Mean-field MARL-based Priority-Aware CSMA/CA Strategy in Large-Scale MANETs
GLOBECOM 2022 - 2022 IEEE Global Communications Conference(2022)
摘要
Mobile ad-hoc network (MANET) has attracted ex-tensive attention in many applications with nodes operating over bandwidth-constrained wireless links in a self-organized manner. Typically, to avoid potential collisions, CSMA/CA method is widely adopted in MANETs and affects the provisioning quality of service (QoS). However, due to the potentially severe collisions in a large-scale MANET, current CSMA/CA methods fail to support QoS effectively, especially for scenarios with different priority services. Here, we propose a priority-aware CSMA/CA strategy to use the higher-priority packets to collect the queueing information from adjacent nodes in a piggyback manner and model the channel access process by a partial observation Markov decision process (POMDP). Furthermore mean-field multi-agent reinforcement learning (MARL) is adopted to allow each individual node in the MANET to dynamically select the appropriate contention window (CW) and gradually compute the global optimal policy. Finally, extensive simulation results verify significantly lower delay and packet loss rate for the higher- priority services and comparable provisioning quality for lower- priority services, which reflects the superiority over baselines.
更多查看译文
关键词
Mean-field game,MARL,MANET,Priority,CSMA/CA
AI 理解论文
溯源树
样例
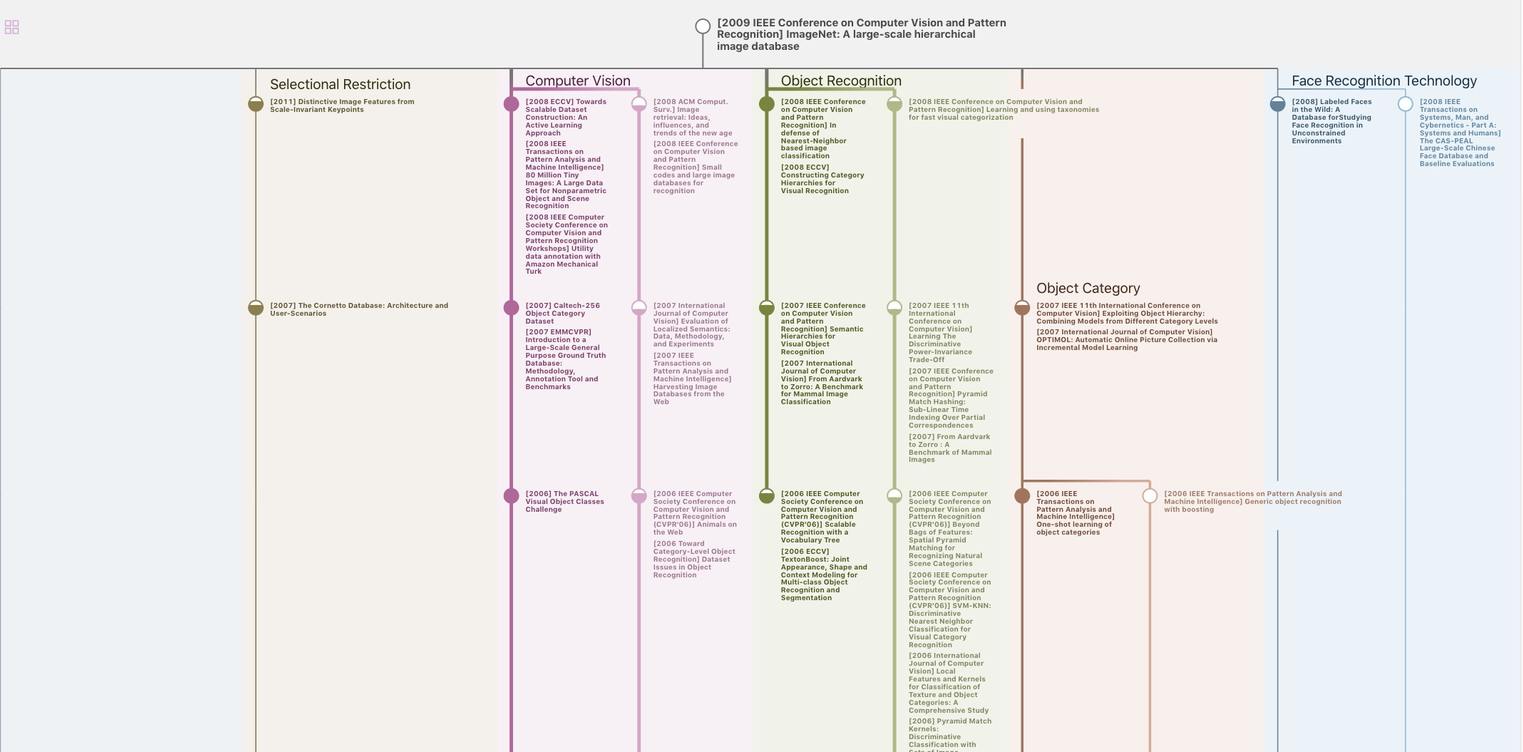
生成溯源树,研究论文发展脉络
Chat Paper
正在生成论文摘要