Automated quantitative trait locus analysis (AutoQTL)
biorxiv(2023)
摘要
Background Quantitative Trait Locus (QTL) analysis and Genome-Wide Association Studies (GWAS) have the power to identify variants that capture significant levels of phenotypic variance in complex traits. However, effort and time are required to select the best methods and optimize parameters and pre-processing steps. Although machine learning approaches have been shown to greatly assist in optimization and data processing, applying them to QTL analysis and GWAS is challenging due to the complexity of large, heterogenous datasets. Here, we describe proof-of-concept for an automated machine learning approach, AutoQTL, with the ability to automate many complex decisions related to analysis of complex traits and generate diverse solutions to describe relationships that exist in genetic data.
Results Using a dataset of 18 putative QTL from a large-scale GWAS of body mass index in the laboratory rat, Rattus norvegicus , AutoQTL captures the phenotypic variance explained under a standard additive model while also providing evidence of non-additive effects including deviations from additivity and 2-way epistatic interactions from simulated data via multiple optimal solutions. Additionally, feature importance metrics provide different insights into the inheritance models and predictive power of multiple GWAS-derived putative QTL.
Conclusions This proof-of-concept illustrates that automated machine learning techniques can be applied to genetic data and has the potential to detect both additive and non-additive effects via various optimal solutions and feature importance metrics. In the future, we aim to expand AutoQTL to accommodate omics-level datasets with intelligent feature selection strategies.
### Competing Interest Statement
The authors have declared no competing interest.
* DEAP
: Distributed Evolutionary Algorithms in Python
DS
: Difference Score
DT
: Decision Tree (regressor)
GF
: Genotype Frequency (feature selector)
GP
: Genetic Programming
GWAS
: Genome-Wide Association Study
LR
: Linear Regression (regressor)
NSGA
: Non-Dominated Sorted Genetic Algorithm II
PCA
: Principal Component Analysis
QTL
: Quantitative Trait Locus
RF
: Random Forest (regressor)
SHAP
: Shapley Additive exPlanations
SNP
: Single Nucleotide Polymorphism
SP
: Select Percentile (feature selector)
TPOT
: Tree-based Pipeline Optimization Tool
V P
: Phenotypic Variance
VT
: Variance Threshold (feature selector)
更多查看译文
AI 理解论文
溯源树
样例
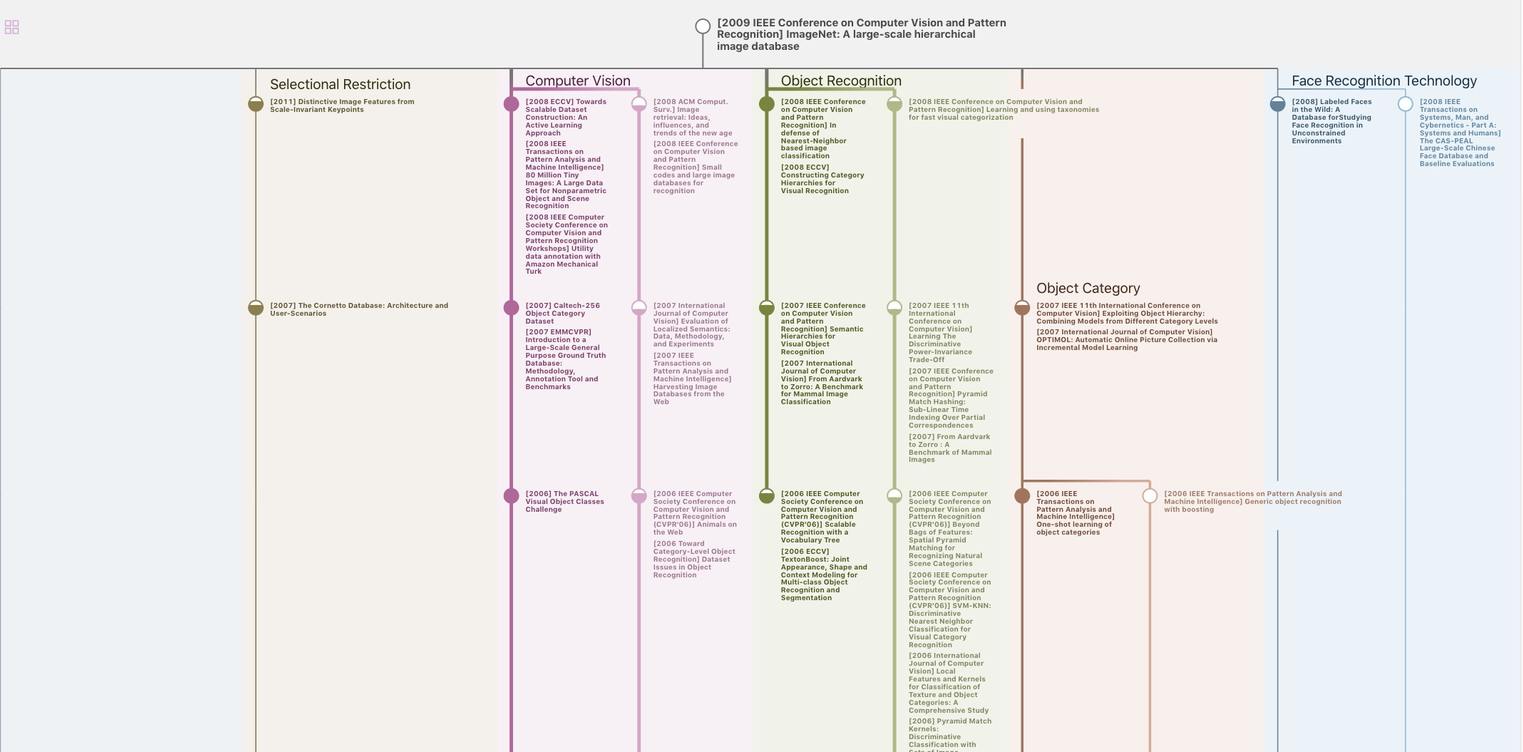
生成溯源树,研究论文发展脉络
Chat Paper
正在生成论文摘要