Language-Informed Transfer Learning for Embodied Household Activities
arXiv (Cornell University)(2023)
摘要
For service robots to become general-purpose in everyday household environments, they need not only a large library of primitive skills, but also the ability to quickly learn novel tasks specified by users. Fine-tuning neural networks on a variety of downstream tasks has been successful in many vision and language domains, but research is still limited on transfer learning between diverse long-horizon tasks. We propose that, compared to reinforcement learning for a new household activity from scratch, home robots can benefit from transferring the value and policy networks trained for similar tasks. We evaluate this idea in the BEHAVIOR simulation benchmark which includes a large number of household activities and a set of action primitives. For easy mapping between state spaces of different tasks, we provide a text-based representation and leverage language models to produce a common embedding space. The results show that the selection of similar source activities can be informed by the semantic similarity of state and goal descriptions with the target task. We further analyze the results and discuss ways to overcome the problem of catastrophic forgetting.
更多查看译文
关键词
transfer learning,activities,language-informed
AI 理解论文
溯源树
样例
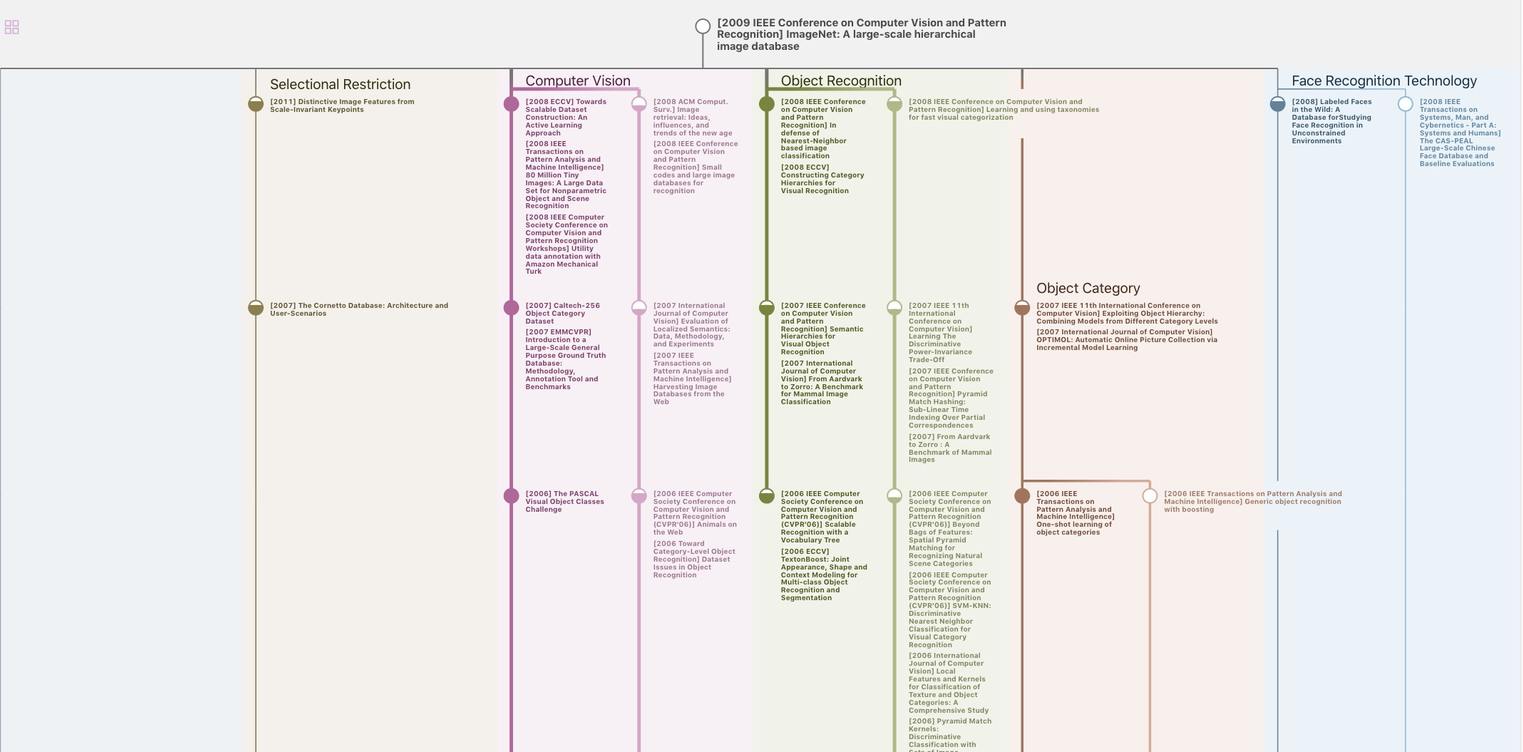
生成溯源树,研究论文发展脉络
Chat Paper
正在生成论文摘要