The Effect of Stellar Encounters on the Dark Matter Annihilation Signal from Prompt Cusps
Monthly Notices of the Royal Astronomical Society(2023)SCI 2区
Donostia Int Phys Ctr DIPC | Zhejiang Univ | Max Planck Inst Astrophys
Abstract
Prompt cusps are the densest quasi-equilibrium dark matter objects; one forms at the instant of collapse within every isolated peak of the initial cosmological density field. They have power-law density profiles, rho alpha r(-1.5) with central phase-space density set by the primordial velocity dispersion of the dark matter. At late times, they account for similar to 1 per cent of the dark matter mass but for > 90 per cent of its annihilation luminosity in all but the densest regions, where they are tidally disrupted. Here we demonstrate that individual stellar encounters rather than the mean galactic tide are the dominant disruptors of prompt cusps within galaxies. Their cumulative effect is fully (though stochastically) characterized by an impulsive shock strength B-* = 2 pi G integral rho(*)(x(t)) dt where rho(*), the total mass density in stars, is integrated over a cusp's entire post-formation trajectory. Stellar encounters and mean tides have only a small effect on the halo annihilation luminosity seen by distant observers, but this is not true for the Galactic halo because of the Sun's position. For a 100 GeV WIMP, Earth-mass prompt cusps are predicted, and stellar encounters suppress their mean annihilation luminosity by a factor of two already at 20 kpc, so that their annihilation emission is predicted to appear almost uniform over the sky. The Galactic centre gamma-ray excess is thus unaffected by cusps. If it is indeed dark matter annihilation radiation, then prompt cusps in the outer Galactic halo and beyond must account for 20-80 per cent of the observed isotropic gamma-ray background in the 1-10 GeV range.
MoreTranslated text
Key words
Direct Detection
PDF
View via Publisher
AI Read Science
AI Summary
AI Summary is the key point extracted automatically understanding the full text of the paper, including the background, methods, results, conclusions, icons and other key content, so that you can get the outline of the paper at a glance.
Example
Background
Key content
Introduction
Methods
Results
Related work
Fund
Key content
- Pretraining has recently greatly promoted the development of natural language processing (NLP)
- We show that M6 outperforms the baselines in multimodal downstream tasks, and the large M6 with 10 parameters can reach a better performance
- We propose a method called M6 that is able to process information of multiple modalities and perform both single-modal and cross-modal understanding and generation
- The model is scaled to large model with 10 billion parameters with sophisticated deployment, and the 10 -parameter M6-large is the largest pretrained model in Chinese
- Experimental results show that our proposed M6 outperforms the baseline in a number of downstream tasks concerning both single modality and multiple modalities We will continue the pretraining of extremely large models by increasing data to explore the limit of its performance
Try using models to generate summary,it takes about 60s
Must-Reading Tree
Example
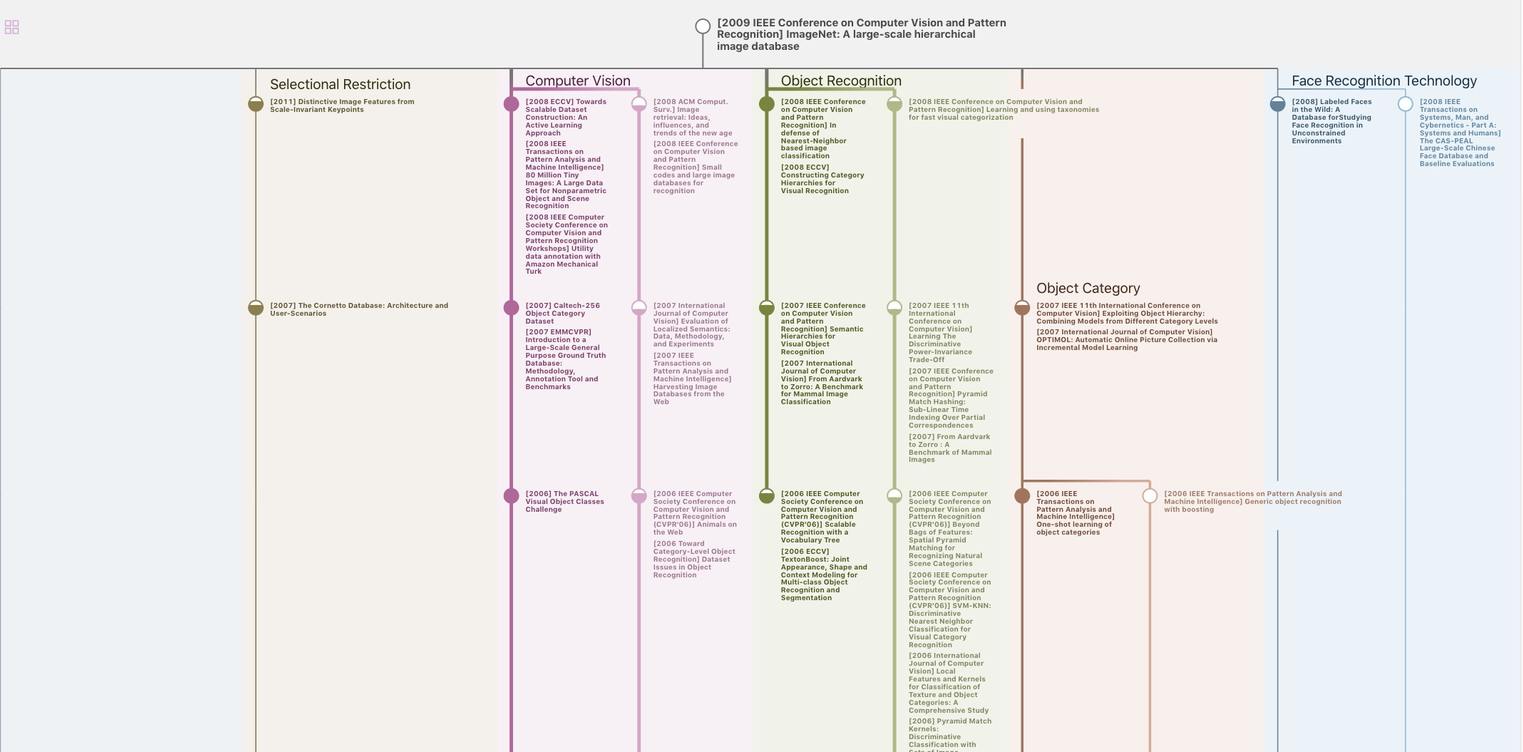
Generate MRT to find the research sequence of this paper
Related Papers
2006
被引用173 | 浏览
2015
被引用34 | 浏览
2011
被引用1411 | 浏览
2011
被引用693 | 浏览
2018
被引用60 | 浏览
2020
被引用70 | 浏览
Data Disclaimer
The page data are from open Internet sources, cooperative publishers and automatic analysis results through AI technology. We do not make any commitments and guarantees for the validity, accuracy, correctness, reliability, completeness and timeliness of the page data. If you have any questions, please contact us by email: report@aminer.cn
Chat Paper
GPU is busy, summary generation fails
Rerequest