Determining the Optimal Neural Network Structure for the Development of Decision Support Programmes in Dental Implantation
Medicinskij alfavit(2023)
摘要
Introduction. Medicine is currently considered one of the strategic and promising areas for effective implementation of artificial intelligence. Artificial intelligence in dentistry is a method of creating a second informed opinion that is based on mathematical decision making and prediction. Neural network technologies are applied in areas such as analysis of dental radiographs, prediction of oral treatment needs in children, classification of dental plaque and treatment planning for orthognathic surgery, and assisted diagnosis of caries.The purpose of the study was to develop an optimal neural network structure to assess the risk of complications in implant treatment of partial and total tooth loss.Material and methods. For the effective selection the optimal topology of the neural network to assess the risk of complications in the implant treatment of tooth loss a series of experimental simulations of neural network architectures by trial and error was conducted. The database was a table of 1800 patient clinical cases. A total of 1626 clinical cases were used for the simulations, which were divided into data for training and testing at a percentage of 80 to 20. Modelling was done using the high-level programming language Python 3.8.8. All the simulations were performed on a 3.00 GHz Intel(R) Core(TM) i5-8500 processor with 16 GB RAM and a 64-bit Windows 10 operating system.Results. Because of the simulations, plots of recognition accuracy as well as error values for each of the developed neural network topologies were obtained. To improve the accuracy of the developed neural network topologies to assess the risk of complications during the implant treatment of maxillofacial pathology, a statistical study of the collected simulation database was conducted. For investigation the relationship between each patient parameter and the «implant engraftment acceptability» parameter, a parametric statistical method represented by the correlation coefficient was chosen. As a result of modeling by trial and error method it was found that using the neural network architecture № 7 without batch normalization layers (BatchNorm1d) allows achieving faster learning results with rather high recognition accuracy in a smaller number of epochs. Transforming the simulation base and reducing the input signal size significantly improved the recognition accuracy in comparison with the results of the first simulation of the different neural network systems for recognition of the implant engraftment success. The proposed topology of the neural network № 5 is the most optimal for the accuracy of recognition of the successful implant survival.
更多查看译文
AI 理解论文
溯源树
样例
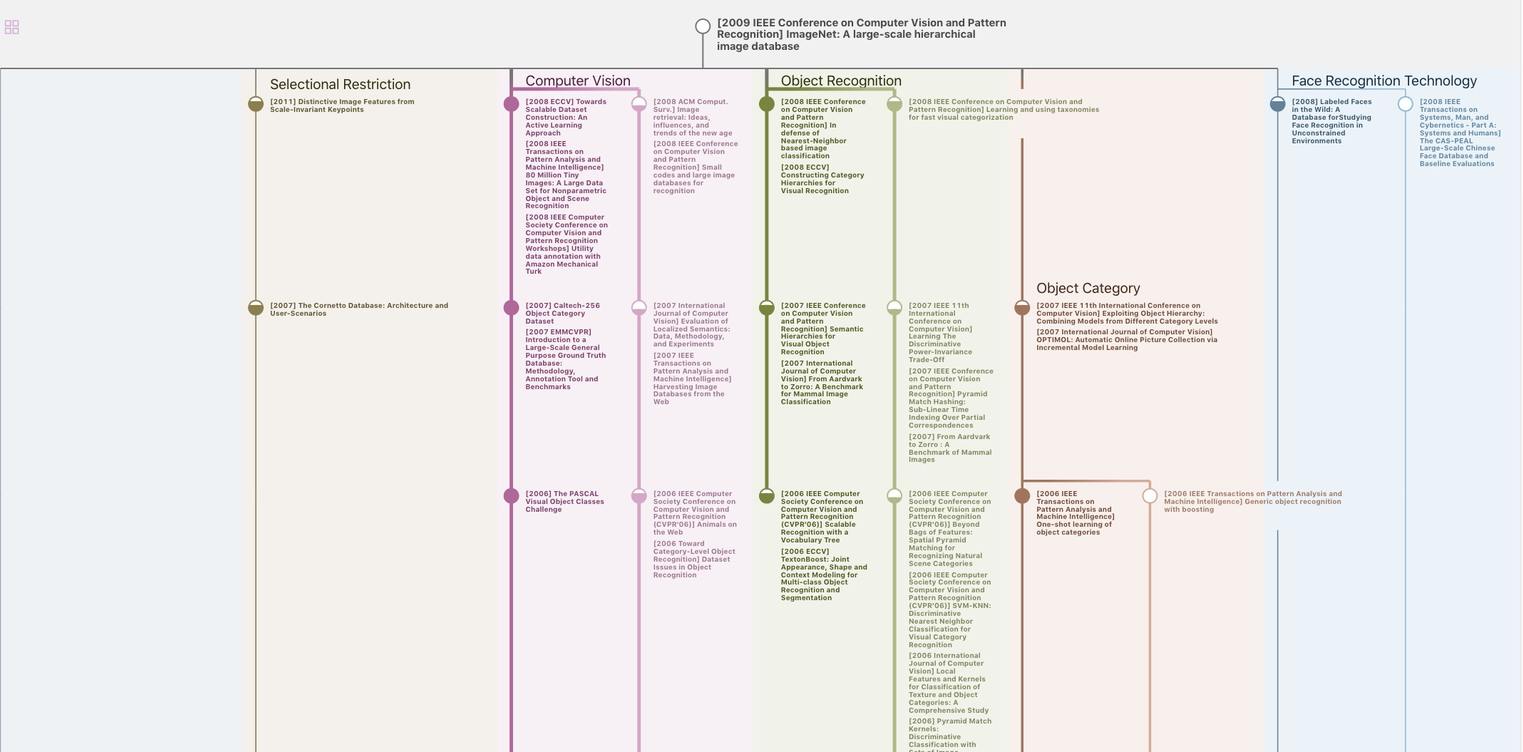
生成溯源树,研究论文发展脉络
Chat Paper
正在生成论文摘要