Ensemble Learning Methods of Adversarial Attacks and Defenses in Computer Vision: Recent Progress
2021 International Conference on Advanced Computing and Endogenous Security(2022)
摘要
Artificial intelligence (AI) has developed rapidly in recent decades and is widely used in many fields, such as natural language processing, voice recognition, and especially computer vision (CV). However, the endogenous security problems brought by the AI model itself, leading to the emergence of adversarial examples (AEs), which can fool the AI models and cause a serious impact on the classification. In recent years, researches show that ensemble learning methods are effective both in generating or detecting AEs. By integrating to generate AEs, the attackers can implement stronger and good transferability attacks to the target models. On the other hand, ensemble learning methods can also be used in defenses that can improve the robustness against AEs. In this paper, we focus on the ensemble learning methods in the CV field, and first introduce the classic adversarial attack and defense technologies. Then, we survey the ensemble learning methods in the adversarial environment and divide them into three types of frameworks (i.e., parallel, sequential, and hybrid). To the best of our knowledge, we are the first to analyze the recent proposed attacks and defenses in the adversarial environment from the perspective of these ensemble frameworks. Additionally, we summarize the advantages and disadvantages of these ensemble methods and frameworks. In the end, we give some suggestions for using ensemble frameworks, and we put forward several opinions from the aspects of attacks, defenses, and evaluations for future research directions in this field.
更多查看译文
关键词
computer vision,adversarial example,adversarial attack,adversarial defense,ensemble learning
AI 理解论文
溯源树
样例
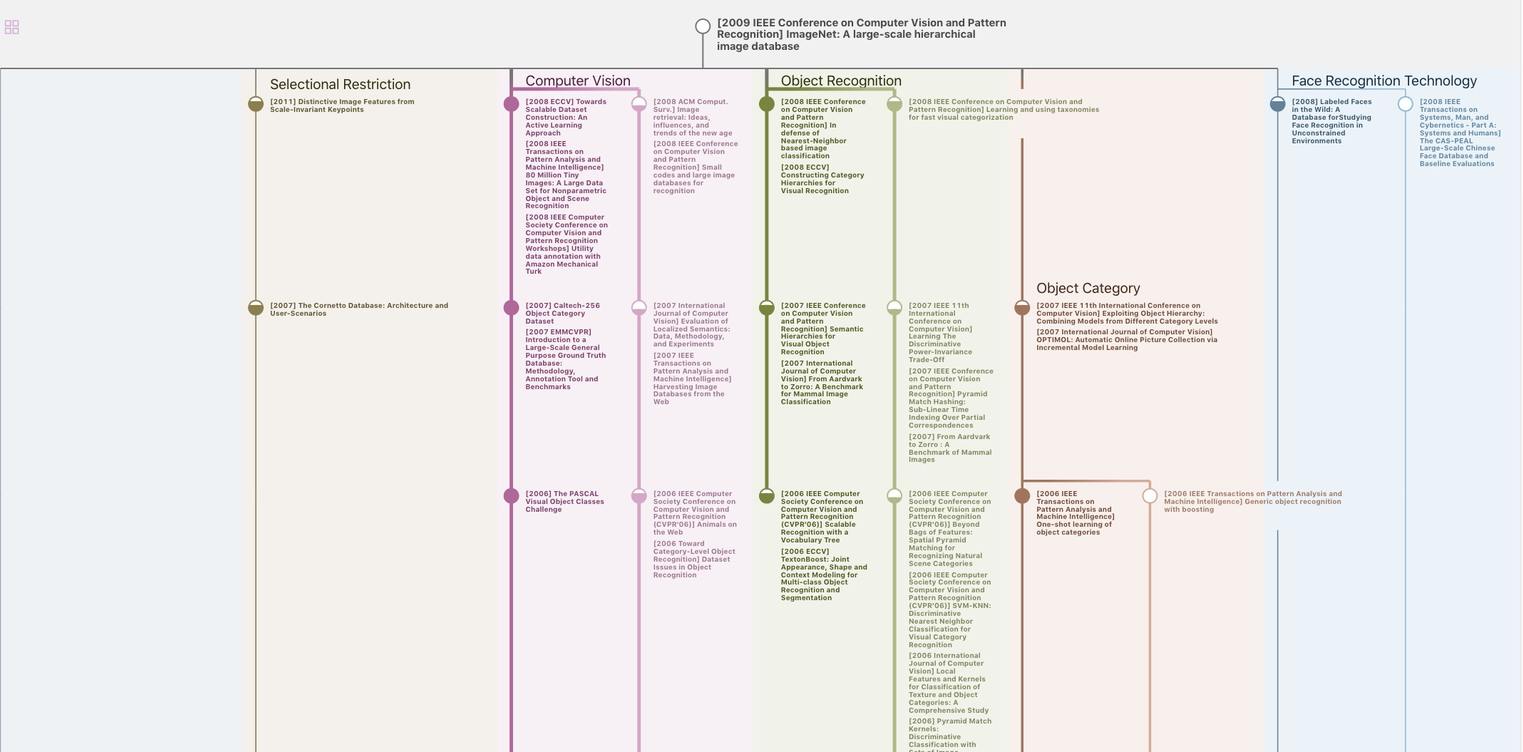
生成溯源树,研究论文发展脉络
Chat Paper
正在生成论文摘要