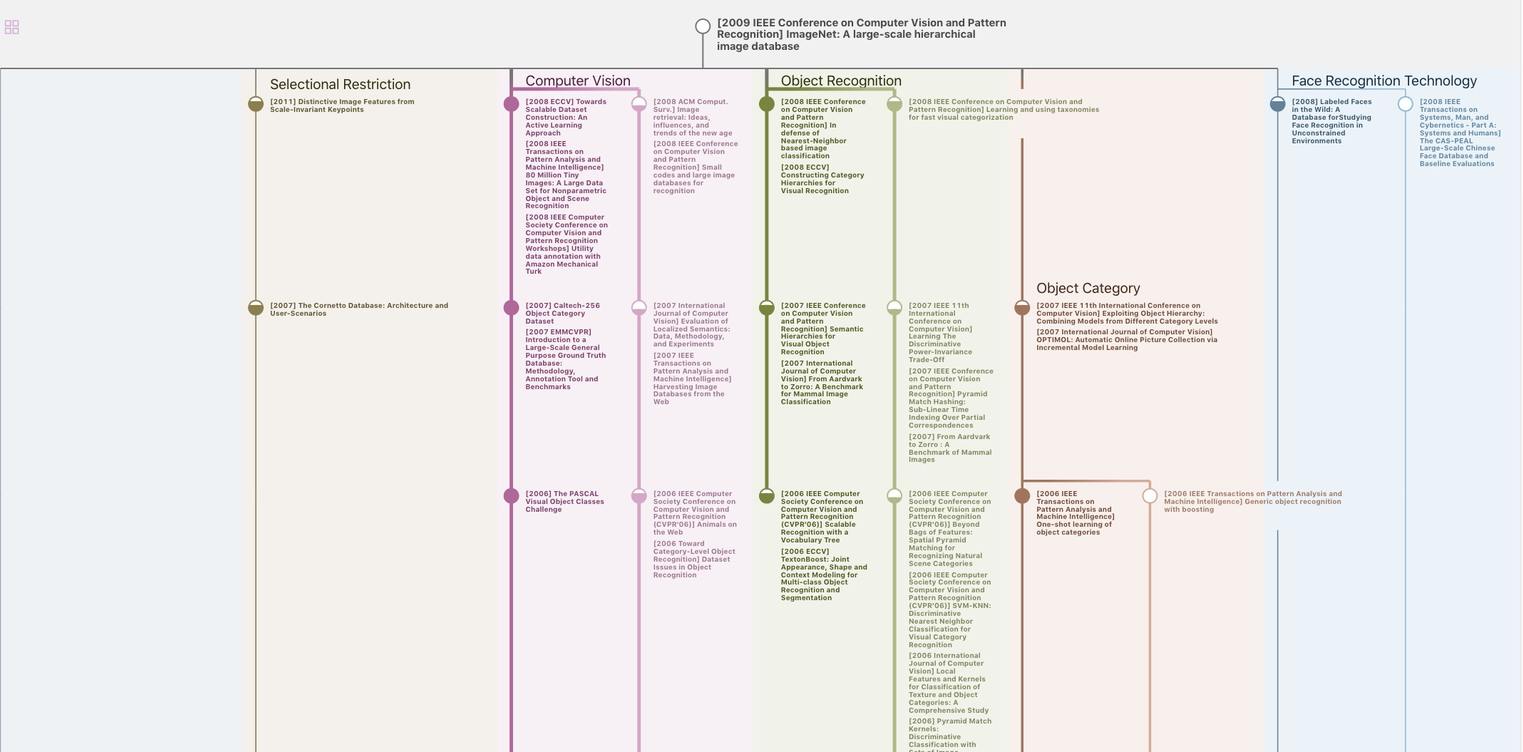
生成溯源树,研究论文发展脉络
Chat Paper
正在生成论文摘要