Computational Strategies and Estimation Performance with Bayesian Semiparametric Item Response Theory Models
JOURNAL OF EDUCATIONAL AND BEHAVIORAL STATISTICS(2023)
Abstract
Item response theory (IRT) models typically rely on a normality assumption for subject-specific latent traits, which is often unrealistic in practice. Semiparametric extensions based on Dirichlet process mixtures (DPMs) offer a more flexible representation of the unknown distribution of the latent trait. However, the use of such models in the IRT literature has been extremely limited, in good part because of the lack of comprehensive studies and accessible software tools. This article provides guidance for practitioners on semiparametric IRT models and their implementation. In particular, we rely on NIMBLE, a flexible software system for hierarchical models that enables the use of DPMs. We highlight efficient sampling strategies for model estimation and compare inferential results under parametric and semiparametric models.
MoreTranslated text
Key words
binary IRT models,Dirichlet process mixture,MCMC strategies,NIMBLE
AI Read Science
Must-Reading Tree
Example
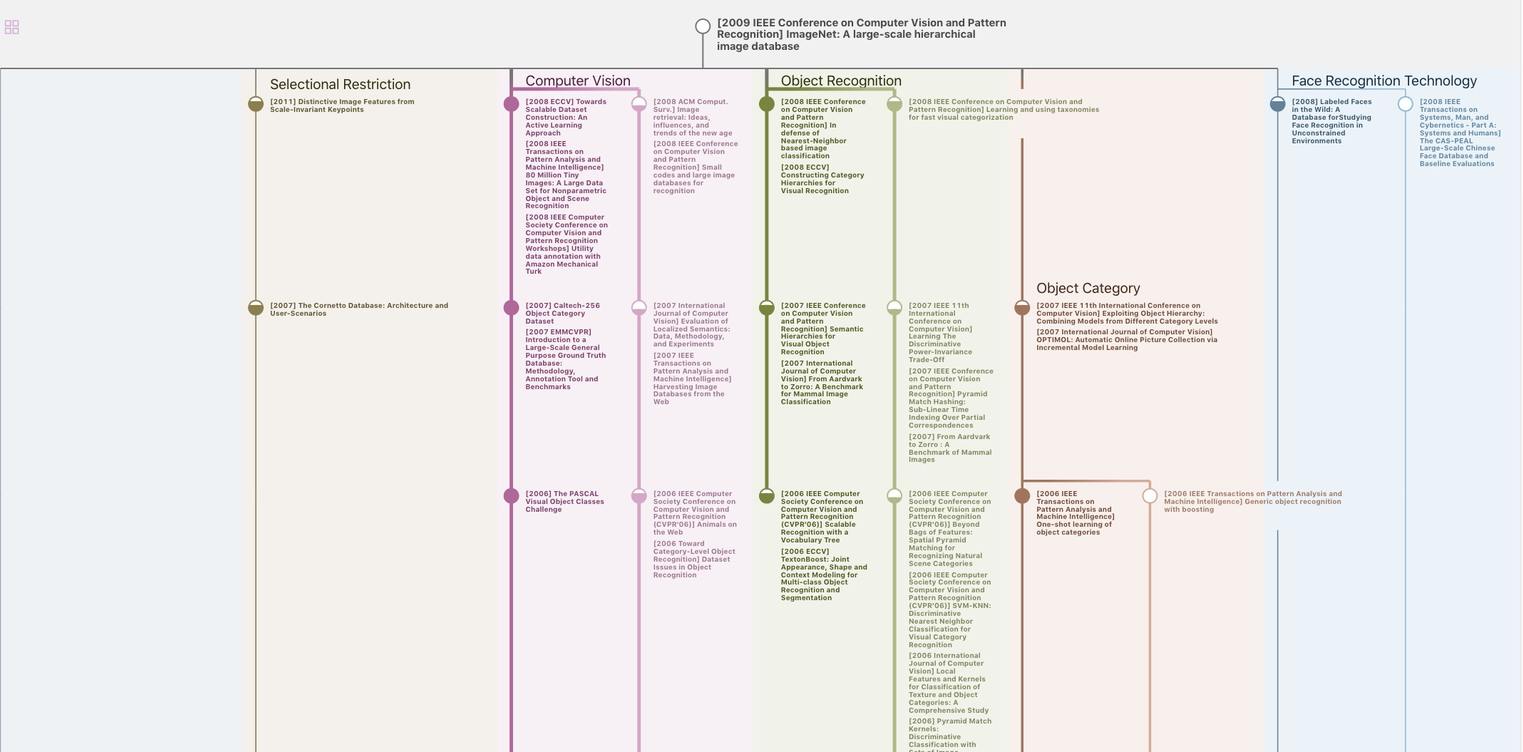
Generate MRT to find the research sequence of this paper
Chat Paper
Summary is being generated by the instructions you defined